How Can Math Help Beat Cancer?
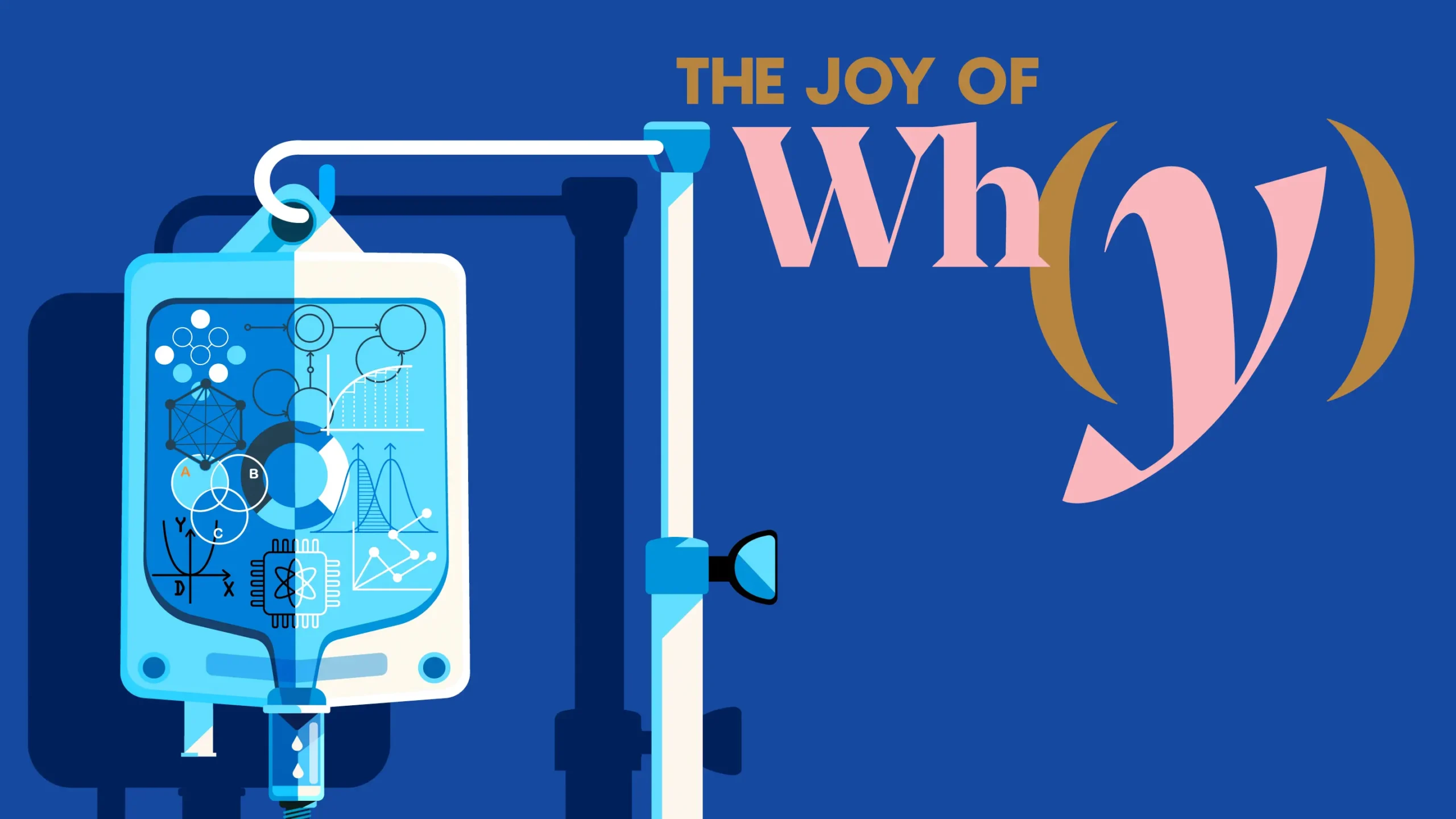
Peter Greenwood for Quanta Magazine
Introduction
When we think about medicine’s war on cancer, treatments such as surgery, radiation and chemotherapy spring to mind first. Now there is another potential weapon for defeating tumors: statistics and mathematical models that can optimize the selection, combination or timing of treatment. Building and feeding these models requires accounting for the complexity of the body, and recognizing that cancer cells are constantly evolving. In this episode, host Steven Strogatz hears from Franziska Michor, a computational biologist, about how our understanding of evolutionary dynamics is being used to devise new anticancer therapies.
