Machine Learning Takes On Antibiotic Resistance
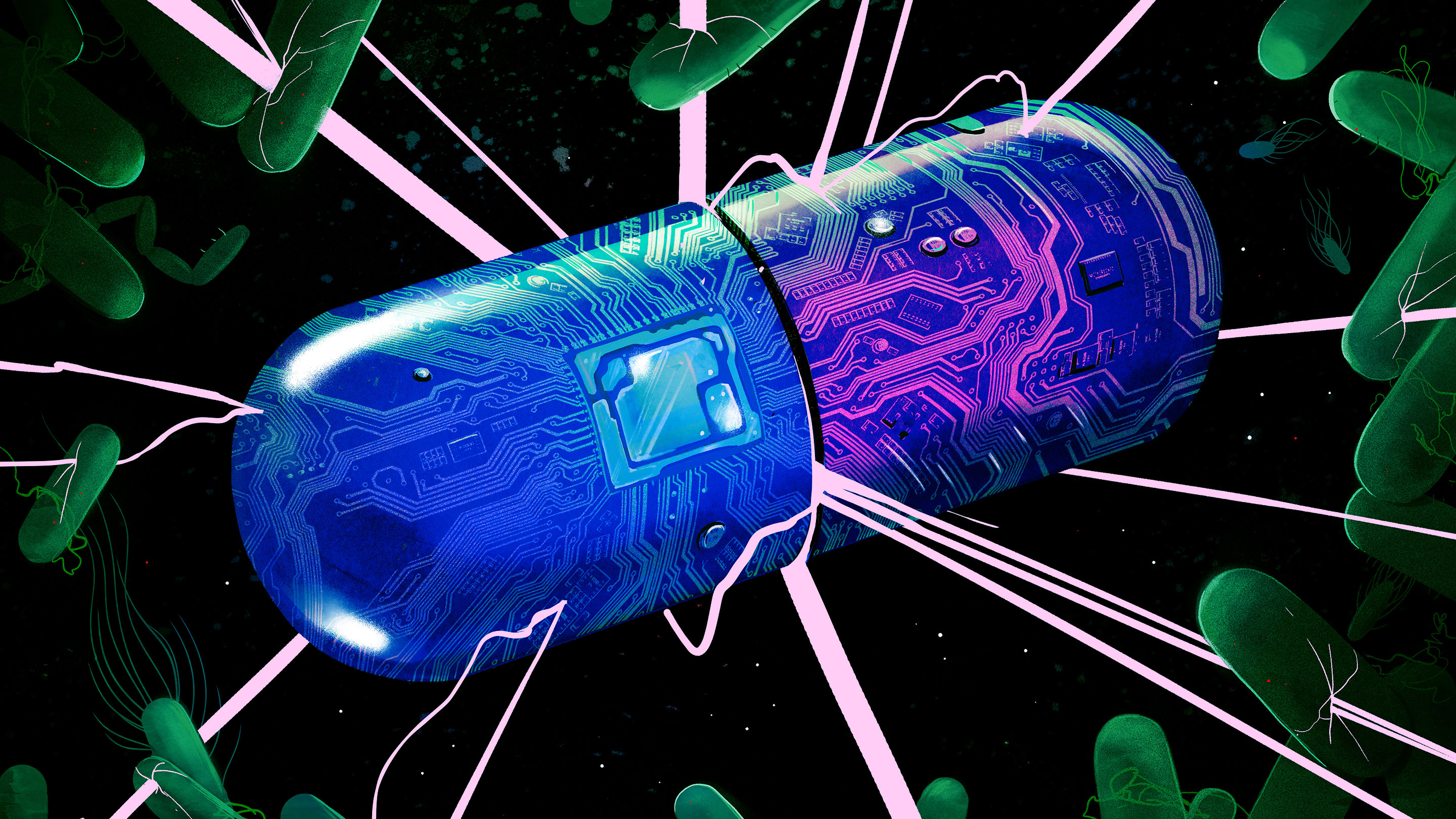
A deep learning neural network has helped to discover a novel antibiotic with an unconventional mechanism of action.
Zoë van Dijk for Quanta Magazine
Introduction
Once-powerful antibiotics are losing their efficacy at a disconcerting pace as bacteria evolve immunity to our drugs. At least 700,000 people around the world now die each year from infections that could formerly be treated with antibiotics. A report last year from the United Nations Interagency Coordination Group on Antimicrobial Resistance warned that if no new major advances are made by 2050, mortality could leap to 10 million deaths a year.
What makes this prognosis all the more dire is that the antibiotic pipeline has slowed to a trickle. In the past two decades, only a few new antibiotics have been found that kill bacteria in novel ways, and rising resistance is a problem for all of them. Meanwhile, traditional methods of identifying antibiotics by screening natural compounds continue to come up short. Because of this, some researchers are now turning from the wet lab to silicon power in hopes of finding an answer.
