Elliptic Curve ‘Murmurations’ Found With AI Take Flight
Introduction
Elliptic curves are among the more beguiling objects in modern mathematics. They don’t seem complicated, but they form an expressway between the math that many people learn in high school and research mathematics at its most abstruse. They were central to Andrew Wiles’ celebrated proof of Fermat’s Last Theorem in the 1990s. They are key tools in modern cryptography. And in 2000, the Clay Mathematics Institute named a conjecture about the statistics (opens a new tab) of elliptic curves one of seven “Millennium Prize Problems,” each of which carries a $1 million prize for its solution. That conjecture, first ventured by Bryan Birch (opens a new tab) and Peter Swinnerton-Dyer (opens a new tab) in the 1960s, still hasn’t been proved.
Understanding elliptic curves is a high-stakes endeavor that has been central to math. So in 2022, when a transatlantic collaboration used statistical techniques and artificial intelligence to discover completely unexpected patterns in elliptic curves, it was a welcome, if unexpected, contribution. “It was just a matter of time before machine learning landed on our front doorstep with something interesting,” said Peter Sarnak (opens a new tab), a mathematician at the Institute for Advanced Study and Princeton University. Initially, nobody could explain why the newly discovered patterns exist. Since then, in a series of recent papers, mathematicians have begun to unlock the reasons behind the patterns, dubbed “murmurations” for their resemblance to the fluid shapes of flocking starlings, and have started to prove that they must occur not only in the particular examples examined in 2022, but in elliptic curves more generally.
The Importance of Being Elliptic
To understand what those patterns are, we have to lay a little groundwork about what elliptic curves are and how mathematicians categorize them.
An elliptic curve relates the square of one variable, commonly written as y, to the third power of another, commonly written as x: y2 = x3 + Ax + B, for some pair of numbers A and B, as long as A and B meet a few straightforward conditions. This equation defines a curve that can be graphed on the plane, as shown below. (Despite the similarity in the names, an ellipse is not an elliptic curve.)
Merrill Sherman/Quanta Magazine
Though plain-looking, elliptic curves turn out to be incredibly powerful tools for number theorists — mathematicians who look for patterns in the integers. Instead of letting the variables x and y range over all numbers, mathematicians like to restrict them to different number systems, which they call defining a curve “over” a given number system. Elliptic curves restricted to the rational numbers — numbers that can be written as fractions — are particularly useful. “Elliptic curves over the real or complex numbers are quite boring,” Sarnak said. “It’s only the rational numbers that are deep.”
Here’s one way that’s true. If you draw a straight line between two rational points on an elliptic curve, the place where that line intersects the curve again will also be rational. You can use that fact to define “addition” in an elliptic curve, as shown below.
Draw a line between P and Q. That line will intersect the curve at a third point, R. (Mathematicians have a special trick for dealing with the case where the line doesn’t intersect the curve by adding a “point at infinity.”) The reflection of R across the x-axis is your sum P + Q. Together with this addition operation, all the solutions to the curve form a mathematical object called a group.
Mathematicians use this to define the “rank” of a curve. The rank of a curve relates to the number of rational solutions it has. Rank 0 curves have a finite number of solutions. Curves with higher rank have infinite numbers of solutions whose relationship to one another using the addition operation is described by the rank.
Ranks are not well understood; mathematicians don’t always have a way of computing them and don’t know how big they can get. (The largest exact rank known for a specific curve is 20.) Similar-looking curves can have completely different ranks.
Elliptic curves also have a lot to do with prime numbers, which are only divisible by 1 and themselves. In particular, mathematicians look at curves over finite fields — systems of cyclical arithmetic that are defined for each prime number. A finite field is like a clock with the number of hours equal to the prime: If you keep counting upward, the numbers start over again. In the finite field for 7, for example, 5 plus 2 equals zero, and 5 plus 3 equals 1.