Listen on Apple Podcasts, Spotify, TuneIn or your favorite podcasting app, or you can stream it from Quanta.
Transcript
[Theme plays]
STEVEN STROGATZ: Cancer has touched most of us at some point in our lives, whether it’s friends, family or ourselves. It’s the second-leading cause of death in the U.S. today. And though the risk of dying from cancer has steadily declined for decades, the number of cases is on the rise, with 2 million new cancer diagnoses expected by the end of 2024.
These numbers and the grief and havoc they cause can make cancer seem almost invincible. But the best way to fight back is to understand this disease at its most fundamental molecular level, and to use math to our advantage.
I’m Steve Strogatz and this is “The Joy of Why,” a podcast from Quanta Magazine, where I take turns at the mic with my cohost, Janna Levin, exploring some of the biggest questions in math and science today.
In this episode, we’re joined by biologist and mathematician Franziska Michor to ask how cancer has evolved into such a formidable disease, and how machine learning and new statistical models can give us the upper hand.
Franziska is a professor of computational biology at Harvard University and the director of the Dana Farber Cancer Institute Center for Cancer Evolution. She studies the evolutionary dynamics of cancer initiation, progression, response to therapy, and the emergence of resistance.
[Theme ends]
Welcome to the show, Franziska. We’re really happy to have you here.
FRANZISKA MICHOR: Thank you, Steve. I’m so excited to be here.
STROGATZ: Oh, that’s great. Well, let us begin with the basics. What is cancer? Could you explain to us how are cancerous cells different from healthy ones? What’s happening on a molecular level?
MICHOR: Yeah. Cancer cells are cells that proliferate out of control in ways and in places where they shouldn’t be growing. So, if you can imagine the structure and architecture of a normal tissue, it’s very well organized, right? If you look at skin, for instance, there’s different layers. Every cell is exactly where it’s supposed to be. It proliferates exactly when it should, and it doesn’t proliferate out of control and start a clump of cells when it shouldn’t.
Now, if that happens, that’s tumorigenesis: cancer development. And the biggest problem that arises is that these cells can leave their primary site and establish colonies elsewhere. That is called metastatic dissemination. And it’s a really big problem clinically, because as you can imagine, surgery just of the primary site then isn’t curable anymore, if the cells have spread all over the body.
STROGATZ: Mm hmm. Now, so I mentioned in the introduction evolution of cancer. And maybe some of our listeners are thinking evolution of primates, evolution of bacteria. It’s a little unfamiliar to speak about cancer as an evolutionary disease or an evolutionary process. What do we mean by evolution in this sense?
MICHOR: Yeah, that’s a very good question and I think often confuses people. So, evolution is a description of changing populations over time. Now, when we usually think about primate evolution or evolution of zebras or other animals in different ecosystems, we think about evolutionary time as in millions or even billions of years.
What we’re talking about here is the evolution or the changes of cell populations within the life span of a patient. And that then describes how a patient who hopefully is initially healthy, doesn’t have any of the changes that predispose or lead to cancer, how those cell populations then change over time to become more and more aggressive, lead to cancerous growth and eventually disseminate a disease.
So, when we talk about cancer evolution, usually we talk about the somatic evolution. So, evolution that arises throughout the life span of one patient. In somatic cells, so not germline, but in cells throughout the body that are supposed to behave in a very well-controlled manner, but then at some point don’t anymore.
STROGATZ: Let me make sure I get those words you are using: germline versus somatic cells. Just remind us about the distinction?
MICHOR: Yeah, so germline are the sex cells that are passed on to the next generation. Somatic cells are cells that are supposed to just fulfill organ specific tasks — skin cells, heart cells, brain cells. And so these cells are not supposed to be passed on from generation to generation. They’re just within the body of one individual. And when that individual dies, those cells are gone.
STROGATZ: Mm hmm. And so did you say that the somatic cells are the ones that have gone cancerous?
MICHOR: In principle, anything can evolve. That’s the power of evolution, and of evolutionary theory, because it describes so much. So, we can use evolutionary theory to try to understand primate evolution, human evolution, the generation of antibiotic resistance. Viral evolution became really important during the pandemic when we were trying to understand why certain strains of the COVID-19 virus became more abundant.
