The AI Tools Making Images Look Better
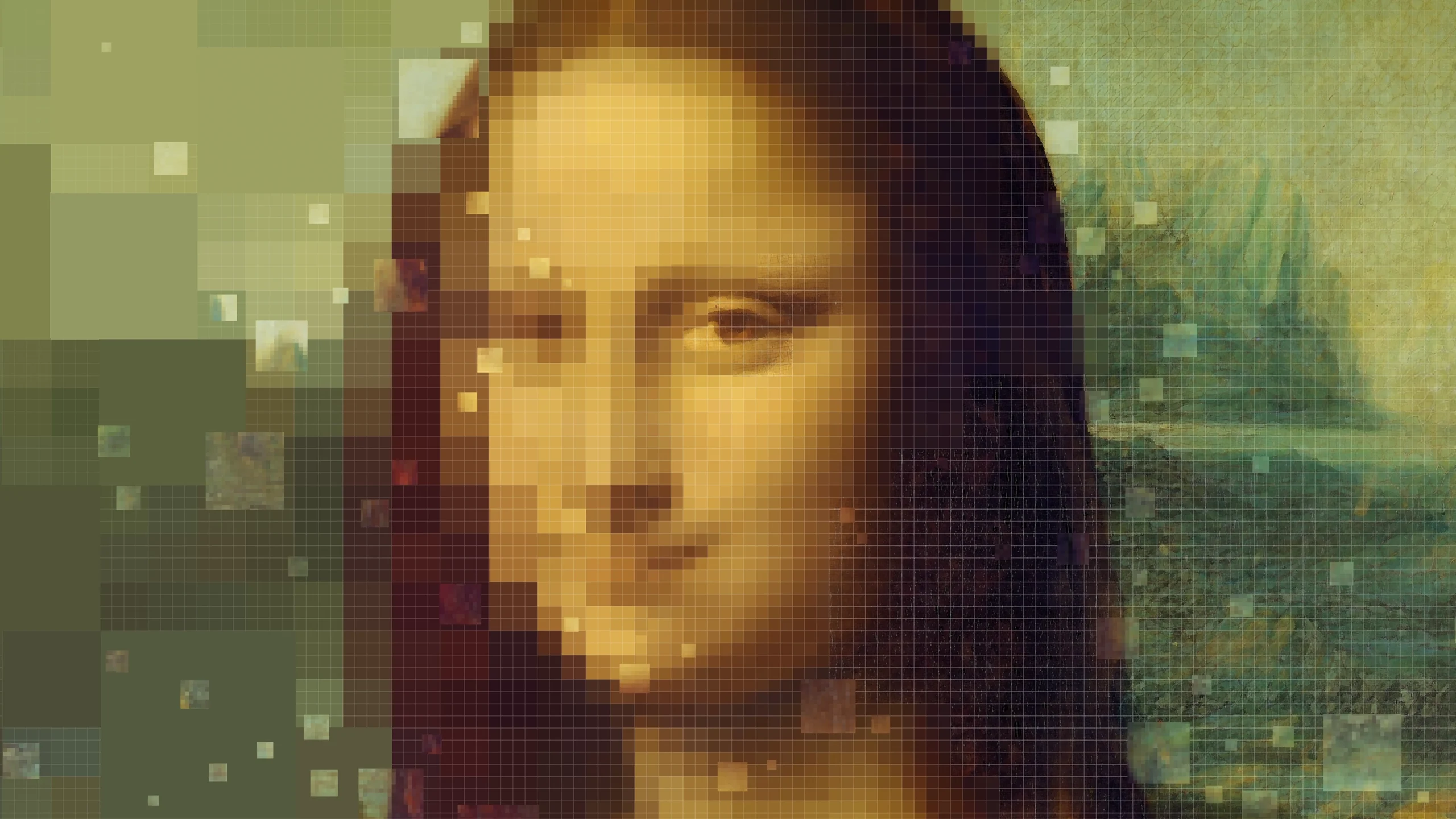
Allison Li/Quanta Magazine; source: C2RMF
Introduction
It’s one of the biggest cliches in crime and science fiction: An investigator pulls up a blurry photo on a computer screen and asks for it to be enhanced, and boom, the image comes into focus, revealing some essential clue. It’s a wonderful storytelling convenience, but it’s been a frustrating fiction for decades — blow up an image too much, and it becomes visibly pixelated. There isn’t enough data to do more.
