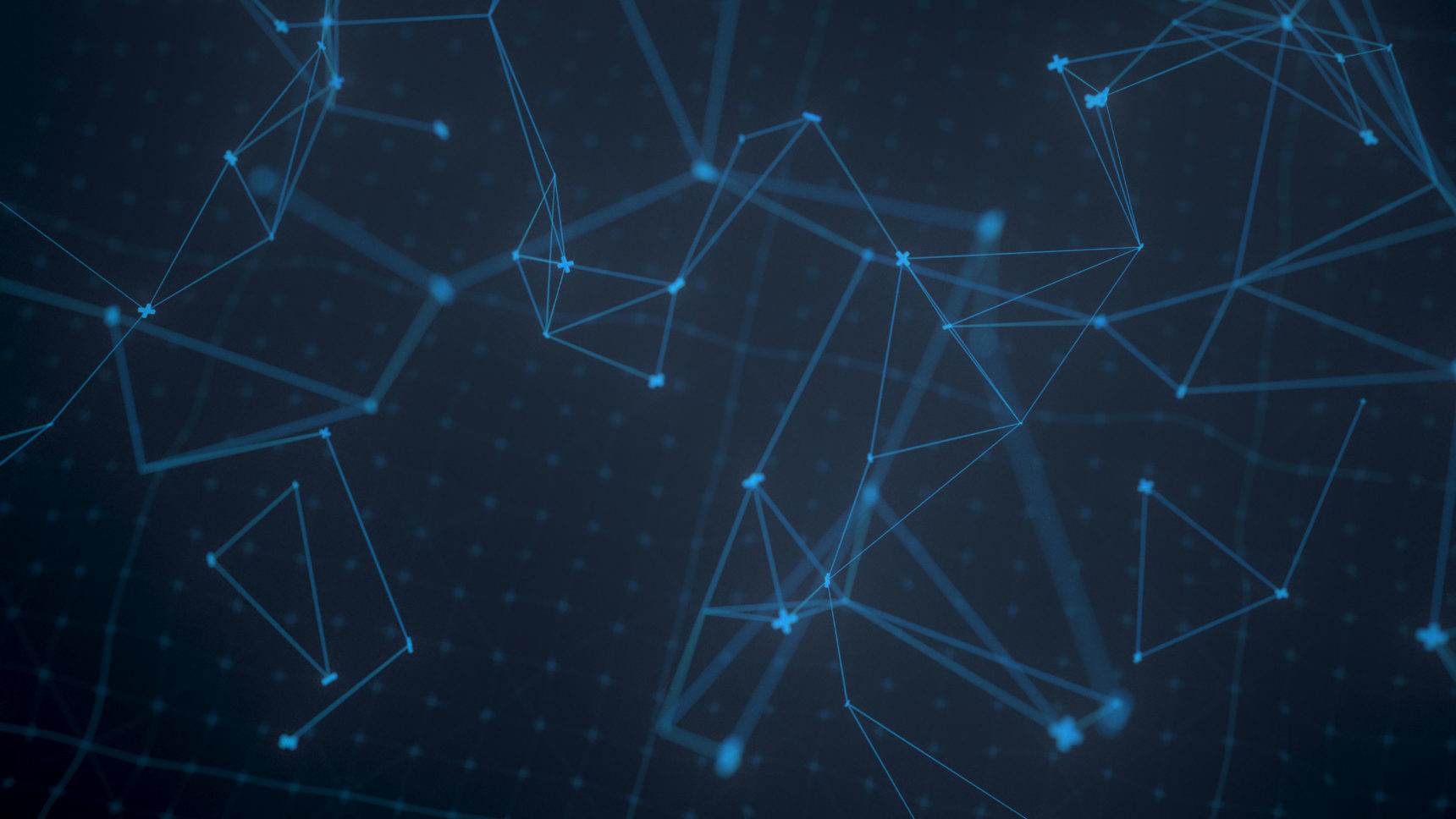
Olena Shmahalo/Quanta Magazine
For mathematicians and computer scientists, this was often a year of double takes and closer looks. Some reexamined foundational principles, while others found shockingly simple proofs, new techniques or unexpected insights in long-standing problems. Some of these advances have broad applications in physics and other scientific disciplines. Others are purely for the sake of gaining new knowledge (or just having fun), with little to no known practical use at this time.
