The Physical Process That Powers a New Type of Generative AI
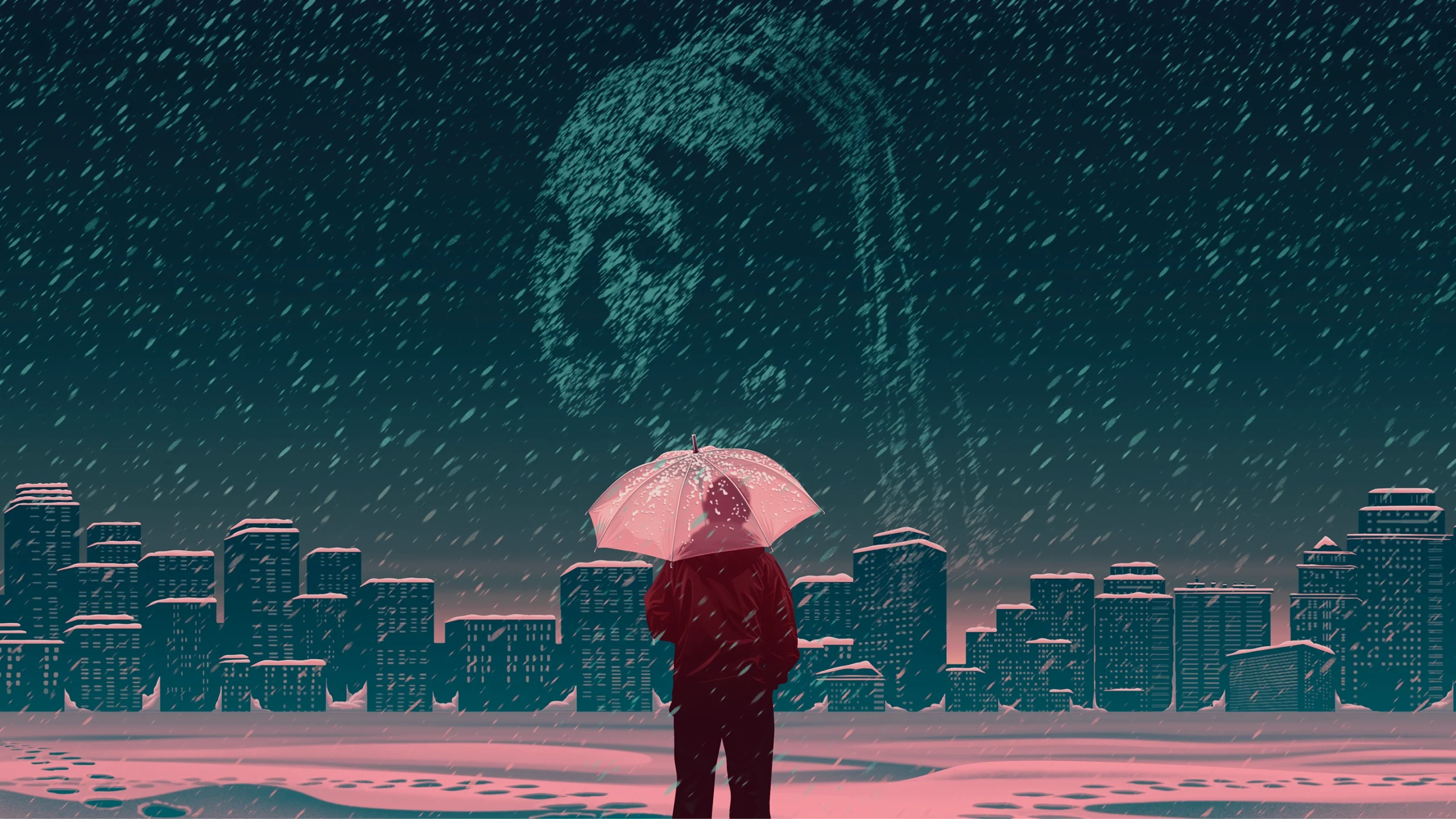
Nash Weerasekera for Quanta Magazine
Introduction
The tools of artificial intelligence — neural networks in particular — have been good to physicists. For years, this technology has helped researchers reconstruct particle trajectories in accelerator experiments, search for evidence of new particles, and detect gravitational waves and exoplanets. While AI tools can clearly do a lot for physicists, the question now, according to Max Tegmark, a physicist at the Massachusetts Institute of Technology, is: “Can we give anything back?”