But we can also use it to describe how cells change over time, and how natural selection acts on those changing populations to increase the frequency of those types of cells that have a fitness advantage, which is something that makes them outcompete some of the other cells, and can give rise to cancer.
STROGATZ: So should I think of the cancer cells outcompeting the normal cells, or are there different strains of cancer cells competing with one another?
MICHOR: All of the above, yes. They can outcompete normal cells, because they grow faster, they have an advantage, they will increase in number over time. But they can also be different clones within the tumor, and this is something that we are increasingly recognizing how heterogeneous each individual tumor really can be, and each individual cancer type even can be. So, when we talk about tumor heterogeneity, that doesn’t just apply to the fact that a tumor from patient A is different from a tumor from patient B. It can also apply to the fact that cell A from patient A is different from cell B from patient A. And so it becomes quite complicated quickly.
STROGATZ: Huh. So we’ve talked a little bit about heterogeneity, and I have a feeling we’ll be coming back to that. I wanted to bring up a different point, a very striking phrase that I encountered, I think maybe in listening to you on the internet, that cancer is a breakdown in the covenant of multicellularity.
MICHOR: Exactly.
STROGATZ: I thought it was an interesting claim. So what does that mean?
MICHOR: So that means really that the body functions because everybody behaves, right? Each cell does what they’re supposed to do. Your retina cells, for instance, they stay in the retina, and they don’t decide one day to march off and sit on top of your nose, right? And so that is a good thing because the body needs to be designed in a certain way in order to function properly.
Now, when that doesn’t work anymore, things can go wrong, and lots of different diseases can come about depending on what exactly goes wrong. But when things proliferate out of control, that’s when we talk about cancer.
Cells don’t behave anymore, they don’t do what they’re supposed to. They become selfish and proliferate out of control, and outcompete other individual cells that are sitting maybe in the same tissue. And so that’s of course a problem. And that’s why it’s very hard to treat. Because usually the differences between a cancer cell and a normal cell are not that many and not that big. It’s not like the difference between a human lung cell and the COVID-19 virus. That’s very different. And so trying to figure out how we treat, if some things are so similar to each other, is actually very tricky.
And that’s why it’s been so hard to come up with medicines that are really capitalizing on these small differences. And in addition, even if we can capitalize on those small differences, it’s not like cancer cells stop evolving. They keep changing even after treatment. And so they can come up with some variants that might be resistant to the drug, even if the drug by itself is very effective.
STROGATZ: Uh-huh. This drug then, by providing its own form of selective pressure on the population, you’re saying, can in effect select what mutants that are able to escape, or at least fare better against this selective pressure of the medicine.
MICHOR: Exactly. So when we think about evolution and the different changes that are being created in a population of cells, it’s not like the cell decides one day, “Oh, I should mutate protein XYZ in order to get away from that mean medicine that was administered here,” right? It’s random changes. But if it so happens that a certain change increases the fitness of a clone, increases the proliferative capacity, even in the presence of that drug, then that clone can increase in frequency, right? And so this is how resistance can get selected for, even in the presence of an otherwise effective therapeutic.
STROGATZ: So we have lots of different treatments these days for cancer. Surgery, radiation, systemic treatments, chemotherapy, immunotherapy. Just roughly speaking, how do all of these kinds of cancer treatments work? What are they doing to interrupt the growth and the spread of cancerous cells?
MICHOR: Yeah. That really depends on which type of drug it is. Radiation, for instance, damages DNA, which has the upside that if there is enough damage, the cell is not able to proliferate, unless it comes up with some rescue strategies to be able to overcome all of the DNA damage that radiation induces. And so therefore, radiation really has been a mainstay of cancer therapy because its mechanism of action is something that can be used against any cell type.
Now there’s other types of drugs. For instance, targeted agents, that identify a specific protein, often a mutated protein, that’s specific to a particular type or subtype of cancer. So a good example is a subtype of lung cancer, which is driven by a mutation in the epidermal growth factor receptor, or EGFR. It’s a specific protein on the surface of cells that, when mutated, tells the cell to grow, grow, grow. Don’t listen to anything, just proliferate, just divide. And so, you can imagine, that this altered protein that’s only present in cancer cells could now be targeted with a small molecule inhibitor that only specifically binds to that mutated protein. And voilà, you have a drug that doesn’t have many side effects because it only binds to things that are present in cancer cells, and it therefore only inhibits cancer cells.