In the February 20 issue of Cell, one team of scientists announced that they — and a powerful deep learning algorithm — had found a totally new antibiotic, one with an unconventional mechanism of action that allows it to fight infections that are resistant to multiple drugs. The compound was hiding in plain sight (as a possible diabetes treatment) because humans didn’t know what to look for. But the computer did.
Using computers and machine learning to make sense of mountains of biomedical data is nothing new. But the team at the Massachusetts Institute of Technology, led by James Collins, who studies applications of systems biology to antibiotic resistance, and Regina Barzilay, an artificial intelligence researcher, achieved success by developing a neural network that avoids scientists’ potentially limiting preconceptions about what to look for. Instead, the computer develops its own expertise.
With this discovery platform, which has been made freely available, “you’re going to identify molecules that don’t look like antibiotics you’re used to seeing,” Collins said. “It really shows how you can use the emerging technology of deep learning in an innovative manner to discover new chemistries.”
Nature’s Dry Well
Ever since Alexander Fleming derived the first antibiotic from fungus, nature has been the font for our antibacterial drugs. But isolating, screening and synthesizing thousands of natural compounds for laboratory tests is extremely expensive and time-consuming.
To narrow the search, researchers have sought to understand how bacteria live and multiply, and then pursued compounds that attack those processes (such as by damaging bacteria’s cell walls, blocking their reproduction, or inhibiting their protein production). “You start with the mechanisms, and then you reverse-engineer the molecule,” Barzilay said.
Even with the introduction of computer-assisted, high-throughput screening methods in the 1980s, however, progress in antibiotic development was virtually nonexistent in the decades that followed. Screening occasionally turned up drug candidates that were toxic to bacteria, but they were too similar to existing antibiotics to be effective against resistant bacteria. Pharmaceutical companies have since largely abandoned antibiotic development, despite the need, in favor of more lucrative drugs for chronic conditions.
The new work by Barzilay, Collins and their colleagues, however, takes a radically fresh, almost paradoxical approach to drug discovery: It ignores how the medicine works. It’s an approach that can succeed only with the support of extremely powerful computing.
Agnostic Learning
Behind the new antibiotic finding is a deep neural network, in which the nodes and connections of its learning architecture are inspired by the interconnected neurons in the brain. Neural networks, which are adept at recognizing patterns, are deployed across various industries and consumer technologies for uses such as image and speech recognition. Conventional computer programs might screen a library of molecules to find certain defined chemical structures, but neural networks can be trained to learn for themselves which structural signatures might be useful — and then find them.
Collins, Barzilay and their team trained their network to look for any compound that would inhibit the growth of the bacterium Escherichia coli. They did so by presenting the system with a database of more than 2,300 chemical compounds that had known molecular structures and were classified as “hits” or “non-hits” on tests of their ability to inhibit the growth of E. coli. From that data, the neural net learned what atom arrangements and bond structures were common to the molecules that counted as hits.
Because only about 10% of the compounds in the training database were known antibiotics, the neural network wasn’t biased by assumptions about how antibiotic molecules should work or what they should look like (Barzilay describes the platform as mechanism-agnostic). Because of this, it was empowered to find compounds that diverged dramatically from current drugs.
Of course, the ability to inhibit bacteria isn’t the only criterion that matters: Cyanide and arsenic can kill some bacteria, too, but that doesn’t make them useful antibiotics. The researchers therefore also trained the algorithm to predict the toxicity of compounds and to weed out candidate molecules on that basis.
They then turned the trained network loose on the Drug Repurposing Hub, a library of more than 6,000 compounds that are already being vetted for use in humans for a wide variety of conditions. “Using such algorithms, we can start to find essentially new properties in molecules that were used for something completely different,” said César de la Fuente, a presidential assistant professor of bioengineering, microbiology and psychiatry at the University of Pennsylvania Perelman School of Medicine who uses artificial intelligence to try to design new antibiotics. Because it offers a way to repurpose drugs that have already been approved by the FDA, it “would speed up the process to actually translating these molecules to the clinic,” he said.
Collins, Barzilay and their team combined their smart neural-net screening for antibacterial properties with a toxicity screen and then eliminated compounds that they scored as too similar to existing antibiotics, since bacteria would probably be resistant to them already. One drug candidate emerged at the top of the pack: c-Jun N-terminal kinase inhibitor SU3327, which is already being studied as a possible treatment for diabetes. The researchers named the compound halicin (an homage to HAL, the artificial intelligence in 2001: A Space Odyssey).
