The Game Theory of Life
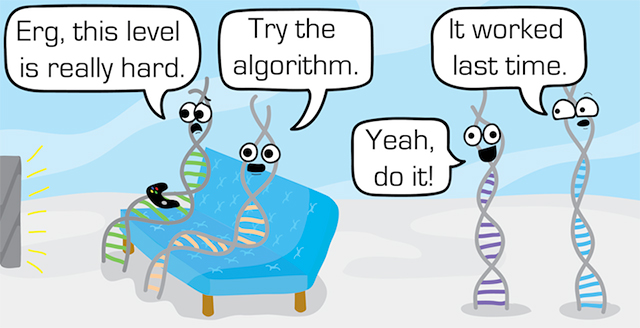
In what appears to be the first study of its kind, computer scientists report that an algorithm discovered more than 50 years ago in game theory and now widely used in machine learning is mathematically identical to the equations used to describe the distribution of genes within a population of organisms. Researchers may be able to use the algorithm, which is surprisingly simple and powerful, to better understand how natural selection works and how populations maintain their genetic diversity.
By viewing evolution as a repeated game, in which individual players, in this case genes, try to find a strategy that creates the fittest population, researchers found that evolution values both diversity and fitness.
Some biologists say that the findings are too new and theoretical to be of use; researchers don’t yet know how to test the ideas in living organisms. Others say the surprising connection, published Monday in the advance online version of the Proceedings of the National Academy of Sciences, may help scientists understand a puzzling feature of natural selection: The fittest organisms don’t always wipe out their weaker competition. Indeed, as evidenced by the menagerie of life on Earth, genetic diversity reigns.
“It’s a very different way to look at selection,” said Stephen Stearns, an evolutionary biologist at Yale University who was not involved in the study. “I always find radically different ways of looking at a problem interesting.”
The algorithm, which has been used to solve problems in linear programming, zero-sum games and a dozen other sophisticated computer science problems, is used to determine how an agent should weigh possible strategies when making a series of decisions. For example, imagine that you have 10 financial experts giving you advice on how to invest your savings. Each day you have to choose to follow one of them. At the start of the investment period, you know nothing about how well each expert performs. But every day, the multiplicative weights update algorithm, as it is called, instructs you to boost the probability of choosing the experts who have given the best advice and decrease it for those who have performed poorly.
“If you do this day after day, at the end of the year, you will do almost as well as if you had followed the best expert from the beginning,” said Christos Papadimitriou, a computer scientist at the University of California, Berkeley. “It’s as if you were omniscient in the beginning, singling out the best expert and following their advice day after day.”
Papadimitriou and his collaborators came across the connection between game theory and evolution when they were searching for a mathematical explanation of sex, which triggers new genetic diversity by mixing up the chromosomes from each parent. They were working with equations commonly used in population genetics, first developed nearly a century ago, that describe how the frequencies of certain genetic variations change with each generation. For example, plants that flourish in the current climate might dwindle as global warming alters conditions.
When they showed the equations to Umesh Vazirani, a computer scientist at Berkeley, he noticed parallels to a repeated coordination game — a scenario in game theory in which success depends on the players choosing mutually beneficial options. As an example, consider a situation in which two prisoners are tempted to turn on each other. If one talks, both lose; if neither talks, both win. Neither prisoner knows what the other will do. (This scenario is different than the well-known prisoner’s dilemma.)
Viewing the algorithm through the lens of evolution, genes are the players, and each gene has a number of different strategies in the form of genetic variations, or alleles. One variant of a gene might make a plant tolerate warmer temperatures or drier soil, for instance. The game is played over and over again; at the end of each round, the gene, or player, evaluates how well each of its alleles performed in the current genetic environment and then boosts the weight of the good performers and downsizes the weight of poor performers.
The researchers said the findings will provide a new way to examine the role of sex in evolution. For example, Papadimitriou said he believes that part of its role is to carry out the multiplicative weights update algorithm, though he hasn’t yet proven this mathematically.
Traditional applications of game theory to evolution examine how evolutionary processes shape an individual’s behavior. They have also been used to study the evolution of altruism and other properties. “But here, we’re talking about something completely different,” said Adi Livnat, a biologist at Virginia Polytechnic Institute in Blacksburg, Va., who collaborated on the study. The new study focuses on genes rather than individual organisms, and on the genetic makeup of the population instead of behavior.