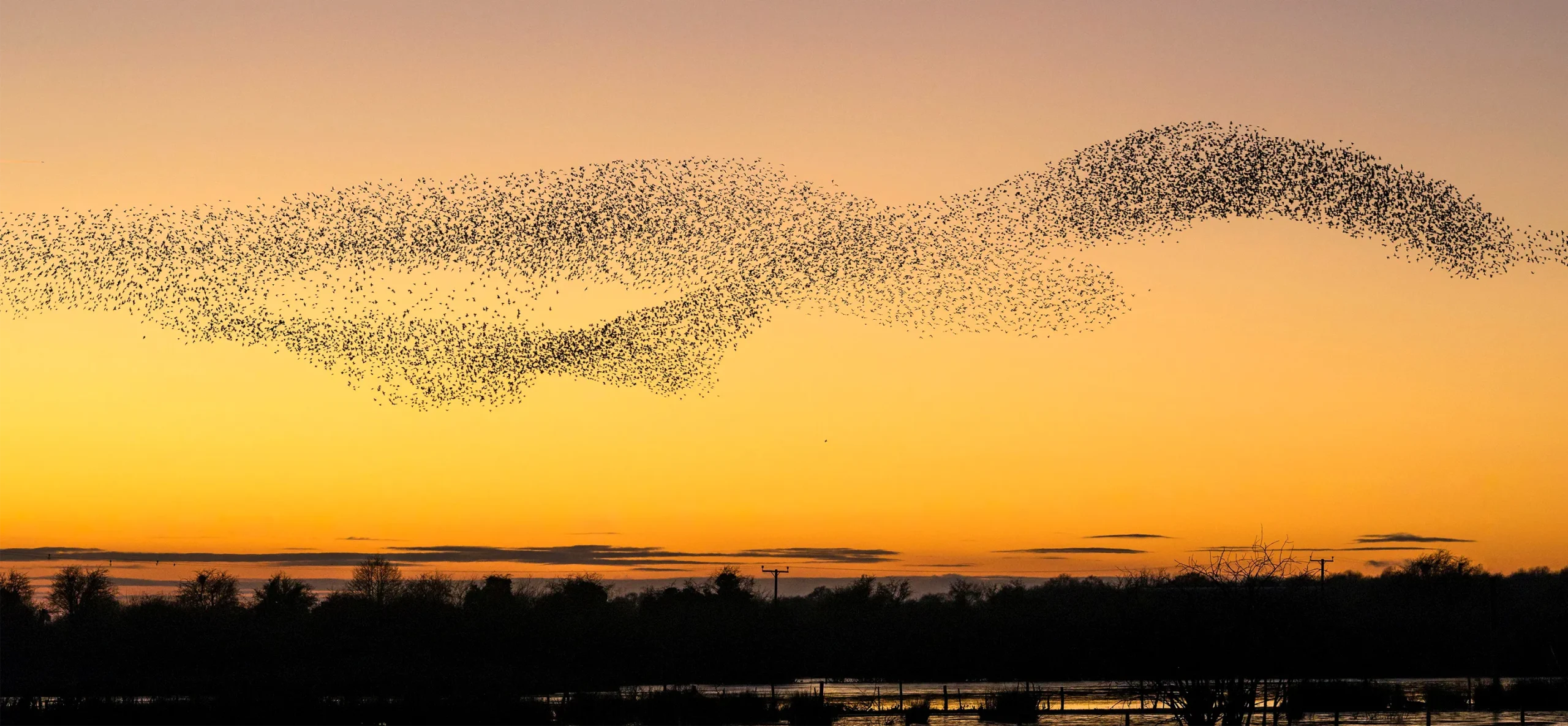
Patterns formed by thousands of elliptic curves bear a striking similarity to murmurations of starlings.
Alex Ramsay/Alamy Stock Photo
An elliptic curve has an associated sequence of numbers, called ap, which relates to the number of solutions there are to the curve in the finite field defined by the prime p. A smaller ap means more solutions; a bigger ap means fewer solutions. Though the rank is hard to calculate, the sequence ap is a lot easier.
On the basis of numerous calculations done on one of the very first computers, Birch and Swinnerton-Dyer conjectured a relationship between an elliptic curve’s rank and the sequence ap. Anyone who can prove they were right stands to win a million dollars and mathematical immortality.
A Surprise Pattern Emerges
After the start of the pandemic, Yang-Hui He (opens a new tab), a researcher at the London Institute for Mathematical Sciences, decided to take on some new challenges. He had been a physics major in college, and had gotten his doctorate from the Massachusetts Institute of Technology in mathematical physics. But he was increasingly interested in number theory, and given the increasing capabilities of artificial intelligence, he thought he’d try his hand at using AI as a tool for finding unexpected patterns in numbers. (He had already been using machine learning (opens a new tab) to classify Calabi-Yau manifolds, mathematical structures that are widely used in string theory.)
