Simpler Math Tames the Complexity of Microbe Networks
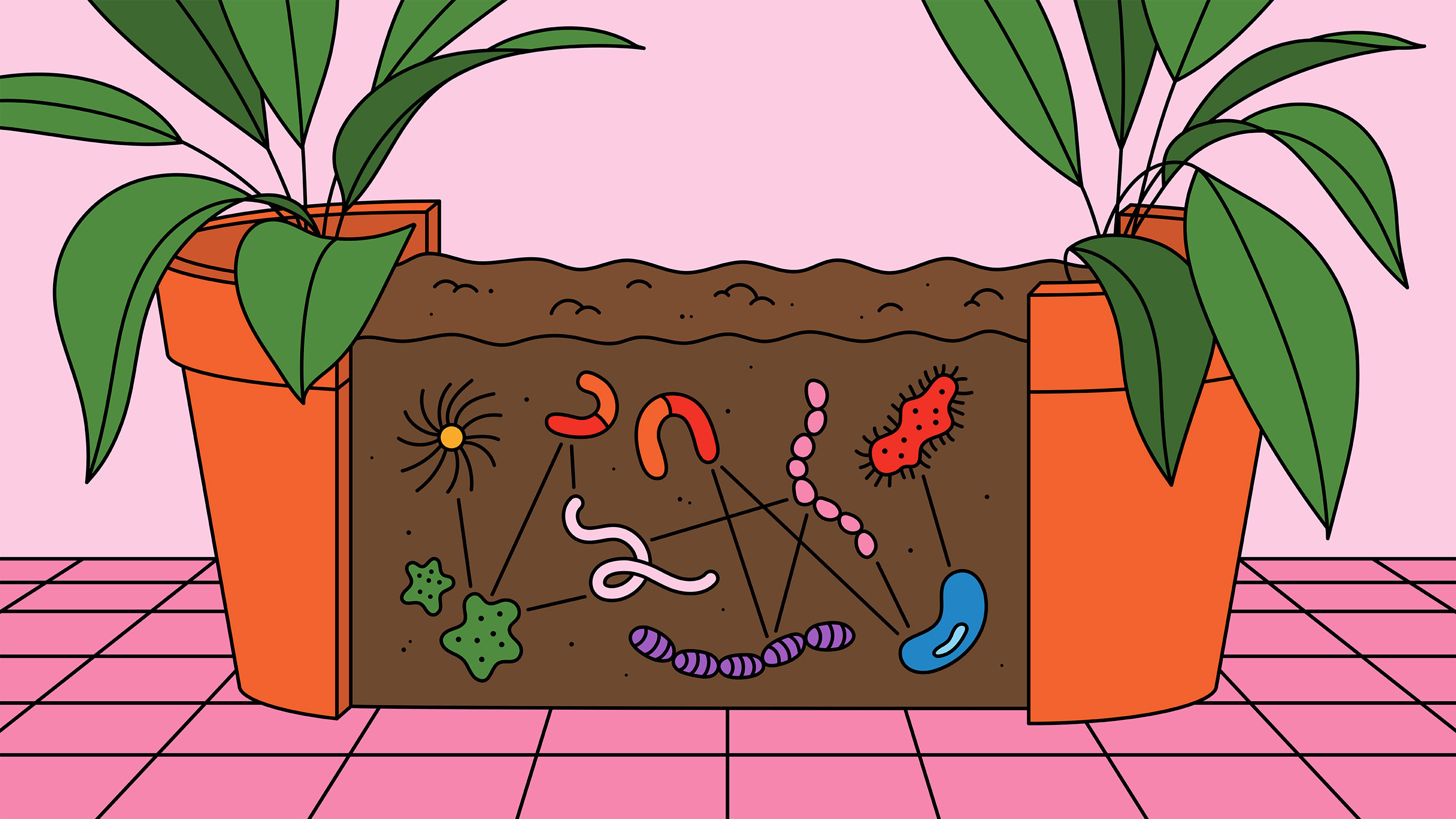
Analyzing the abundant interactions within the communities of microbes that inhabit soil, the intestines and other environments has always been a formidable challenge.