Tegmark believes that his physicist peers can make significant contributions to the science of AI, and he has made this his top research priority. One way physicists could help advance AI technology, he said, would be to replace the “black box” algorithms of neural networks, whose workings are largely inscrutable, with well-understood equations of physical processes.
The idea is not brand-new. Generative AI models based on diffusion — the process that, for instance, causes milk poured into a cup of coffee to spread uniformly — first emerged in 2015, and the quality of the images they generate has improved significantly since then. That technology powers popular image-producing software such as DALL·E 2 and Midjourney. Now, Tegmark and his colleagues are learning whether other physics-inspired generative models might work as well as diffusion-based models, or even better.
Late last year, Tegmark’s team introduced a promising new method of producing images called the Poisson flow generative model (PFGM). In it, data is represented by charged particles, which combine to create an electric field whose properties depend on the distribution of the charges at any given moment. It’s called a Poisson flow model because the movement of charges is governed by the Poisson equation, which derives from the principle stating that the electrostatic force between two charges varies inversely with the square of the distance between them (similar to the formulation of Newtonian gravity).
That physical process is at the heart of PFGM. “Our model can be characterized almost completely by the strength and direction of the electric field at every point in space,” said Yilun Xu, a graduate student at MIT and co-author of the paper. “What the neural network learns during the training process is how to estimate that electric field.” And in so doing, it can learn to create images because an image in this model can be succinctly described by an electric field.
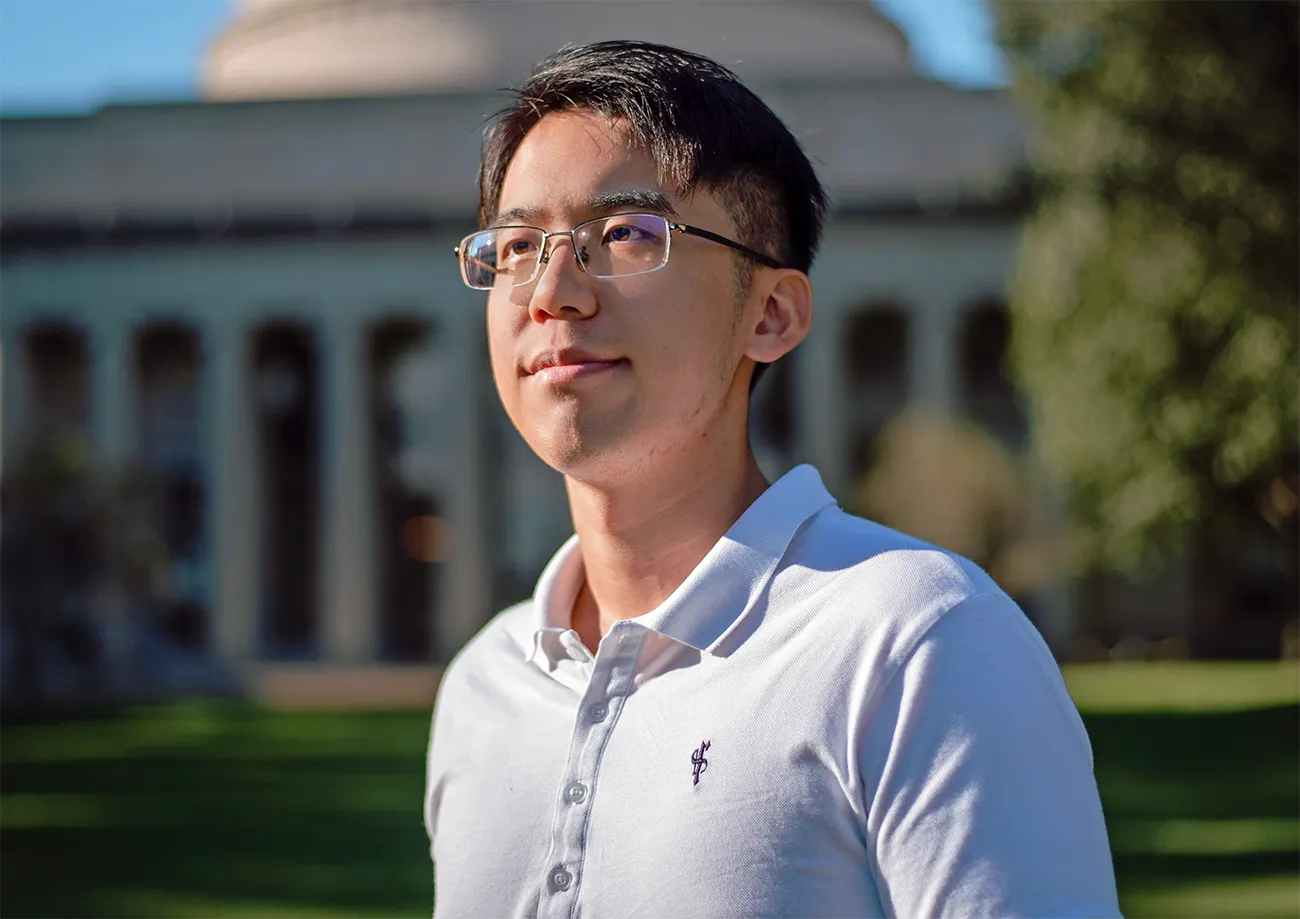
Yilun Xu helped establish a new way for neural networks to create images by exploiting the physical process whereby charges particles produce an electric field.