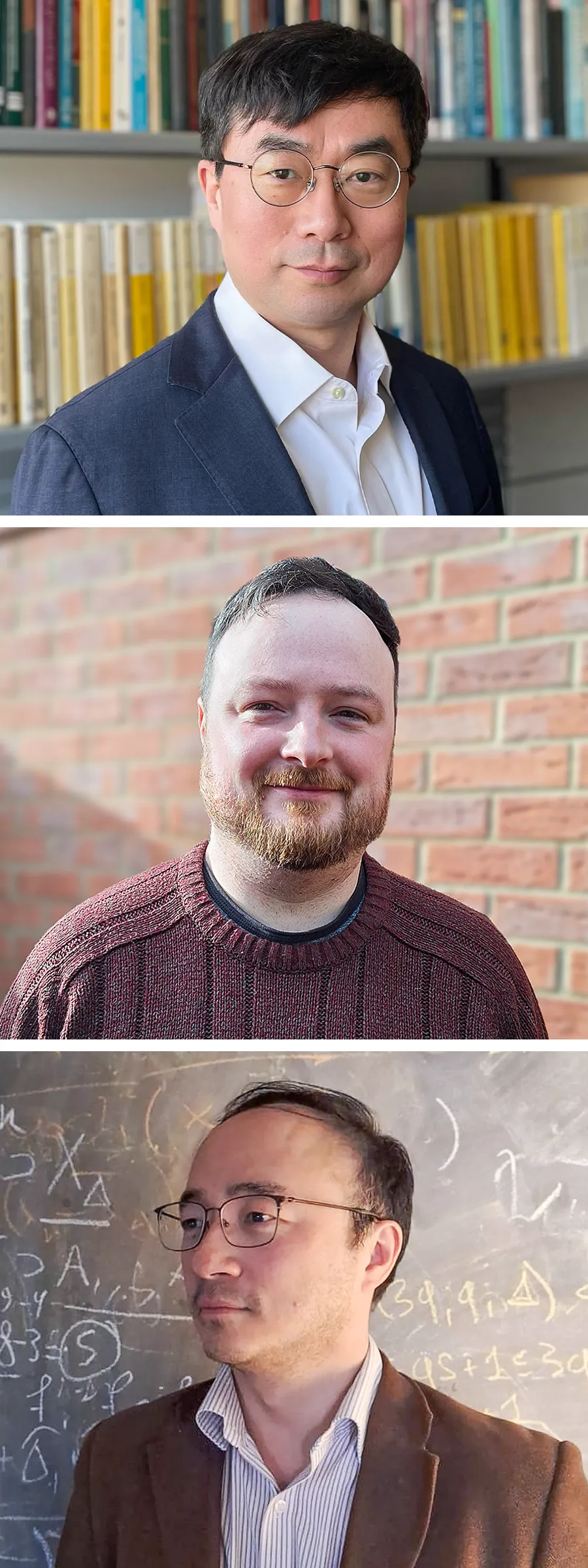
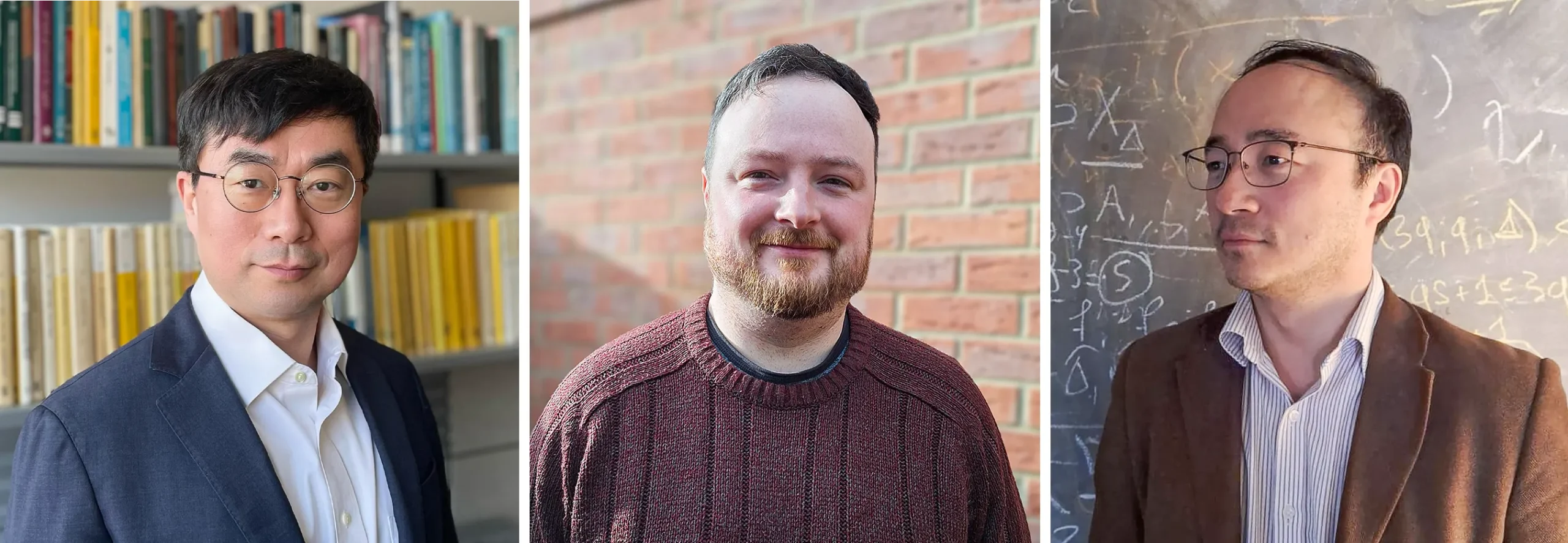
When Kyu-Hwan Lee (left) and Thomas Oliver (center) began working with Yang-Hui He (right) to use artificial intelligence to find mathematical patterns, they expected it to be a lark, rather than an effort that would lead to new discoveries.
From left: Grace Lee; Sophie Oliver; courtesy of Yang-Hui He
In August 2020, as the pandemic deepened, the University of Nottingham hosted him for an online talk (opens a new tab). He was pessimistic about his progress, and about the very possibility of using machine learning to uncover new math. “His narrative was that number theory was hard because you couldn’t machine-learn things in number theory,” said Thomas Oliver (opens a new tab), a mathematician at the University of Westminster who was in the audience. As He remembers, “I couldn’t find anything because I wasn’t an expert. I was not even using the right things to look at this.”
Oliver and Kyu-Hwan Lee (opens a new tab), a mathematician at the University of Connecticut, began working with He. “We decided to do this just to learn what machine learning was, rather than to seriously study mathematics,” Oliver said. “But we quickly found that you could machine-learn a lot of things.”
Oliver and Lee suggested that He apply his techniques to examine L-functions, infinite series closely related to elliptic curves through the sequence ap. They could use an online database of elliptic curves and their related L-functions called the LMFDB (opens a new tab) to train their machine learning classifiers. At the time the database