The approach could illuminate a long-standing mystery in population biology. Just as in the financial world, where it’s best to keep a diversified portfolio, Vazirani and his collaborators found that the algorithm values both fitness and diversity. You might be tempted to place all your money on a soaring stock. But if circumstances change and that stock starts to tank, you’re better off having invested in a more balanced selection. Similarly, an organism’s genes may be perfectly tailored to a particular set of environmental conditions, but if those conditions change, a genetically diverse population is more likely to survive. “Evolution is, of course, interested in performance,” Papadimitriou said. “But it’s also interested in hedging its bets, keeping around a lot of genetic diversity because who knows what will come next.”
Evolutionary biologists know that in practice, a genetically diverse population is often more resilient than a homogeneous one because it is better able to respond to changing environments. But they have struggled to explain how such diversity is maintained. In the short term, one would expect diversity to drop as the fittest members of a population spread, knocking out the weaker, genetically dissimilar members. How do long-term needs surmount the short-term pressures?
The findings provide a “speculative suggestion” for how this might happen, though the authors don’t propose a specific mechanism, said Nick Barton, a biologist at the Institute of Science and Technology in Austria who was not involved in the study. “I don’t think it gives us the algorithm that can achieve the diversity we see on Earth in 3.5 billion years, when life first began,” he said.
Evolution and Entropy
One of the surprising discoveries of Papadimitriou’s study is that natural selection values not just fitness, but also genetic diversity, which in more technical terms is referred to as entropy. This view that evolution optimizes not just mean fitness but mean fitness and entropy is not well known, “but I think it’s a deep observation,” Adami said.
The Berkeley team isn’t the first to highlight the role entropy might play in evolution. But until now, the subject has mainly been of interest to mathematicians rather than biologists. “Applications of entropy in evolution have had a bad name, because they were very ill-defined,” Barton said. “More recently, there have been some interesting, and much sounder, ideas, which make a link between fields that are addressing a similar issue: Statistical physics and evolutionary biology both try to understand the overall properties of a complicated system, independent of the microscopic details.” These more recent results are mathematically sound, but they still don’t connect well with existing biological understanding, he said. “So it’s not clear to biologists how [the results] might help explain their open questions.”
Stearns and others in the field say it’s too soon to assess how the findings will affect our understanding of evolution. Even though the connection between different fields is interesting, “it does not actually help us understand biological evolution,” said Chris Adami, a physicist and computational biologist at Michigan State University, who was not involved in the study. “Unless such a relationship allows you to say something new either in computer science or biology, it’s just an observation.”
Evolutionary biologists are often skeptical of mathematical insights from outsiders. Although mathematicians and computer scientists regularly publish in the field, biologists disagree over how much their contributions have done to shape it. “I think it will take some time to figure out how the paper plays out,” Stearns said. “If this doesn’t cause any new data to be gathered, then it won’t be very important.” Even if the findings don’t prove relevant in the short-term, they might prove important over the long –term. Sometimes it can take decades before the right technology or approach arises to test a new theory, Stearns said.
The equations in the study are based on certain assumptions that may limit their applicability to the real world. For example, the equations don’t account for mutations, which would introduce new alleles, or strategies, into the game. (Adding this factor makes the mathematics much more complex.) Some say this simplification is a serious drawback, while others maintain that it is not so important in the short term, when existing variations have the strongest impact. “What happens when you move away from the assumptions?” said Lee Altenberg, a senior fellow at the Konrad Lorenz Institute in Austria. “They have pinned a single point on the map. But to know whether that means anything, you have to start departing from that point.”
One outcome of the analysis is likely to puzzle biologists. According to the standard view of evolution, the further a generation lies in the past, the less impact it has on the present — your ancestors from 1,000 years ago probably had less effect on your fitness than your grandparents. But if the Berkeley team’s insights hold up, “it shows us that every past generation contributes equally to what happens in the next generation,” Stearns said. “That’s an intriguing and wildly implausible claim from the standpoint of regular evolution.” Papadimitriou said his team was also perplexed by that outcome. “It is something that hopefully will make researchers rethink, revisit and interpret,” he said.
“You can’t really test these theorems in relation to real life,” Barton said. “They are tools for getting intuition about how to understand evolution.”
This article was reprinted on TheGuardian.com.