Quanta covered the decade-long effort to rid mathematics of the rigid equal sign and replace it with the more flexible concept of “equivalence.” We also wrote about emerging ideas for a general theory of neural networks, which could give computer scientists a coveted theoretical basis to understand why deep learning algorithms have been so wildly successful.
Meanwhile, ordinary mathematical objects like matrices and networks yielded unexpected new insights in short, elegant proofs, and decades-old problems in number theory suddenly gave way to new solutions. Mathematicians also learned more about how regularity and order arise from chaotic systems, random numbers and other seemingly messy arenas. And, like a steady drumbeat, machine learning continued to grow more powerful, altering the approach and scope of scientific research, while quantum computers (probably) hit a critical milestone.
Building a Bedrock of Understanding
What if the equal sign — the bedrock of mathematics — was a mistake? A growing number of mathematicians, led in part by Jacob Lurie at the Institute for Advanced Study, want to rewrite their field, replacing “equality” with the looser language of “equivalence.” Currently, the foundations of mathematics are built with collections of objects called sets, but decades ago a pair of mathematicians began working with more versatile groupings called categories, which convey more information than sets and more possible relationships than equality. Since 2006, Lurie has produced thousands of dense pages of mathematical machinery describing how to translate modern math into the language of category theory.
More recently, other mathematicians have begun establishing the foundational principles of a field with no prevailing dogma to cast aside: neural networks. The technology behind today’s most successful machine learning algorithms is becoming increasingly indispensable in science and society, but no one truly understands how it works. In January, we reported on the ongoing efforts to build a theory of neural networks that explains how structure could affect a network’s abilities.
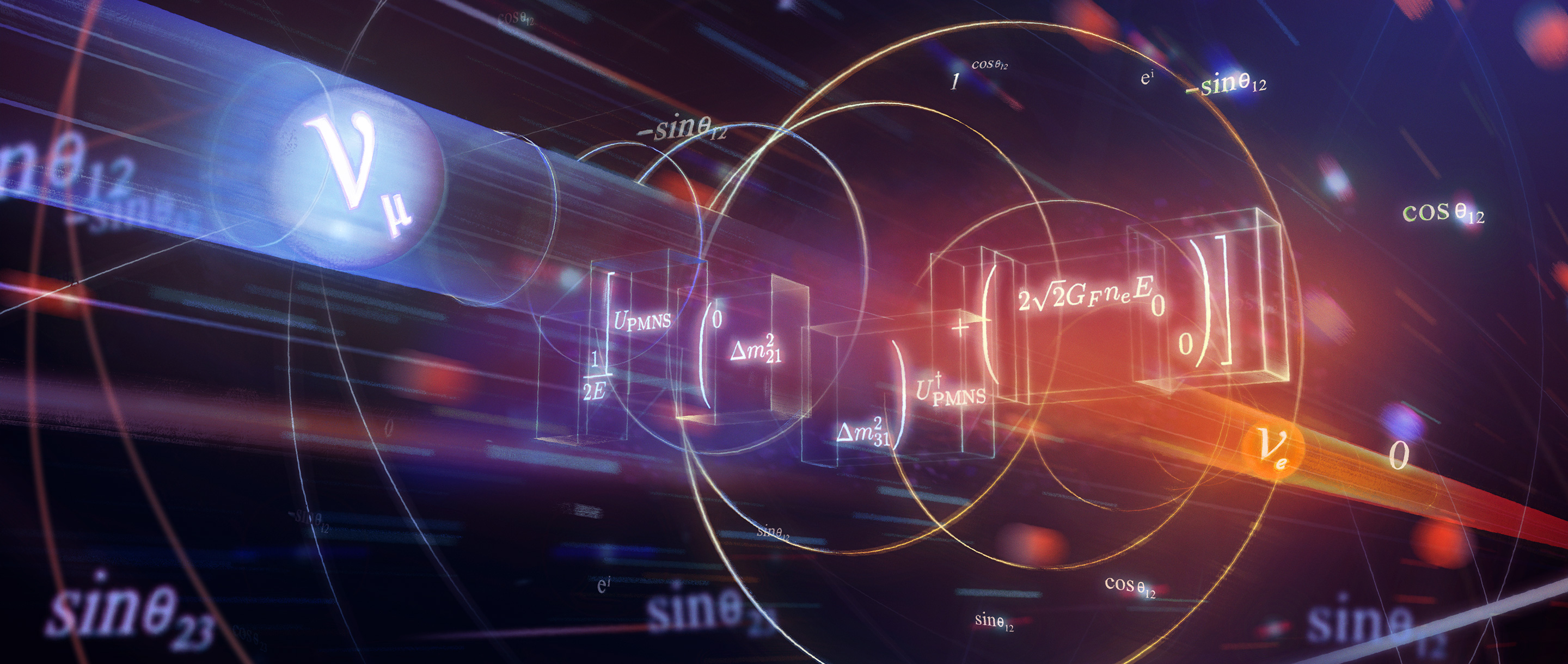
Maciej Rebisz for Quanta Magazine
A New Look at Old Problems