had a little over 3 million elliptic curves over the rationals. By October 2020, they had a paper (opens a new tab) that used information gleaned from L-functions to predict a particular property of elliptic curves. In November they shared another paper (opens a new tab) that used machine learning to classify other objects in number theory. By December, they were able to predict the ranks of elliptic curves (opens a new tab) with high accuracy.
But they weren’t sure why their machine learning algorithms were working so well. Lee asked his undergraduate student Alexey Pozdnyakov to see if he could figure out what was going on. As it happens, the LMFDB sorts elliptic curves according to a quantity called the conductor, which summarizes information about primes for which a curve fails to behave well. So Pozdnyakov tried looking at large numbers of curves with similar conductors simultaneously — say, all the curves with conductors between 7,500 and 10,000.
This amounted to about 10,000 curves in total. About half of these had rank 0, and half rank 1. (Higher ranks are exceedingly rare.) He then averaged the values of ap for all the rank 0 curves, separately averaged ap for all the rank 1 curves, and plotted the results. The two sets of dots formed two distinct, easily discernible waves. That was why the machine learning classifiers had been able to correctly ascertain the ranks of particular curves.
“At first I just felt happy that I’d completed the assignment,” Pozdnyakov said. “But Kyu-Hwan immediately recognized that this pattern was surprising, and that’s when it became really exciting.”