“If you just naïvely upscale an image, it’s going to be blurry. There’s going to be a lot of detail, but it’s going to be wrong,” said Bryan Catanzaro, vice president of applied deep learning research at Nvidia.
Recently, researchers and professionals have begun incorporating artificial intelligence algorithms into their image-enhancing tools, making the process easier and more powerful, but there are still limits to how much data can be retrieved from any image. Luckily, as researchers push enhancement algorithms ever further, they are finding new ways to cope with those limits — even, at times, finding ways to overcome them.
In the past decade, researchers started enhancing images with a new kind of AI model called a generative adversarial network, or GAN, which could produce detailed, impressive-looking pictures. “The images suddenly started looking a lot better,” said Tomer Michaeli, an electrical engineer at the Technion in Israel. But he was surprised that images made by GANs showed high levels of distortion, which measures how close an enhanced image is to the underlying reality of what it shows. GANs produced images that looked pretty and natural, but they were actually making up, or “hallucinating,” details that weren’t accurate, which registered as high levels of distortion.
Michaeli watched the field of photo restoration split into two distinct sub-communities. “One showed nice pictures, many made by GANs. The other showed data, but they didn’t show many images, because they didn’t look nice,” he said.
In 2017, Michaeli and his graduate student Yochai Blau looked into this dichotomy more formally. They plotted the performance of various image-enhancement algorithms on a graph of distortion versus perceptual quality, using a known measure for perceptual quality that correlates well with humans’ subjective judgment. As Michaeli expected, some of the algorithms resulted in very high visual quality, while others were very accurate, with low distortion. But none had both advantages; you had to pick one or the other. The researchers dubbed this the perception-distortion trade-off.
Michaeli also challenged other researchers to come up with algorithms that could produce the best image quality for a given level of distortion, to allow fair comparisons between the pretty-picture algorithms and the nice-stats ones. Since then, hundreds of AI researchers have reported on the distortion and perception qualities of their algorithms, citing the Michaeli and Blau paper that described the trade-off.
Sometimes, the implications of the perception-distortion trade-off aren’t dire. Nvidia, for instance, found that high-definition screens weren’t nicely rendering some lower-definition visual content, so in February it released a tool that uses deep learning to upscale streaming video. In this case, Nvidia’s engineers chose perceptual quality over accuracy, accepting the fact that when the algorithm upscales video, it will make up some visual details that aren’t in the original video. “The model is hallucinating. It’s all a guess,” Catanzaro said. “Most of the time it’s fine for a super-resolution model to guess wrong, as long as it’s consistent.”
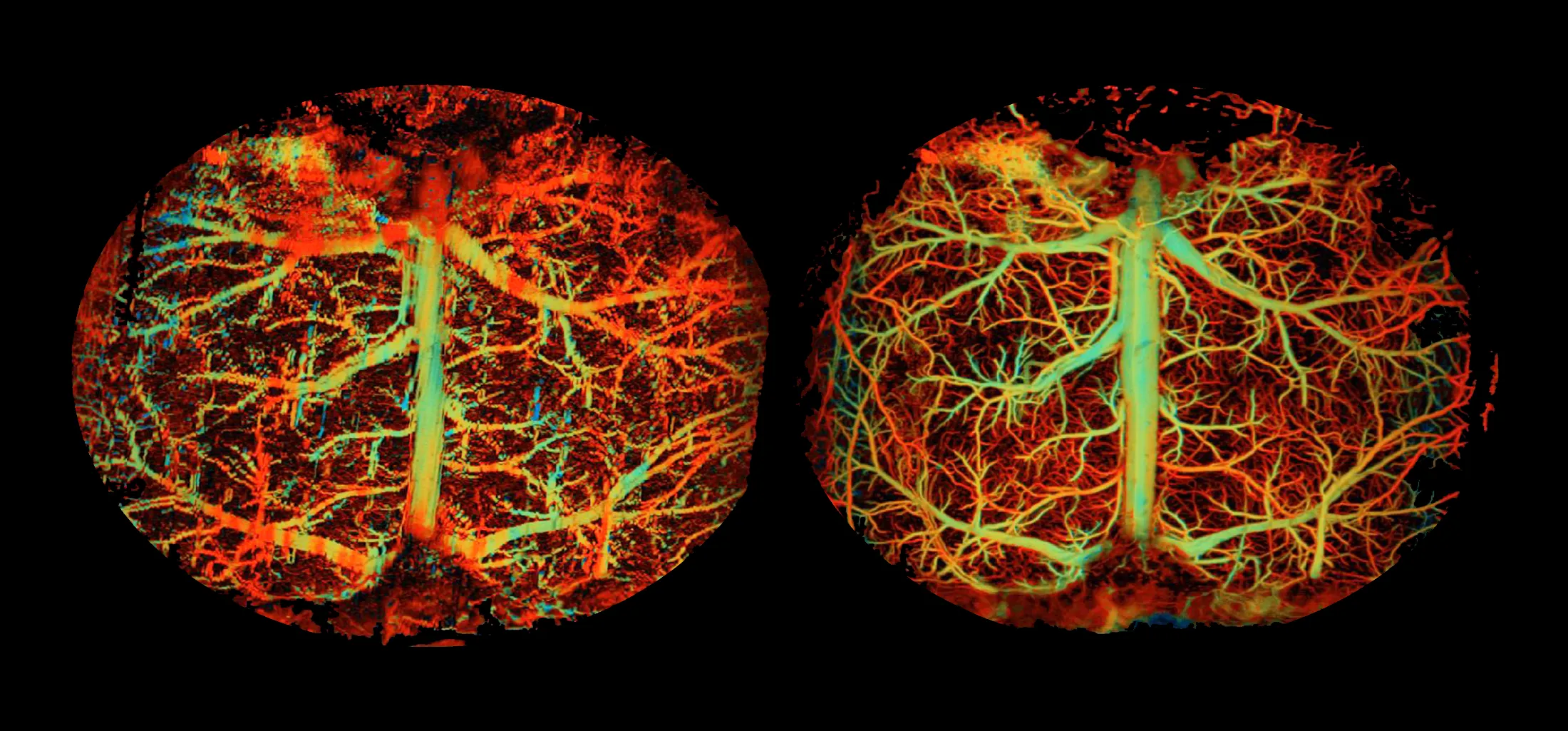