Just because a path is familiar doesn’t mean it can’t still hold new secrets. Mathematicians, physicists and engineers have worked with mathematical terms called “eigenvalues” and “eigenvectors” for centuries, using them to describe matrices that detail how objects stretch, rotate or otherwise transform. In August, three physicists and a mathematician described a simple new formula they’d stumbled upon that relates the two eigen-terms in a new way – one that made the physicists’ work studying neutrinos much simpler while yielding new mathematical insights. After the article’s publication, the researchers learned that the relationship had been discovered and neglected multiple times before.
The familiar also gave way to novel insights in computer science, when a mathematician abruptly solved one of the biggest open problems in the field by proving the “sensitivity” conjecture, which describes how likely you are to affect the output of a circuit by changing a single input. The proof is disarmingly simple, compact enough to be summarized in a single tweet. And in the world of graph theory, another spartan paper (this one weighing in at just three pages) disproved a decades-old conjecture about how best to choose colors for the nodes of a network, a finding that affects maps, seating arrangements and sudokus.
The Signal in the Noise
Mathematics often involves an imposition of order on disorder, a wresting of hidden structures out of the seemingly random. In May, a team used so-called magic functions to show that the best ways of arranging points in eight- and 24-dimensional spaces are also universally optimal – meaning they solve an infinite number of problems beyond sphere packing. It’s still not clear exactly why these magic functions should be so versatile. “There are some things in mathematics that you do by persistence and brute force,” said the mathematician Henry Cohn. “And then there are times like this where it’s like mathematics wants something to happen.”
Others also found patterns in the unpredictable. Sarah Peluse proved that numerical sequences called “polynomial progressions” are inevitable in large enough collections of numbers, even if the numbers are chosen randomly. Other mathematicians showed that under the right conditions, consistent patterns emerge from the doubly random process of analyzing in a random way the shapes produced by random means. Further cementing the link between disorder and meaning, Tim Austin proved in March that all mathematical descriptions of change are, ultimately, a mix of orderly and random systems – and even the orderly ones need a trace of randomness in them. Finally, in the real world, physicists have been working toward understanding when and how chaotic systems, from blinking fireflies to firing neurons, can synchronize and beat as one.