So that’s a great idea. Guess what the catch is?
STROGATZ: I don’t know. So far it sounds very good.
MICHOR: Evolution is the catch, right? So, let’s say there’s all these proliferating cancer cells that are now not being able to proliferate as effectively anymore because they’re being treated with that drug. But now one of them randomly kicks out a mutation that blocks that small molecule inhibitor from binding.
STROGATZ: Oh, I see. So, the target gets itself twisted in some way or puts a little knob on itself that it didn’t used to have or something to make the binding not as sticky or effective.
MICHOR: Exactly. And then, if it’s the right knob or the right thing that sticks out, that could be a resistant subclone. And then, even though maybe the majority of cells are still responding to treatment, that resistant subclone has a chance to grow out and lead to a resurgence of the disease.
STROGATZ: All right. Let’s shift gears to talking about the mathematical side of things now, because you are really a pioneer. I mean, there are other people in this culture of mathematical oncology. But your lab, in particular, really brings a very multidisciplinary approach to cancer research, combining mathematical modeling with bioinformatics, statistics, machine learning, to answer biological questions. How can math help us?
MICHOR: I think math helps us everywhere. And I’m sure I’m preaching to the choir when I say it’s easier to think about complex things if we actually can formulate them as mathematical equations or constructs. And once we have this mathematical construct, we can actually use the tools of math to ask questions. For instance, we have built predictive mathematical modeling strategies to try to describe how such heterogeneous cancer cell populations would respond to treatment, and then to use these mathematical modeling platforms to systematically ask what would be the best possible way to treat this patient or this patient population, that can also be heterogeneous in all kinds of different characteristics.
And that’s a nontrivial question, right? Because there’s lots of ways in which we could treat cancer patients. In our EGFR small molecule–inhibitor example that we just discussed, the question could be, should I take one pill every day of this drug? Or maybe should I take two pills only every second day? Or maybe I should take three pills, but only every third day. Now, the search space of these different hypothetical treatment strategies, or clinical strategies, can become quite large. Because not only is the dose variable, also the time of day is variable. Maybe potentially what I combine these drugs with, maybe I administer in combination with something else, maybe immunotherapy, maybe traditional chemotherapy, maybe radiation. So the search space of potential strategies I could use in the clinic is quickly in the millions.
Now, how would we systematically check through all of that? We could run a million mouse trials, which will be a lot of dead mice and a long time. And in the end of all of this, still the question, whether the mouse model we are using is actually a relevant model system for the human disease that we’re thinking about. Often these mouse models are less heterogeneous. Often the immune system is a little different. Sometimes we’re even using mouse models that don’t have a functioning immune system. So there’s lots of question marks about that.
So one idea that we had was, why don’t we circumvent all that? Why don’t we try, based on all datasets that are available — that could be cell-line datasets, that could be mouse datasets, it could be human datasets, it could be all of the above, genomic aspects, phenotypic aspects, how quickly cells grow in response to different doses of each drug alone and in combination — and build a mathematical model that describes how the number of individual cells changes over time depending on how we treat. And then once we have this parameterized model, we could just ask, “Now what would be the predicted best way of treating this patient population?”
STROGATZ: Well, it sounds marvelous. You mentioned genomic data, cell-line data, mouse data. I mean, it’s one thing to make a model of how mouse cells will respond to a certain treatment, but when you speak of a model to do all of this, a parameterized model, it seems like it’s really an enormous family of parametrized models.
MICHOR: You’re totally right. So obviously the devil is in the detail, right? And it very much depends on which question we are asking. It’s such rigorous science, at least on the data generation side. And on the mathematical side, there’s actually a big aspect that’s almost like an art form. There’s this hugely complex biology with so many different aspects and so many different details that might or might not matter. Part of the reason that physics is so much more evolved — sorry for the pun, that was not intended — in terms of using math to understand phenomena is because you can do isolated experiments, right?
So, you can imagine that if I drop this microphone to the floor, whether it’s raining outside or not will make no difference to the physics of the microphone falling to my office floor. However, if you do a cell line experiment or an experiment in a mouse, whether the mouse is dehydrated or not could actually make a difference to the response to this chemotherapeutic agent. And so it’s very hard to do experiments in isolation as it is easier to do in physics. And so that’s why biological modeling, I think, is a little bit trickier to do than in some other applications, just because it’s such a complex system. And there’s so many interconnected aspects that we really have to try to disentangle, to distill down to the most important aspects that we need to capture as mathematical equations.