Testing in the lab revealed that halicin not only stopped the growth of E. coli efficiently but also killed off other bacteria, including Mycobacterium tuberculosis (the cause of tuberculosis), Clostridioides difficile (better known as C. diff, which causes gastrointestinal illness) and a wide variety of other antibiotic-resistant bacteria that cause sepsis, pneumonia, wound infections and other common hard-to-treat infections. Equally promising was that while most antibiotics generate hints of resistance after a matter of days of laboratory testing, halicin failed to produce resistant E. coli mutants after a month of repeated exposure.
It was only after testing halicin in the lab and seeing its wide success as an antibiotic that the researchers began to probe its mechanisms of action. Through RNA sequencing and other experiments, they discovered that it interferes with the movement of protons and the electrochemical potential across bacterial membranes. Reactions dependent on those proton gradients are crucial to cells’ metabolism and mobility, but medicinal chemists had not thought of them as a vulnerability to target in bacteria.
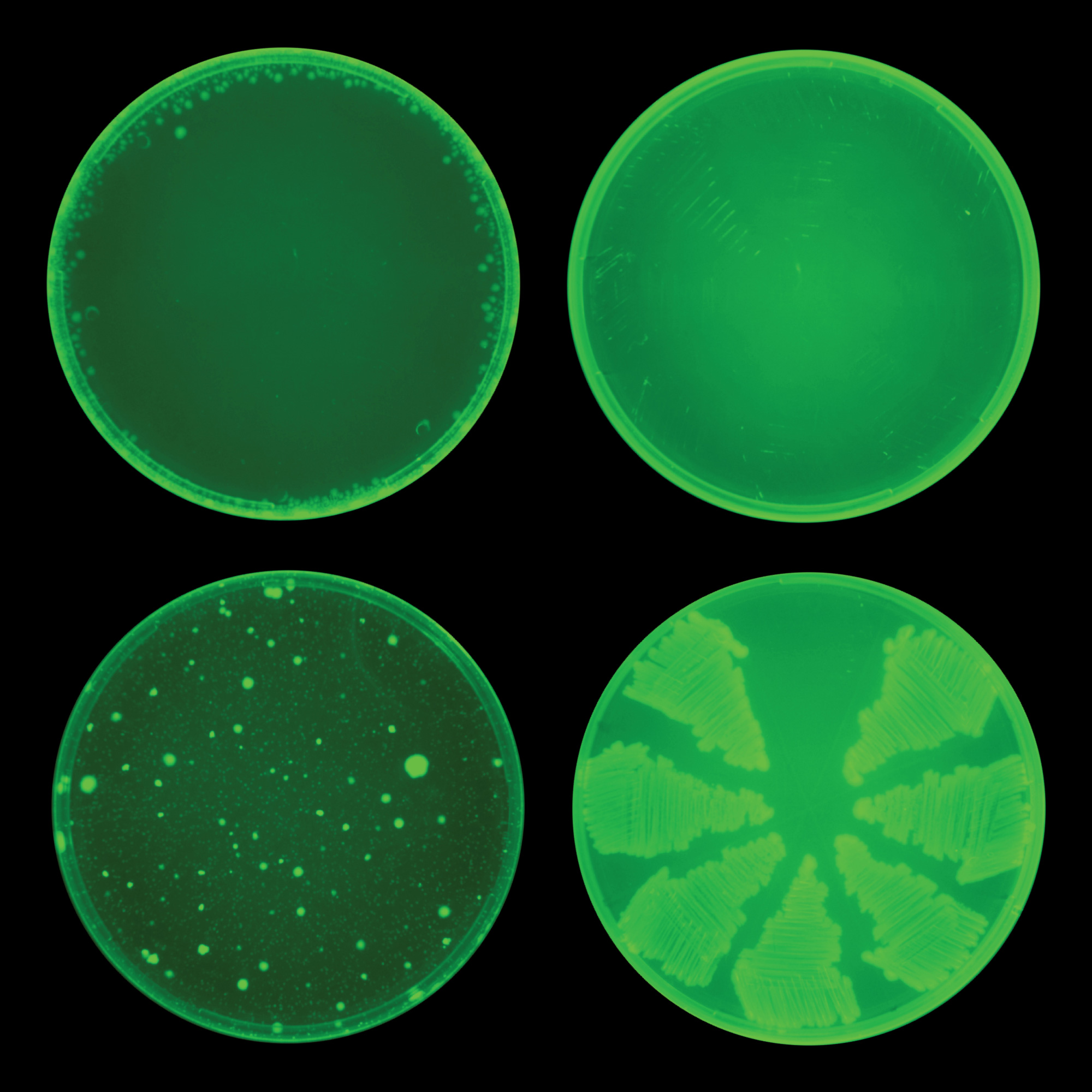
The top two culture dishes are treated with halicin, the novel antibiotic identified by a neural network. The bottom two dishes are treated with ciprofloxacin, a conventional antibiotic. Bacterial growth is greatly reduced in the top dishes because the cells do not seem to become resistant to halicin.