A view of the blood flow in a mouse’s brain (left) and the same view produced by using AI tools to improve the quality and accuracy of the image.
Junjie Yao and Xiaoyi Zhu/Duke University
Applications in research and medicine of course demand far more accuracy. AI technology has led to major advances in imaging, but it “sometimes comes with unwanted side effects, such as overfitting or [adding] fake features, and thus needs to be treated with extreme care,” said Junjie Yao, a biomedical engineer at Duke University. Last year, he co-wrote a paper describing how AI tools can improve existing methods of measuring blood flow and metabolism in the brain — while staying safely on the accurate side of the perception-distortion trade-off.
One way to circumvent limits on how much data can be extracted from an image is to simply incorporate data from more images — though that’s often not so simple. Researchers who study the environment through satellite imagery have made progress in combining different sources of visual data. In 2021, a group of researchers in China and the U.K. fused data from two different types of satellites to get a better view of deforestation in the Congo Basin, the second-largest tropical rainforest in the world and one of the biggest stores of biodiversity. The researchers took data from two Landsat satellites, which have measured deforestation for decades, and used deep learning techniques to refine the resolution of the images from 30 meters to 10 meters. They then fused that image set with data from two Sentinel-2 satellites, which have a slightly different array of detectors. The combined imagery “allowed 11% to 21% more disturbed areas to be detected than was possible using the Sentinel-2 or Landsat-7/8 images alone,” they wrote.
Michaeli suggests another way to get around, if not through, hard limits on the accessibility of information. Instead of settling on one firm answer for how to enhance a low-quality image, models could show multiple different interpretations of the original image. In a paper titled “Explorable Super Resolution,” he helped demonstrate how image-enhancement tools could present a user with multiple suggestions. One fuzzy, low-resolution image of a person wearing what appears to be a grayish shirt could be reconstructed into a higher-resolution image in which the shirt has black and white vertical stripes, horizontal stripes, or checks, all of which are equally plausible.
In another example, Michaeli took a low-quality photo of a license plate and ran it through a leading AI image enhancer, which showed that a 1 on the license plate looked most like a zero. But when the image was processed by a different, more open-ended algorithm that Michaeli designed, the digit looked equally likely to be a zero, 1 or 8. This approach could help rule out other numerals without erroneously concluding that the digit was zero.
As different disciplines grapple with the perception-distortion trade-off in their own ways, the question of how much we can extract from AI imagery and how much we can trust those images remains central. “We should keep in mind that to output these nice images, the algorithms just make up details,” Michaeli said. We can mitigate those hallucinations, but the all-powerful, crime-solving “enhance” button will remain a dream.