STROGATZ: And so, in practice, when you and your group have done this, is there a lot of trial and error in figuring out the right level of detail?
MICHOR: Yeah, there’s some trial and error. We’ve made models that we thought had captured the essential aspects, yet then weren’t able to accurately predict certain behavior. An example that I’m thinking about was a study that we performed in order to identify best radiation schedules for a very aggressive brain cancer, called glioblastoma. And so we had built a model that was relatively simple, just an ordinary differential equation–based system. And we were trying to predict what the best way would be to administer a certain amount of radiation to different cohorts of mice, in which we wanted to validate the predictions of these models.
And what we realized was that most treatment arms were correctly predicted, but we got two arms wrong. We realized that we had made an assumption that was potentially a little bit too simple. And that was that all cells, after being treated with radiation, would wake up at the same time and start growing again at the same time. And that was a little simplistic because cells are different, right? Just like you and I are a little different, each cell is a little different. And so instead of having a certain fixed time at which these cells would come back out of quiescence and start growing again, we needed a distribution of times.
And so we updated the model. And then with model version two, we were able to identify the optimum strategy, which we then validated in mouse trials. So that’s an example of [where] we don’t know a priori what the right level of detail is, right? Sometimes that becomes clearer as you do the research, and as you identify models and make predictions and validate them with actual data. And sometimes we get it wrong. That’s OK.
STROGATZ: I see. It sounds, in a way, like classic scientific method. Because, I mean, now having mentioned machine learning, I was thinking maybe there’s some very modern approach here with the machine coming up with mysterious parameter choices.
MICHOR: Well, that’s one example where we’re doing something based on a dynamical systems approach, right? That’s a little bit different than if we were to have the machine automatically train a whole number of different models to try to identify the best predictive model. Now, which method we use for which question depends on the question, right? It’s not like we have one tool in our toolbox and we are looking for applications across cancer genetics.
STROGATZ: Well, you’re unusual that way, right? You must know a lot of scientists who like one tool. Good for you that you are problem-driven more than tool-driven. I happen to like that style of science myself.
MICHOR: Yeah, I do, too. And in fact, I think it’s the only way to go. Because at the end of the day, we’re trying to make a contribution that makes the world a better place. And so that will drive us to ask different questions over the years and years that we’re working on trying to come up with better treatment strategies and better medicines, better approaches, to help patients in the clinic. Some things need longitudinal statistical methods, some things need bioinformatics methods, some things need a combination of the above, some things need machine learning, but not everything needs machine learning. And so, I think that makes for a very interesting research life. Because we are doing very different things depending on which day you come to visit us, and it definitely isn’t a dull place.
STROGATZ: We’ll be right back after this message.
[Break]
STROGATZ: Welcome back to “The Joy of Why.” Now if you don’t mind, let me ask you a personal question about your own upbringing. Is there something that pointed you in this very remarkable cross-disciplinary direction of combining pretty advanced math with pretty advanced biology or medicine?
MICHOR: Yeah. I am my parents’ daughter, and my mom was a nurse. And she was trained as a newborn nurse, but eventually worked with older patients. And it was very clear that there is so much to be gained from making an impact on patients’ lives, whatever that might be — making them feel better, treating them better, having better medicines. And so the humanitarian aspect was always super important to me because I had this shining example of my mom, who was such a good nurse.
And then my dad was a professor of mathematics at the University of Vienna, and he was very abstract. I remember as a kid sitting there and being told these derivations of some infinite dimensional space, and how you grow teeth on curves, and geodesics and what that all means, and being completely confused. But then finally in the middle of the night, there would be this hammering, “Eureka, I got it!” sounding through the house, waking us all up. And he was so happy. Because the question was clear in his fields. And once the question was clear and there was a proof, and there was a solution to the problem, it was true forever. And so that was super appealing to me.