Lee and Oliver were enthralled. “Alexey showed us the picture, and I said it looks like that thing that birds do,” Oliver said. “And then Kyu-Hwan looked it up and said it’s called a murmuration, and then Yang said we should call the paper ‘Murmurations of Elliptic Curves (opens a new tab).’”
They uploaded their paper in April 2022 and forwarded it to a handful of other mathematicians, nervously expecting to be told that their so-called “discovery” was well known. Oliver said that the relationship was so visible that it should have been noticed long ago.
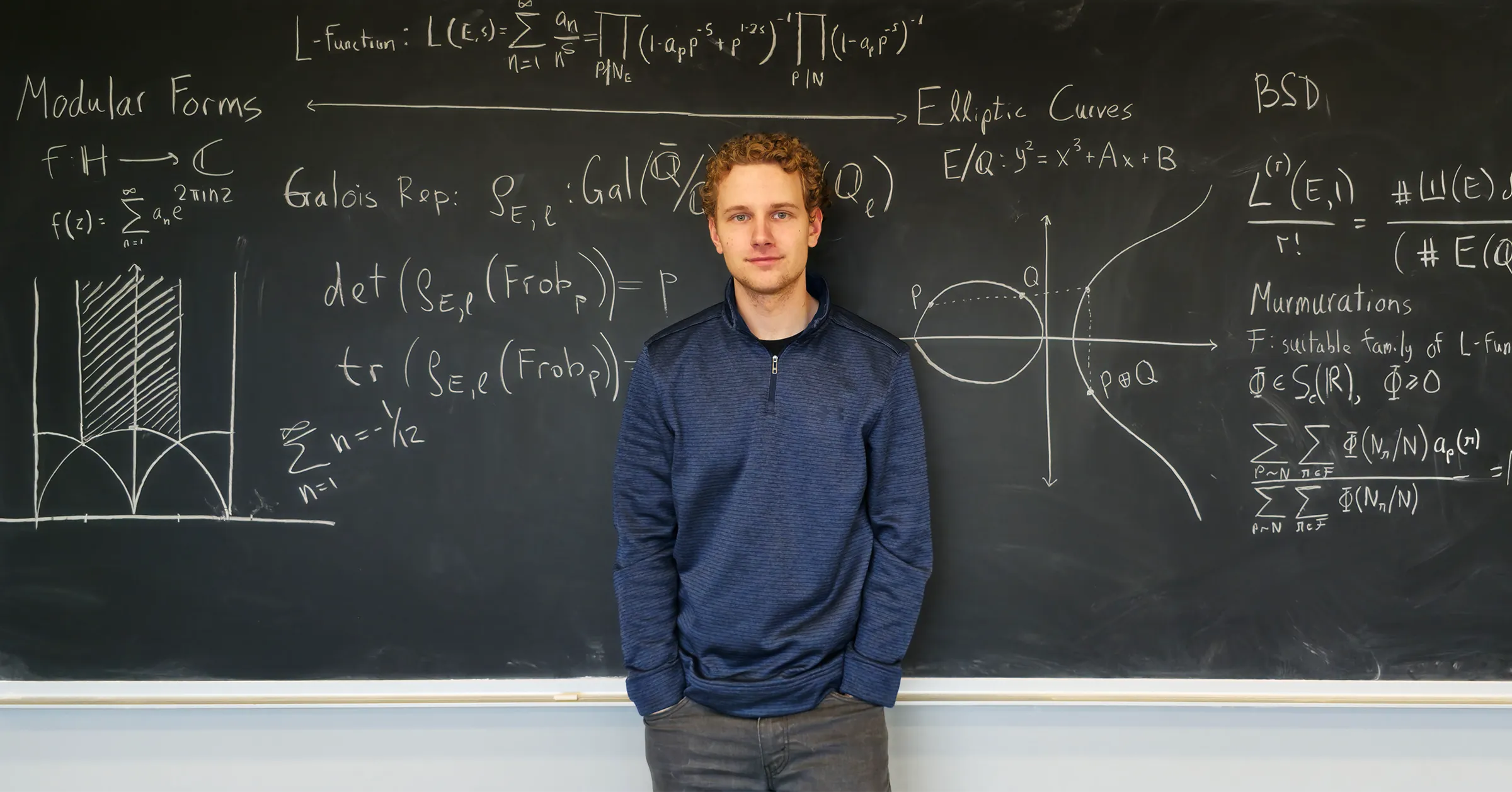
Alexey Pozdnyakov, an undergraduate at the University of Connecticut, was the first person to observe the patterns now known as murmurations.