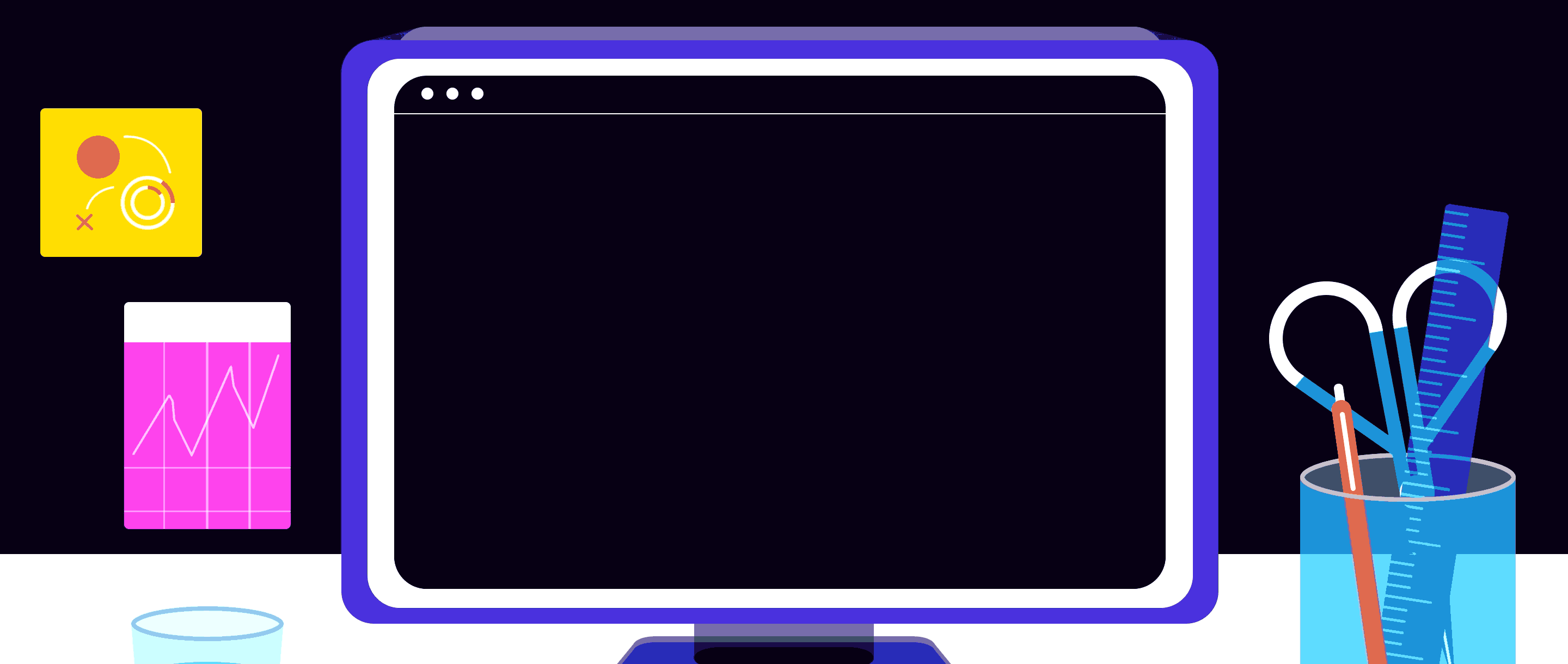
Mengxin Li for Quanta Magazine
Playing With Numbers
We all learned how to multiply in elementary school, but in March, two mathematicians described an even better, faster method. Rather than multiply every digit with every other digit, which quickly grows untenable with big enough numbers, would-be multipliers can combine a series of techniques that includes adding, multiplying and rearranging digits to arrive at a product after significantly fewer steps. This may, in fact, be the most efficient possible way to multiply large numbers.
Other fun insights into the world of numbers this year include finally discovering a way to express 33 as the sum of three cubes, proving a long-standing conjecture about when you can approximate irrational numbers like pi and deepening the connections between the sums and products of a set of numbers.