Allison Filice for Quanta Magazine
Introduction
Over the past century, scientists have become adept at plotting the ecological interactions of the diverse organisms that populate the planet’s forests, plains and seas. They have established powerful mathematical techniques to describe systems ranging from the carbon cycles driven by plants to the predator-prey dynamics that dictate the behavior of lions and gazelles. Understanding the inner workings of microbial communities that can involve hundreds or thousands of microscopic species, however, poses a far greater challenge.
Microbes nourish each other and engage in chemical warfare; their behavior shifts with their spatial arrangements and with the identities of their neighbors; they function as populations of separate species but also as a cohesive whole that can at times resemble a single organism. Data collected from these communities reveal incredible diversity but also hint at an underlying, unifying structure.
Scientists want to tease out what that structure might be — not least because they hope one day to be able to manipulate it. Microbial communities help to define ecosystems of all shapes and sizes: in oceans and soil, in plants and animals. Some health conditions correlate with the balance of microbes in a person’s gut, and for a few conditions, such as Crohn’s disease, there are known causal links to onset and severity. Controlling the balance of microbes in different settings might provide new ways to treat or prevent various illnesses, improve crop productivity or make biofuels.
But to reach that level of control, scientists first have to work out all the ways in which the members of any microbial community interact — a challenge that can become incredibly complicated. In a paper published in Nature Communications last month, a team of researchers led by Yang-Yu Liu, a statistical physicist at Harvard Medical School, presented an approach that gets around some — though by no means all — of the formidable obstacles.
Many biologists are highly skeptical about the utility of that approach in practice. But whether or not the method is productive, the new paper joins a growing body of work seeking to make sense of how microbes interact, and to illuminate one of the field’s biggest unknowns: whether the main drivers of change in a microbial community are the microbes themselves or the environment around them.
Gleaning More From Snapshots
“We understand so little about the mechanisms underlying how microbes interact with each other,” said Joao Xavier, a computational biologist at Memorial Sloan Kettering Cancer Center, “so trying to understand this problem using methods that come from data analysis is really important at this stage.”