And then as an undergrad, I started doing experiments in Vienna. And these experiments were really interesting because they produced the primary data, and how exciting is that to actually be looking at the primary data. But very quickly you realize that there could be a completely unknown reason why months and months of work went out the window. And it’s not because you did something wrong. It’s because one of the reagents was maybe outdated, or somebody else had maybe not completely closed the lid. And now I realize that was my fault anyways, and I should have checked better before I used those reagents. But back then, I thought it was really frustrating that things can go wrong, and not because I made a mistake. In my world, things go wrong all the time, but it’s always my fault. I made a coding error, or I forgot about a minus or I forgot my basic calculus. And so, I like the agency that math brings. I like the fact that if I make a mistake, it will be wrong. But if I don’t make a mistake, it will be right. And isn’t that nice?
STROGATZ: I hear you. And of course, as a fellow mathematician, I can resonate with a lot of what you’re saying. I have also been interested in trying to collaborate with biologists. My Ph.D. was on human sleep and circadian rhythms. And the culture of math is so different from the culture of biology and medicine, too. These are three separate cultures. Like the attitude that you have as a mathematician where you say something that your dad could prove is true forever. You know, we’re used to that math is true forever. Science is being turned over all the time. What have been some of the either challenges or exciting aspects of moving into the world of biology and medicine to a point where, I hope, you feel accepted. Is that even the case?
MICHOR: I do. And I have to say, I’m not just a mathematician and not just a biologist. I’m actually very interdisciplinary from the very beginning. So my undergrad already in Vienna was both math and molecular biology. Because I was so interested in both aspects from the very beginning, I feel like I learned both languages simultaneously.
So, I don’t feel like a mathematician going into a biologist’s world, and I don’t feel like a biologist going into a mathematician’s world. I kind of feel very comfortable at this interdisciplinary space, and very excited about the opportunities that this interdisciplinary space presents us with. Because there’s so many questions we can ask that really should be asked, that nobody has asked, maybe because of the language barriers and cultural barriers that you describe between different fields. And that’s been really exciting for us.
STROGATZ: Well, we did briefly talk about how sometimes machine learning is helpful. And I’m wondering, are those tools helping us see cancer and its treatment in new ways? AI and machine learning?
MICHOR: Absolutely. And I think we’re just at the beginning of the revolution that machine learning is going to bring about in cancer biology and in medicine. And there are extremely powerful tools that we can use. And the applicability of those tools, of course, depends on the question that you want to ask. There’s lots of prediction tasks that are quite amenable to machine learning approaches. For instance, in radiology, right? If you are trying to identify which speckle on a CT scan or an MRI actually are tumor versus maybe something else, maybe a bile duct, maybe some other structure. There’s extreme talent that radiologists have, and experience, that they have to deploy in order to accurately disentangle all of these different structures. But maybe that can be aided by machine learning approaches that are trained on large enough, gold-standard labeled data sets, such that maybe later on we can do this automatically. We’re not quite there yet, but I think a lot of progress has been made lately in order to answer questions like that. And so I’m super excited about the future and what these really powerful technologies will be able to bring to the table.
STROGATZ: Is it helping you in your evolutionary dynamics work on cancer?
MICHOR: Yes. There are some interesting applications that we can do. For instance, we can use strategies such as reinforcement learning, which is a particular type of machine learning that penalizes the model if it does badly. Doing badly could mean something like selecting a treatment strategy that doesn’t minimize the tumor cell population as much as possible. And so approaches like that can be used.
But I think one of the hesitations that come with some of the machine learning approaches is that it’s less mechanistically understandable. You can make a prediction model that could be very great, but it might not necessarily give you deeper logical insight into the mechanism of why the prediction is so great. And so that has to be carefully looked at. Some approaches and some interpretation strategies or feature ranking approaches can be used to make that more interpretable. But that’s definitely something that’s on top of our minds.
STROGATZ: Yes. That is certainly a common criticism of machine learning as it stands today, that it doesn’t explain itself yet. So, there is a lot of work now at the cutting edge in so-called explanatory AI. You know, if we could understand what they have in mind when they tune their weights a certain way. It’s hard to know whether we can trust them, at the moment.
MICHOR: Yeah. But there’s ways in which you can identify the top important features that are most important in predicting a particular outcome. And so, ranking those features by importance then allows us to see, for instance, which genes are most important for predicting whether a patient will respond to immunotherapy, which is a project we’re currently working on in the lab.
And so that would be a simpler machine learning approach that might not be the one that gives rise to the best prediction accuracy but comes with the upside of actually being interpretable because we can identify features that are really important for driving the prediction score. And so that’s a tradeoff. Interpretability versus maybe complexity, which could come with better prediction accuracy. But that really needs to be carefully investigated and also tested on independent data to see how robust these strategies are.