Vladimir Pozdnyakov
Almost immediately, the preprint garnered interest, particularly from Andrew Sutherland (opens a new tab), a research scientist at MIT who is one of the managing editors of the LMFDB. Sutherland realized that 3 million elliptic curves weren’t enough for his purposes. He wanted to look at much larger conductor ranges to see how robust the murmurations were. He pulled data from another immense repository of about 150 million elliptic curves. Still unsatisfied, he then pulled in data from a different repository with 300 million curves.
“But even those weren’t enough, so I actually computed a new data set of over a billion elliptic curves, and that’s what I used to compute the really high-res pictures,” Sutherland said. The murmurations showed up whether he averaged over 15,000 elliptic curves at a time or a million at a time. The shape stayed the same even as he looked at the curves over larger and larger prime numbers, a phenomenon called scale invariance. Sutherland also realized that murmurations are not unique to elliptic curves, but also appear in more general L-functions. He wrote a letter summarizing his findings (opens a new tab) and sent it to Sarnak and Michael Rubinstein (opens a new tab) at the University of Waterloo.
“If there is a known explanation for it I expect you will know it,” Sutherland wrote.
They didn’t.
Explaining the Pattern
Lee, He and Oliver organized a workshop on murmurations in August 2023 at Brown University’s Institute for Computational and Experimental Research in Mathematics (ICERM). Sarnak and Rubinstein came, as did Sarnak’s student Nina Zubrilina (opens a new tab).
Zubrilina presented her research into murmuration patterns in modular forms, special complex functions which, like elliptic curves, have associated L-functions. In modular forms with large conductors, the murmurations converge into a sharply defined curve, rather than forming a discernible but dispersed pattern. In a paper (opens a new tab) posted on October 11, 2023, Zubrilina proved that this type of murmuration follows an explicit formula she discovered.
“Nina’s big achievement is that she’s given a formula for this; I call it the Zubrilina murmuration density formula,” Sarnak said. “Using very sophisticated math, she has proven an exact formula which fits the data perfectly.”
Her formula is complicated, but Sarnak hails it as an important new kind of function, comparable to the Airy functions that define solutions to differential equations used in a variety of contexts in physics, ranging from optics to quantum mechanics.