But current strategies for gaining such insights cannot make use of a wealth of data that have already been collected. Existing approaches require time-series data: measurements taken repeatedly from the same hosts or communities over long stretches of time. Starting with an established model of population dynamics for one species, scientists can use those measurements to test assumptions about how certain species affect others over time, and based on what they find out, they then adjust the model to fit the data.
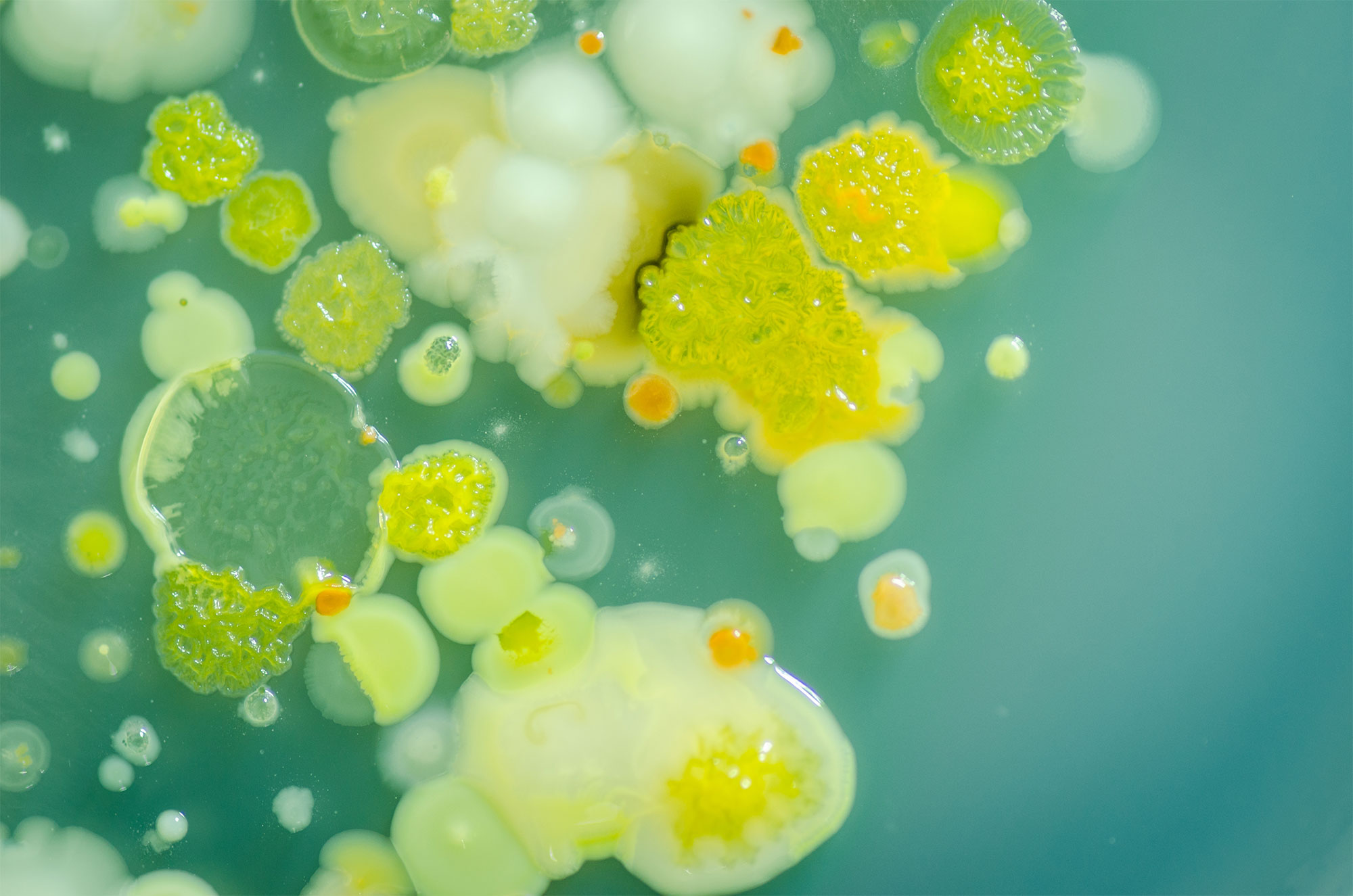
In diverse communities of growing bacteria, the number of potential interactions among them rapidly becomes astronomical as the number of species increases. Measuring the effects of those interactions over time has also been impractical in many real-world systems.
Such time-series data are difficult to obtain, and a lot is needed to get results. Moreover, the samples are not always informative enough to yield reliable inferences, particularly in relatively stable microbial communities. Scientists can get more informative data by adding or removing microbial species to perturb the systems — but doing so poses ethical and practical issues, for example, when studying the gut microbiota of people. And if the underlying model for a system isn’t a good fit, the subsequent analysis can go very far astray.
Because gathering and working with time-series data are so difficult, most measurements of microbes — including the information collected by the Human Microbiome Project, which characterized the microbial communities of hundreds of individuals — tend to fall into a different category: cross-sectional data. Those measurements serve as snapshots of separate populations of microbes during a defined interval, from which a chronology of changes can be inferred. The trade-off is that although cross-sectional data are much more readily available, inferring interactions from them has been difficult. The networks of modeled behaviors they yield are based on correlations rather than direct effects, which limits their usefulness.
Imagine two types of microbes, A and B: When the abundance of A is high, the abundance of B is low. That negative correlation doesn’t necessarily mean that A is directly detrimental to B. It could be that A and B thrive under the opposite environmental conditions, or that a third microbe, C, is responsible for the observed effects on their populations.