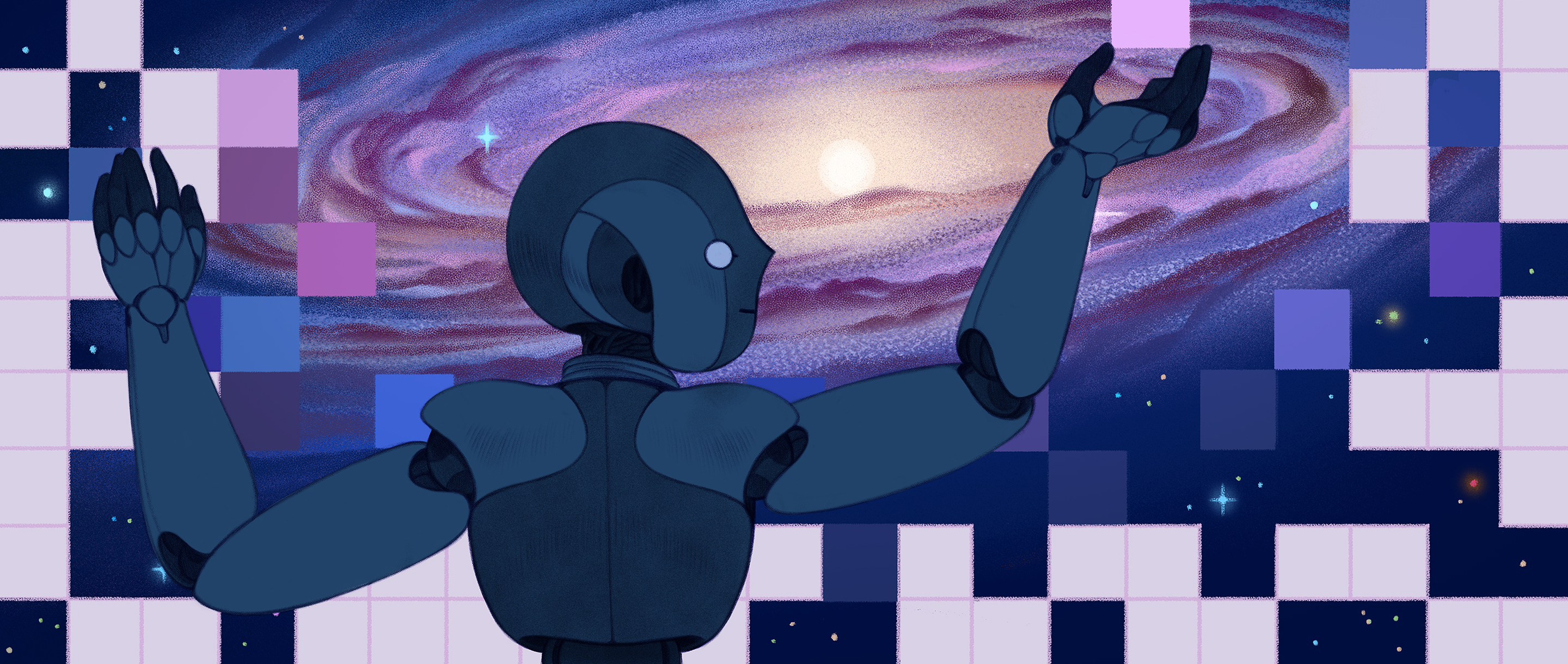
Rachel Suggs for Quanta Magazine
Machine Learning’s Growing Pains
Scientists are increasingly turning to machines for help not just in acquiring data, but also in making sense of it. In March, we reported on the ways machine learning is changing how science is done. A process called generative modeling, for example, may be a “third way” to formulate and test hypotheses, after the more traditional means of observations and simulations – though many still see it as merely an improved method of processing information. Either way, Dan Falk wrote, it’s “changing the flavor of scientific discovery, and it’s certainly accelerating it.”
As for what the machines are helping us learn, researchers announced pattern-finding algorithms that have the potential to predict earthquakes in the Pacific Northwest, and a multidisciplinary team is decoding how vision works by creating a mathematical model based on brain anatomy. But there’s still far to go: A team in Germany announced that machines often fail at recognizing pictures because they focus on textures rather than on shapes, and a neural network nicknamed BERT learned to beat humans at reading comprehension tests, only for researchers to question whether the machine was truly comprehending or just getting better at test-taking.