STROGATZ: OK. So let us now move from the math side of it to the clinical side. For instance, how do the mathematical models help us predict the development of drug resistance or help us tailor cancer treatment to particular patients or for particular kinds of cancer?
MICHOR: Well, there’s lots of different ways in which we can use data to make predictions about individual scenarios. So, mathematically, we are interested in describing cancer cell populations that are growing and dying as maybe a stochastic process. For instance, a branching process, where we can describe how the cell number changes over time, independent of how quickly cells grow and how quickly they die.
And now the reason this stochastic formulation makes sense is that things happen by chance sometimes. Sometimes when we have a small number of cells, they can die out just by chance, because we are selecting them randomly more for death than for proliferation in a stochastic interpretation like this.
So, we could use a model like that to ask, well, what’s the probability that a certain patient who receives a therapeutic intervention according to a certain administration schedule will come up with resistance? I say probability because given that there’s underlying randomness, not every patient will come up as resistant at exactly the same time, or the resistant clone won’t be exactly equally large. And so, formulating these questions of population dynamics as probabilistic mathematical equations helps us address certain questions. Such as, how long might it take until resistance comes up or what can we do, clinically, such that it takes as long as possible until resistance comes up?
STROGATZ: Mm hmm. So, are we at the stage yet of doing clinical trials on people?
MICHOR: We are at that stage. We have run three clinical trials based on our mathematical modeling framework so far. Two in lung cancer, one in brain cancer. And in all of those scenarios, we have mathematical models that allow us to predict how we should treat in the best possible way. And then we tested that in human patients.
STROGATZ: And so, if I heard you right, you said two trials so far in lung cancer patients, one in brain — glioblastoma or a different …?
MICHOR: Yes.
STROGATZ: OK. And why those two? Is it just those are the first two or is there something uniquely favorable about those forms of cancer for comparison to these predictions?
MICHOR: Yeah, that’s a good question. Brain cancer, I’ve always been interested in just because there’s so little that works. This is a cancer type that’s very aggressive. Median survival is 14 months after diagnosis, even with very aggressive treatment of radiation and chemotherapy and surgery. And while there have been improvements, the overall survival has changed very little in the last 50 years. And so, this is a cancer type that I’m really interested in, just because it’s such a large unmet clinical need. And so, this is one of the cancer types that we focused on in order to understand how we could best administer radiation therapy.
And we touched upon this a little bit earlier, but one of the clinical trials that we ran was to see whether the mathematically derived radiation schedule would actually be administrable in patients. And this comes in many stages. Because if you have a non-standard administration schedule, first you have to show that you can actually give this to patients. And remember, these patients are very sick. It’s hard to bring them in multiple times a day at very specifically defined time points — timing of which matters, as we show in these mathematical modeling approaches. And so, the first approach then is to run a small pilot trial to demonstrate feasibility.
And so that’s what we did. It was called the MARS-Glio trial, here at Brigham and Women’s, at the Dana Farber Cancer Institute, where we treated 14 patients with a schedule that was very non-standard, but derived based on these mathematical models that we built, which were parameterized with mouse data, as well as with human data. And so, we found that this is feasible, that we can really give recurrent glioblastoma patients a very non-standard schedule that involves coming in three times a day for three days in a row for radiation. But these patients were troopers. And, while the trial wasn’t powered for efficacy, so we can’t say whether our schedule was significantly improving survival, we definitely observed that we steered the evolution of these tumors, because we saw a very different pattern of recurrence after they were treated with our optimized schedule, as compared to a standard radiation schedule.
STROGATZ: Recur. What does it mean? Recurrence of tumors where they’re recurring elsewhere in the brain or they shrink and then they come back?
MICHOR: Exactly. So, most tumors shrink; all glioblastomas come back. It’s an incurable disease, which is part of the reason we are so interested in it. But where it comes back gives us insight into how these tumor cells evolve over time and respond to treatment. And so largely they recur within the field of radiation. And what we saw in this trial was that they had a significant enrichment in patients that recurred outside the field of radiation, which means that we were effective at controlling tumor cell growth within the area of radiation.
Yet, there still is this dispersion of tumor cells, which might already have occurred before radiation, such that there is the possibility of recurrence outside the field of radiation. So, there’s much more work to be done, but it definitely makes a difference.