But now, Liu and his colleagues claim that cross-sectional data can say something about direct ecological interactions after all. “A method that doesn’t need time-series data would create a lot of possibilities,” Xavier said. “If such a method works, it would open up a bunch of data that’s already out there.”
A Simpler Framework
Liu’s team sifts through those mountains of data by taking a simpler, more fundamental approach: Rather than getting caught up in measuring the specific, finely calibrated effects of one microbial species on another, Liu and his colleagues characterize those interactions with broad, qualitative labels. The researchers simply infer whether the interactions between two species are positive (species A promotes the growth of species B), negative (A inhibits the growth of B) or neutral. They determine those relationships in both directions for every pair of species found in the community.
Liu’s work builds on prior research that used cross-sectional data from communities that differ by only a single species. For instance, if species A grows alone until it reaches an equilibrium, and then B is introduced, it is easy to observe whether B is beneficial, harmful or unrelated to A.
The great advantage of Liu’s technique is that it allows relevant samples to differ by more than one species, heading off what would otherwise be an explosion in the number of samples needed. In fact, according to his study’s findings, the number of required samples scales linearly with the number of microbial species in the system. (By comparison, with some popular modeling-based approaches, the number of samples needed increases with the square of the number of species in the system.) “I consider this really encouraging for when we talk about the network reconstruction of very large, complex ecosystems,” Liu said. “If we collect enough samples, we can map the ecological network of something like the human gut microbiota.”
Those samples allow scientists to constrain the combination of signs (positive, negative, zero) that broadly define the interactions between any two microbial strains in the network. Without such constraints, the possible combinations are astronomical: “If you have 170 species, there are more possibilities than there are atoms in the visible universe,” said Stefano Allesina, an ecologist at the University of Chicago. “The typical human microbiome has more than 10,000 species.” Liu’s work represents “an algorithm that, instead of exhaustively searching among all possibilities, pre-computes the most informative ones and proceeds in a much quicker way,” Allesina said.