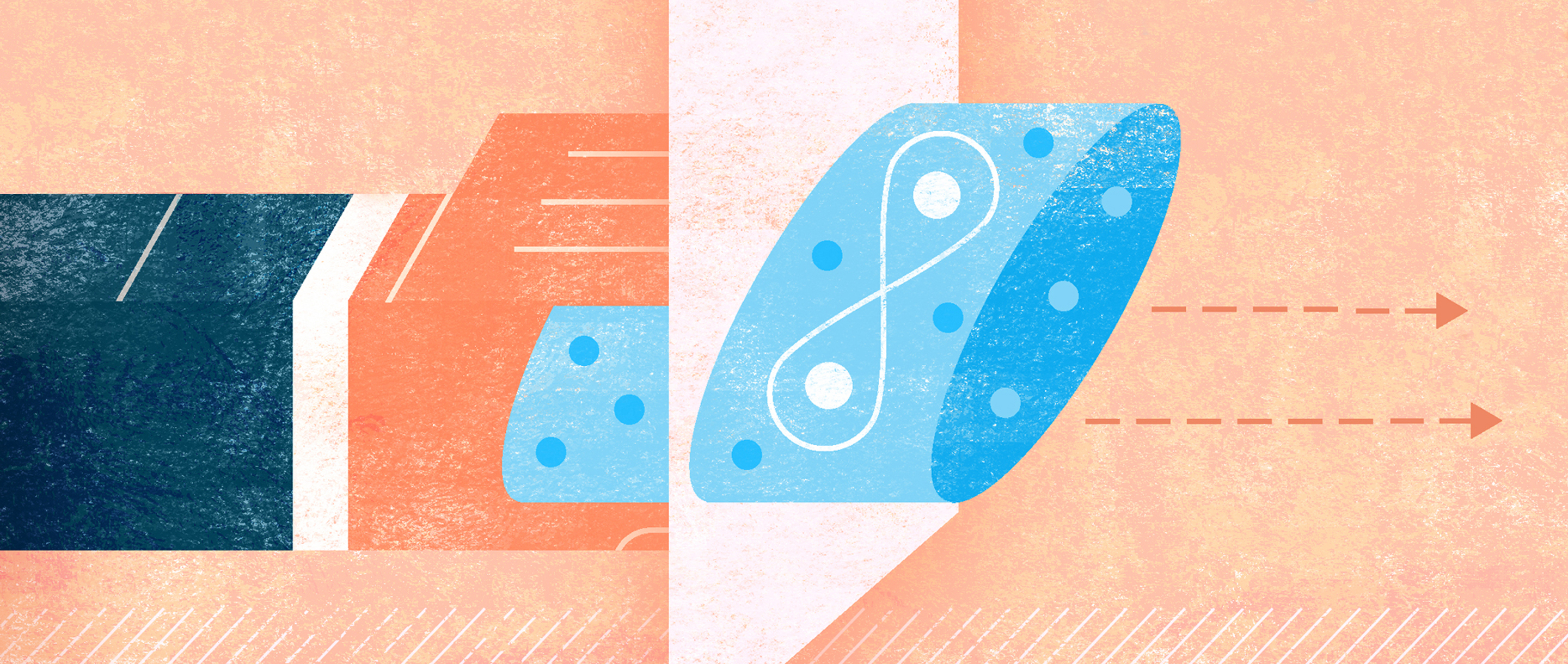
James O’Brien for Quanta Magazine
Next Steps for Quantum Computers
After years of suspense, researchers finally achieved a major quantum computing milestone this year – though as with all things quantum, it’s a development suffused with uncertainty. Regular, classical computers are built from binary bits, but quantum computers instead use qubits, which exploit quantum rules to enhance computational power. In 2012, John Preskill coined the term “quantum supremacy” to describe the point at which a quantum computer outperforms a classical one. Reports of increasingly fast quantum systems led many insiders to suspect we would reach that point this year, and in October Google announced that the moment had finally arrived. A rival tech company, IBM, disagreed, however, arguing that Google’s claim deserved “a large dose of skepticism.” Nevertheless, the clear progress in building viable quantum computers over the years has also motivated researchers like Stephanie Wehner to build a next-generation, quantum internet.