Tianyuan Zhang
PFGM can create images of the same quality as those produced by diffusion-based approaches and do so 10 to 20 times faster. “It utilizes a physical construct, the electric field, in a way we’ve never seen before,” said Hananel Hazan, a computer scientist at Tufts University. “That opens the door to the possibility of other physical phenomena being harnessed to improve our neural networks.”
Diffusion and Poisson flow models have a lot in common, besides being based on equations imported from physics. During training, a diffusion model designed for image generation typically starts with a picture — a dog, let’s say — and then adds visual noise, altering each pixel in a random way until its features become thoroughly shrouded (though not completely eliminated). The model then attempts to reverse the process and generate a dog that’s close to the original. Once trained, the model can successfully create dogs — and other imagery — starting from a seemingly blank canvas.
Poisson flow models operate in much the same way. During training, there’s a forward process, which involves adding noise, incrementally, to a once-sharp image, and a reverse process in which the model attempts to remove that noise, step by step, until the initial version is mostly recovered. As with diffusion-based generation, the system eventually learns to make images it never saw in training.
But the physics underlying Poisson models is entirely different. Diffusion is driven by thermodynamic forces, whereas Poisson flow is driven by electrostatic forces. The latter represents a detailed image using an arrangement of charges that can create a very complicated electric field. That field, however, causes the charges to spread more evenly over time — just as milk naturally disperses in a cup of coffee. The result is that the field itself becomes simpler and more uniform. But this noise-ridden uniform field is not a complete blank slate; it still contains the seeds of information from which images can be readily assembled.
In early 2023, the team upgraded their Poisson model, extending it to encompass an entire family of models. The augmented version, PFGM++, includes a new parameter, D, which allows researchers to adjust the dimensionality of the system. This can make a big difference: In familiar three-dimensional space, the strength of the electric field produced by a charge is inversely related to the square of the distance from that charge. But in four dimensions, the field strength follows an inverse cube law. And for every dimension of space, and every value of D, that relation is somewhat different.
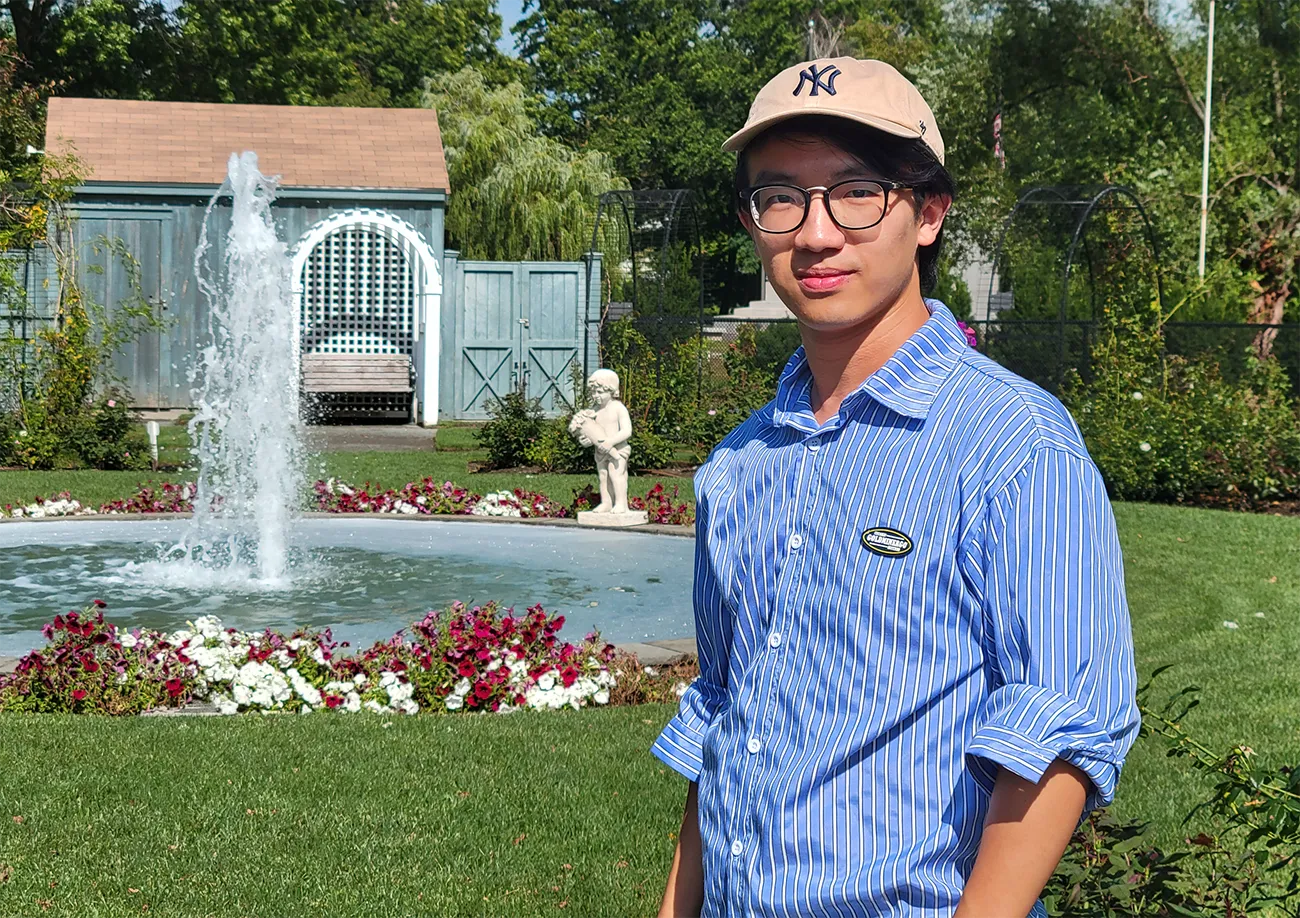
Ziming Liu was also part of the team that expanded PFGM to include multiple possible dimensions, which allows researchers to fine tune a neural network’s robustness and its ease of training.
Wenting Gong