Perhaps most important, with Liu’s method, researchers don’t need to presuppose a model of what the interactions among microbes might be. “Those decisions can often be quite subjective and open to conjecture,” said Karna Gowda, a postdoctoral fellow studying complex systems at the University of Illinois, Urbana-Champaign. “The strength of this study [is that] it gets information out of the data without resorting to any particular model.”
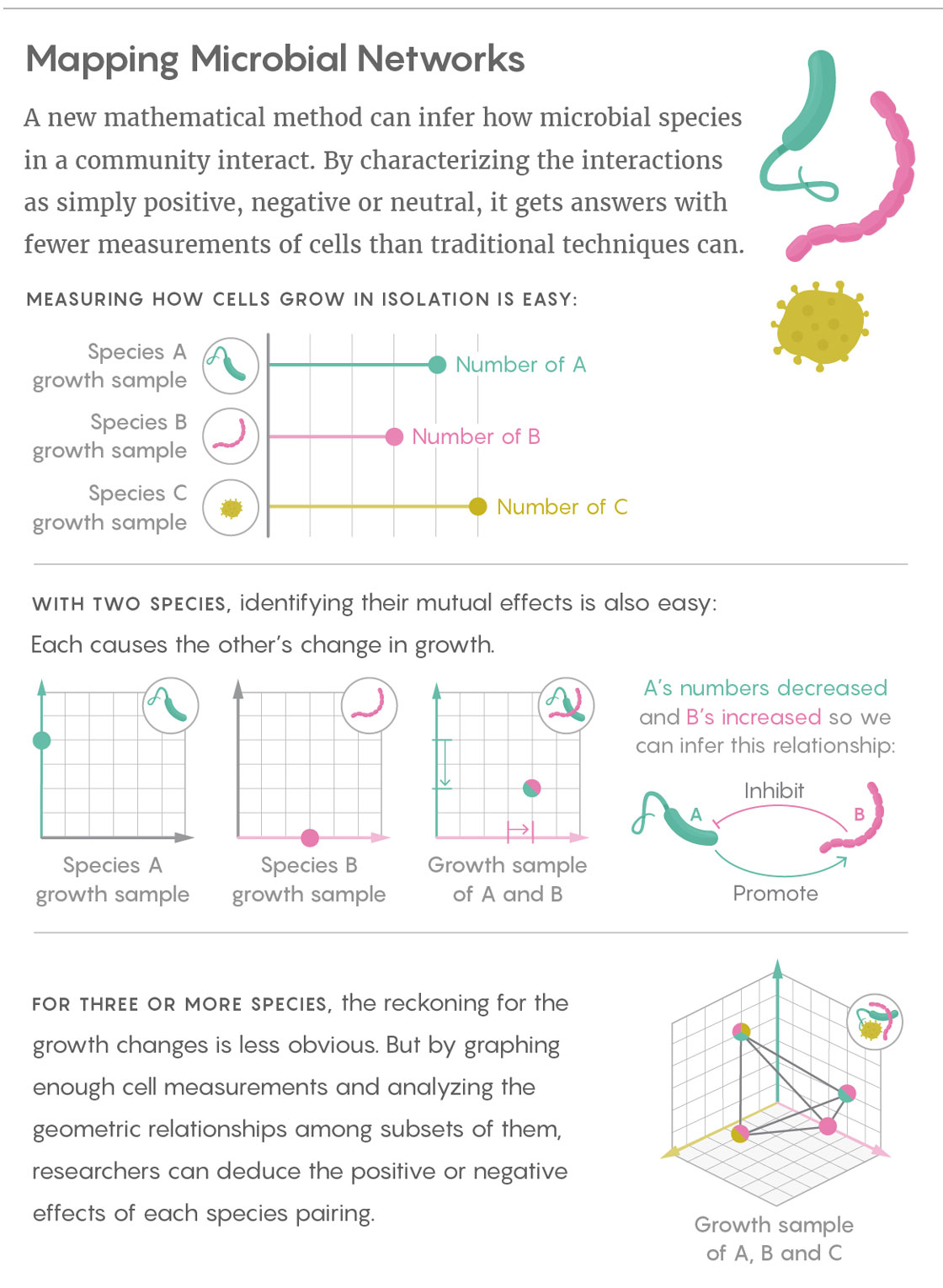
Lucy Reading-Ikkanda/Quanta Magazine
Instead, scientists can use the method to verify when a certain community’s interactions follow the equations of classical population dynamics. In those cases, the technique allows them to infer the information their usual methods sacrifice: the specific strengths of those interactions and the growth rates of species. “We can get the real number, not just the sign pattern,” Liu said.
In tests, when given data from microbial communities of eight species, Liu’s technique generated networks of inferred interactions that included 78 percent of those that Jonathan Friedman, a systems biologist at the Hebrew University of Jerusalem and one of Liu’s co-authors, had identified in a previous experiment. “It was better than I expected,” Friedman said. “The mistakes it made were when the real interactions I had measured were weak.”
Liu hopes to eventually use the method to make inferences about communities like those in the human microbiome. For example, he and some of his colleagues posted a preprint on biorxiv.org in June that detailed how one could identify the minimum number of “driver species” needed to push a community toward a desired microbial composition.
A Greater Question
Realistically, Liu’s goal of fine-tuning microbiomes lies far in the future, if ever. His approach is hampered by severe technical limitations: For instance, it relies on data about the absolute abundance of each species in a sample, which is difficult to get — indeed, nearly all the microbiome data ever collected record relative abundances. The kind of genomic sequencing done in these experiments is also not ideal for accurately classifying microbes by species or other taxonomic grouping.
Moreover, some scientists have more fundamental conceptual reservations — ones that tap into a much larger question: Are changes in the composition of a microbial community mainly due to the interactions between the microbes themselves, or to the perturbations in their environment?