Courtesy of the Collins Lab at MIT
Additional screening of a much larger collection of compounds — more than 107 million of them — with the same trained network brought to light a second promising potential antibiotic. Ordinarily, the idea of screening such a mountain of molecules would be ludicrous: “It would be impossible to physically test over 100 million compounds for antibiotic activity,” said Jacob Durrant, an assistant professor of biological sciences at the University of Pittsburgh and the author of numerous studies on the use of machine learning to fight antibiotic resistance. But the algorithm was able to assess and rank all those molecules in just four days, narrowing the field to just 23 promising contenders for physical testing.
One of them, as yet unnamed, emerged as the best new possible antibiotic from that set. According to Jonathan Stokes, a postdoctoral fellow in Collins’ lab and the first author on the new paper, the tests results showing this compound’s antibacterial prowess came in while the Cell paper was undergoing revisions, so the scientists decided to include it with the halicin announcement. “We are in the early stages of elucidating [a] mechanism” of its action and details about what pathogens it affects and how safe it is for mammalian cells, he explained in an email.
Making Resistance Futile
According to Collins, he and his team are now working to tighten the network’s focus to certain pathogens more specifically. This could lead to the development of more narrow-spectrum antibiotics that would have less impact on the body’s native microbiome, which contributes to health.
In his lab, de la Fuente is looking to accelerate the entire process of antibiotic discovery. His lab combines a machine learning platform that generates new molecules in silico with a synthesizer that prints out these new molecules for a pipetting robot, which then performs experiments on them. The results of those experiments can then feed back into the computer so that it can learn and improve continuously. “That would give us a better shot at having molecules readily available to treat drug-resistant infection,” de la Fuente said.
He and his colleagues are also interested in applying machine learning to drug design rather than simply using it to screen existing compounds. “The hypothesis is that nature has run out of inspiration in terms of providing us with new antibiotics,” de la Fuente said. “That’s why we think that machines … could diversify natural molecules to convert them to synthetic versions that would be much more effective.”
To this end, they use computer models to quickly simulate evolutionary changes, including the effects of mutation, selection and genetic recombination, with feedback loops of that sequence of events. With computers, it should be possible to extrapolate the properties of proteins with novel sequences “that have never really been sampled through evolution,” de la Fuente said. “And that might yield new potential molecules with functions that we haven’t seen before.”
Durrant agreed. “The same approach could be applied to drug discovery efforts targeting other conditions, such as cancer and neurodegenerative diseases,” he said.
Some researchers are deploying machine learning to better understand the resistant microbes themselves. A Nature Communications study from 2018 described helpful patterns generated by a computer algorithm that analyzed 1,595 strains of M. tuberculosis and uncovered unexpected genetic signatures of resistance that could be targeted in the future. As the authors noted, a key feature of this application was “the platform’s ability to generate hypotheses” beyond what human researchers could imagine.
These networks might someday also be able to provide a more holistic picture of bacterial behavior and help scientists understand how environmental influences alter the efficacy of antibiotics, de la Fuente said. Probing immense quantities of data for patterns in nutrient levels, pH, oxygen concentrations and other factors could help point the way to more effective treatments in the future.
The Human Touch
The success of the new algorithm prompted some headline writers to proclaim the dawn of a new era of antibiotics invented by AI. But as a machine-learning expert, Barzilay is quick to qualify those claims. The discovery would not have been possible without thoughtful human work, she noted. “It’s not the machine that invented the molecule. It’s that the machine helped humans to scan the huge space of possibilities and zoom in on the fruitful set of hypotheses that they tested.”
Collins agreed and noted that without quality training data selected by humans, even a strong algorithm can be ineffective or even biased. Additionally, more than one researcher cautioned that the amount of quality data available for training networks to find novel antibiotics is currently limited, which presents a hurdle for future work.
For all its apparent power, in silico work will not replace in vitro testing — and certainly not clinical trials. Even though Collins predicts they will someday “get north of 90%” in predictive accuracy (from their current 51%), subsequent experimentation will continue to be essential.
De la Fuente added, “Whenever you use computers you always reach the same roadblock, which is that … the machine thinks that the molecules it has created will be good — as antibiotics in this case. So we need to validate the molecules” with laboratory experiments.
So the human researchers see these as new computational location tools, probing the deep molecular landscape for unseen potential pharmacological riches.
One of the ultimate successes in this field would be to use AI to discover or design truly “resistance-proof” antibiotics. Although this is one of the projects de la Fuente is currently undertaking, he said that “‘resistance-proof’ is kind of a moonshot goal.” As a microbiologist, he said, “I have much respect for bacteria. … The best we can do is try to keep up with them in this arms race … without thinking that we won the battle.”
This article was reprinted on TheAtlantic.com.