That single innovation gave Poisson flow models far greater variability, with the extreme cases offering different benefits. When D is low, for example, the model is more robust, meaning it is more tolerant of the errors made in estimating the electric field. “The model can’t predict the electric field perfectly,” said Ziming Liu, another graduate student at MIT and co-author of both papers. “There’s always some deviation. But robustness means that even if your estimation error is high, you can still generate good images.” So you may not end up with the dog of your dreams, but you’ll still end up with something resembling a dog.
At the other extreme, when D is high, the neural network becomes easier to train, requiring less data to master its artistic skills. The exact reason isn’t easy to explain, but it owes to the fact that when there are more dimensions, the model has fewer electric fields to keep track of — and hence less data to assimilate.
The enhanced model, PFGM++, “gives you the flexibility to interpolate between those two extremes,” said Rose Yu, a computer scientist at the University of California, San Diego.
And somewhere within this range lies an ideal value for D that strikes the right balance between robustness and ease of training, said Xu. “One goal of future work will be to figure out a systematic way of finding that sweet spot, so we can select the best possible D for a given situation without resorting to trial and error.”
Another goal for the MIT researchers involves finding more physical processes that can provide the basis for new families of generative models. Through a project called GenPhys, the team has already identified one promising candidate: the Yukawa potential, which relates to the weak nuclear force. “It’s different from Poisson flow and diffusion models, where the number of particles is always conserved,” Liu said. “The Yukawa potential allows you to annihilate particles or split a particle into two. Such a model might, for instance, simulate biological systems where the number of cells does not have to stay the same.”
This may be a fruitful line of inquiry, Yu said. “It could lead to new algorithms and new generative models with potential applications extending beyond image generation.”
And PFGM++ alone has already exceeded its inventors’ original expectations. They did not realize at first that when D is set to infinity, their amped-up Poisson flow model becomes indistinguishable from a diffusion model. Liu discovered this in calculations he carried out earlier this year.
Mert Pilanci, a computer scientist at Stanford University, considers this “unification” the most important result stemming from the MIT group’s work. “The PFGM++ paper,” he said, “reveals that both of these models are part of a broader class, [which] raises an intriguing question: Might there be other physical models for generative AI awaiting discovery, hinting at an even grander unification?”