Some scientists think it’s impossible to gain valuable information without taking environmental factors into account, which Liu’s method does not. “I’m a bit skeptical,” said Pankaj Mehta, a biophysicist at Boston University. He is doubtful because the method assumes that the relationship between two microbial strains does not change as their shared environment does. If that’s indeed the case, Mehta said, then the method would be applicable. “It would be really exciting if what they’re saying is true,” he said. But he questions whether such cases will be widespread, pointing out that microbes might compete under one set of conditions but help each other in a different environment. And they constantly modify their own surroundings by means of their metabolic pathways, he added. “I’m not sure how you can talk about microbial interactions independent of their environment.”
A more sweeping criticism was raised by Alvaro Sanchez, an ecologist at Yale University who has collaborated with Mehta on mechanistic, resource-based models. He emphasized that the environment overwhelmingly determines the composition of microbial communities. In one experiment, he and his colleagues began with 96 completely different communities. When all were exposed to the same environment, Sanchez said, over time they tended to converge on having the same families of microbes in roughly the same proportions, even though the abundance of each species within the families varied greatly from sample to sample. And when the researchers began with a dozen identical communities, they found that changing the availability of even one sugar as a resource created entirely divergent populations. “The new composition was defined by the carbon [sugar] source,” Sanchez said.
The effects of the microbes’ interactions were drowned out by the environmental influences. “The structure of the community is determined not by what’s there but by the resources that are put in … and what [the microbes] themselves produce,” Mehta said.
That’s why he’s unsure how well Liu’s work will translate into studies of microbiomes outside the laboratory. Any cross-sectional data taken for the human microbiome, he said, would be influenced by the subjects’ different diets.
Liu, however, says this wouldn’t necessarily be the case. In a study published in Nature in 2016, he and his team found that human gut and mouth microbiomes exhibit universal dynamics. “It was a surprising result,” he said, “to have strong evidence of healthy individuals having a similar universal ecological network, despite different diet patterns and lifestyles.”
His new method may help bring researchers closer to unpacking the processes that shape the microbiome — and learning how much of them depends on the species’ relationships rather than the environment.
Researchers in both camps can also work together to provide new insights into microbial communities. The network approach taken by Liu and others, and the more detailed metabolic understanding of microbial interactions, “represent different scales,” said Daniel Segrè, a professor of bioinformatics at Boston University. “It’s essential to see how those scales relate to each other.” Although Segrè himself focuses on molecular, metabolism-based mappings, he finds value in gaining an understanding of more global information. “It’s like, if you know a factory is producing cars, then you also know it has to produce engines and wheels in certain fixed proportions,” he said.
Such a collaboration could have practical applications, too. Xavier and his colleagues have found that the microbiome diversity of cancer patients is a huge predictor of their survival after a bone marrow transplant. The medical treatments that precede transplant — acute chemotherapy, prophylactic antibiotics, irradiation — can leave patients with microbiomes in which one microbe overwhelmingly dominates the composition. Such low diversity is often a predictor of low patient survival: According to Xavier, his colleagues at Sloan Kettering have found that the lowest microbial diversity can leave patients with five times the mortality rate seen in patients with high diversity.
Xavier wants to understand the ecological basis for that loss of microbial diversity, in the hopes of designing preventive measures to maintain the needed variability or interventions to reconstitute it. But to do that, he also needs the information Liu’s method provides about microbial interactions. For example, if a patient takes a narrow-spectrum antibiotic, might that affect a broader spectrum of microbes because of ecological dependencies among them? Knowing how an antibiotic’s effects could propagate throughout a microbial network could help physicians determine whether the drug could cause a huge loss to a patient’s microbiome diversity.
“So both the extrinsic perturbation and the intrinsic properties of the system are important to know,” Xavier said.
Correction: This article was updated on February 14 to be more explicit about the reservations that many scientists have concerning the usefulness of the approach for describing the interactions in microbial networks. The subhead of the article was also modified to better reflect that skepticism.
This article was reprinted on Wired.com.