STROGATZ: Well, it is a very tragic, awful disease. I’m thinking of my own mentor, who died of glioblastoma and — like you described the patients as troopers — he had surgery. He used to describe going in front of what he called a linear accelerator to administer the radiation. That seemed kind of amazing to think about, but maybe that is not so unusual. His brain would swell and, I mean, it was really awful. So, thank you for working on this. Anyone who knows about glioblastoma knows it’s just an absolutely dreadful diagnosis.
MICHOR: Yeah, it really is. But there’s lots of hope on the horizon, lots of new agents and different approaches and different strategies that people are using to try to make a difference. We’re working on a project right now that has a way to serially biopsy these tumor cell populations over time, to really see how they change, and how they’re different from each other, and to inform how we should design new drugs and new combinations, in order to actually make progress for this devastating disease. I’m hopeful that there will be progress and scientific insight that can lead to extended survival for this disease.
STROGATZ: Well, let’s talk about these more hopeful things then. Are there trends or breakthroughs on the horizon that you’re excited about now? Are there some you would single out?
MICHOR: I’m really excited about early diagnostics. So there’s the ability to detect early signs of tumors within the bloodstream of a patient. And this is a little bit of a tricky proposition, because lots of stuff is floating around in the bloodstream. But if you fish out the right stuff — and you have good prediction models, which are mostly machine learning approaches — then you can identify fragments of DNA, called tumor-derived circulating DNA, or CT-DNA, that can be used to predict whether a certain patient has a tumor already, even though they’re clinically normal and there isn’t any sign or symptoms of the disease.
And so, I’m excited about that because it moves us from a very reactionary approach, right? Somebody is diagnosed with late-stage cancer. We’re trying to do the best we can, but the disease has spread across the body. It’s really hard to get under control, to maybe a situation in which very early on, we can try to understand whether a patient has a tumor or not, and then tailor treatment when survival chances are much larger.
And so in conjunction with that, I’m also really interested in the early progression. What happens to a normal tissue, changing from normal to maybe precancerous and then cancerous? And can we identify what the drivers of these transitions are and build specific agents, maybe even for cancer prevention, but definitely for intervention, at very early phases? And we can now collect datasets at such big magnitudes, and at single-cell resolution, across so many different patients, to start to be able to understand some of the mechanisms that drive these transitions. And that’s what I’m really excited about.
STROGATZ: It does sound exciting. And so, let me leave you with this last question, then. We are “The Joy of Why.” What is it about your research that brings you joy?
[Theme plays]
MICHOR: It’s a very good question. It brings me joy to discover something about a biological system that is really relevant, which is why I enjoy working on cancer. Because if you find something that could actually make a difference. It could help patients. It could help a real person. The goal of making the world a better place, and the process of going there, brings me great joy.
STROGATZ: Well, that’s marvelous. Thank you. We’ve been speaking with Franziska Michor, professor of computational biology at Harvard University, and it has been just delightful and uplifting to talk to you. Thanks so much for joining us.
MICHOR: Thank you so much. It was my pleasure.
[Theme continues]
STROGATZ: Thanks for listening. If you’re enjoying “The Joy of Why” and you’re not already subscribed, hit the subscribe or follow button where you’re listening. You can also leave a review for the show — it helps people find this podcast.
“The Joy of Why” is a podcast from Quanta Magazine, an editorially independent publication supported by the Simons Foundation. Funding decisions by the Simons Foundation have no influence on the selection of topics, guests or other editorial decisions in this podcast or in Quanta Magazine.
“The Joy of Why” is produced by PRX Productions. The production team is Caitlin Faulds, Livia Brock, Genevieve Sponsler, and Merritt Jacob. The executive producer of PRX Productions is Jocelyn Gonzales. Morgan Church and Edwin Ochoa provided additional assistance. From Quanta Magazine, John Rennie and Thomas Lin provided editorial guidance, with support from Matt Carlstrom, Samuel Velasco, Nona Griffin, Arleen Santana and Madison Goldberg.
Our theme music is from APM Music. Julian Lin came up with the podcast name. The episode art is by Peter Greenwood and our logo is by Jaki King and Kristina Armitage. Special thanks to the Columbia Journalism School and Bert Odom-Reed at the Cornell Broadcast Studios.
I’m your host, Steve Strogatz. If you have any questions or comments for us, please email us at [email protected]. Thanks for listening.
[Theme fades out]