Though Zubrilina’s formula was the first, others have followed. “Every week now, there’s a new paper out,” Sarnak said, “mainly using Zubrilina’s tools, explaining other aspects of murmurations.”
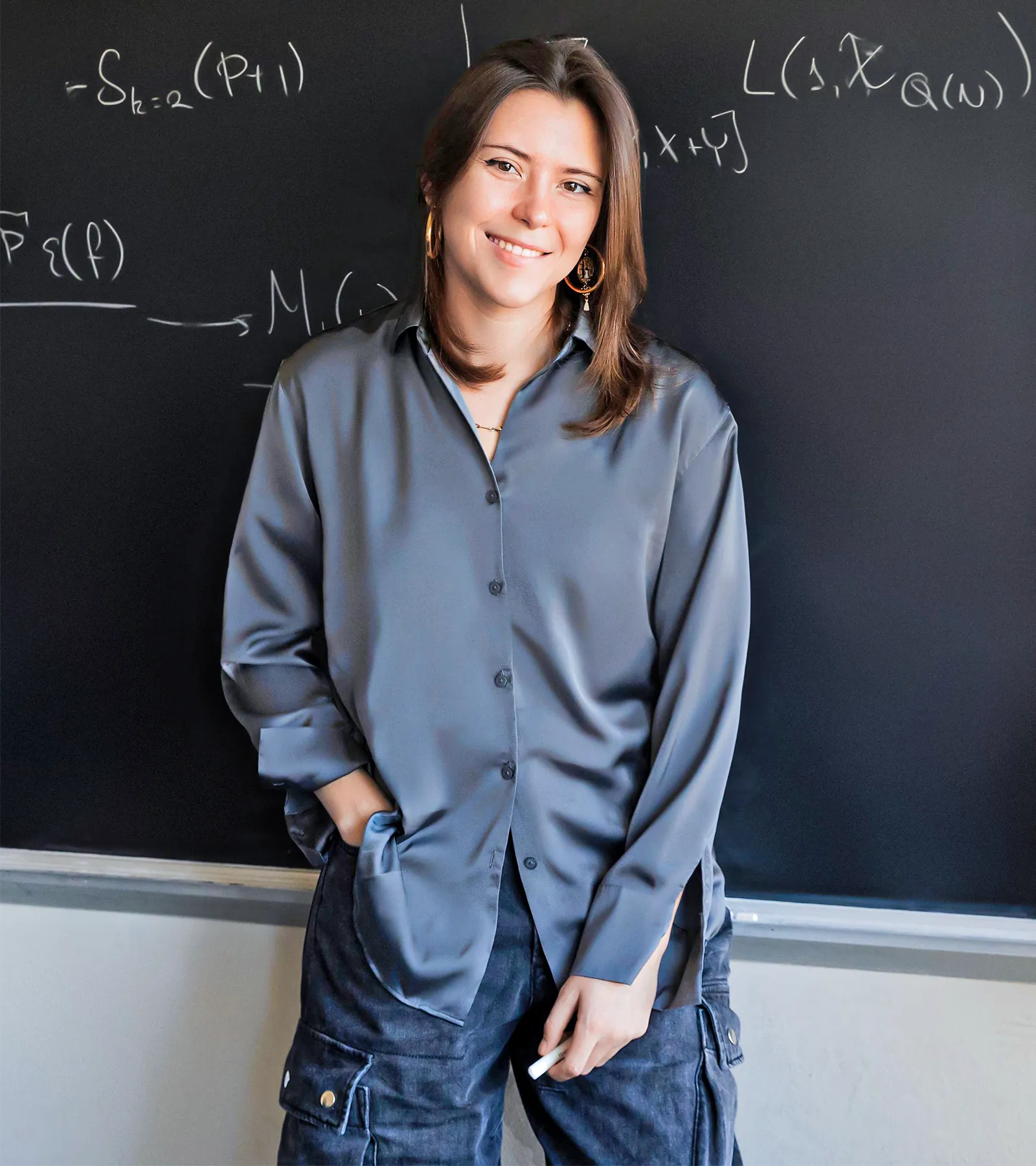
Nina Zubrilina, who is close to finishing her doctorate at Princeton, proved a formula that explains the murmuration patterns.
Julie Dassaro Photography
Jonathan Bober (opens a new tab), Andrew Booker (opens a new tab) and Min Lee (opens a new tab) of the University of Bristol, together with David Lowry-Duda (opens a new tab) of ICERM, proved the existence of a different type of murmuration in modular forms in another October paper (opens a new tab). And Kyu-Hwan Lee, Oliver and Pozdnyakov proved the existence (opens a new tab) of murmurations in objects called Dirichlet characters that are closely related to L-functions.
Sutherland was impressed by the significant dose of luck that had led to the discovery of murmurations. If the elliptic curve data hadn’t been ordered by conductor, the murmurations would have disappeared. “They were fortunate to be taking data from the LMFDB, which came pre-sorted according to the conductor,” he said. “It’s what relates an elliptic curve to the corresponding modular form, but that’s not at all obvious. … Two curves whose equations look very similar can have very different conductors.” For example, Sutherland noted that y2 = x3 – 11x + 6 has conductor 17, but flipping the minus sign to a plus sign, y2 = x3 + 11x + 6 has conductor 100,736.
Even then, the murmurations were only found because of Pozdnyakov’s inexperience. “I don’t think we would have found it without him,” Oliver said, “because the experts traditionally normalize ap to have absolute value 1. But he didn’t normalize them … so the oscillations were very big and visible.”
The statistical patterns that AI algorithms use to sort elliptic curves by rank exist in a parameter space with hundreds of dimensions — too many for people to sort through in their minds, let alone visualize, Oliver noted. But though machine learning found the hidden oscillations, “only later did we understand them to be the murmurations.”
Editor’s Note: Andrew Sutherland, Kyu-Hwan Lee and the L-functions and modular forms database (LMFDB) have all received funding from the Simons Foundation, which also funds this editorially independent publication. Simons Foundation funding decisions have no influence on our coverage. More information is available here.