What Is Machine Learning?
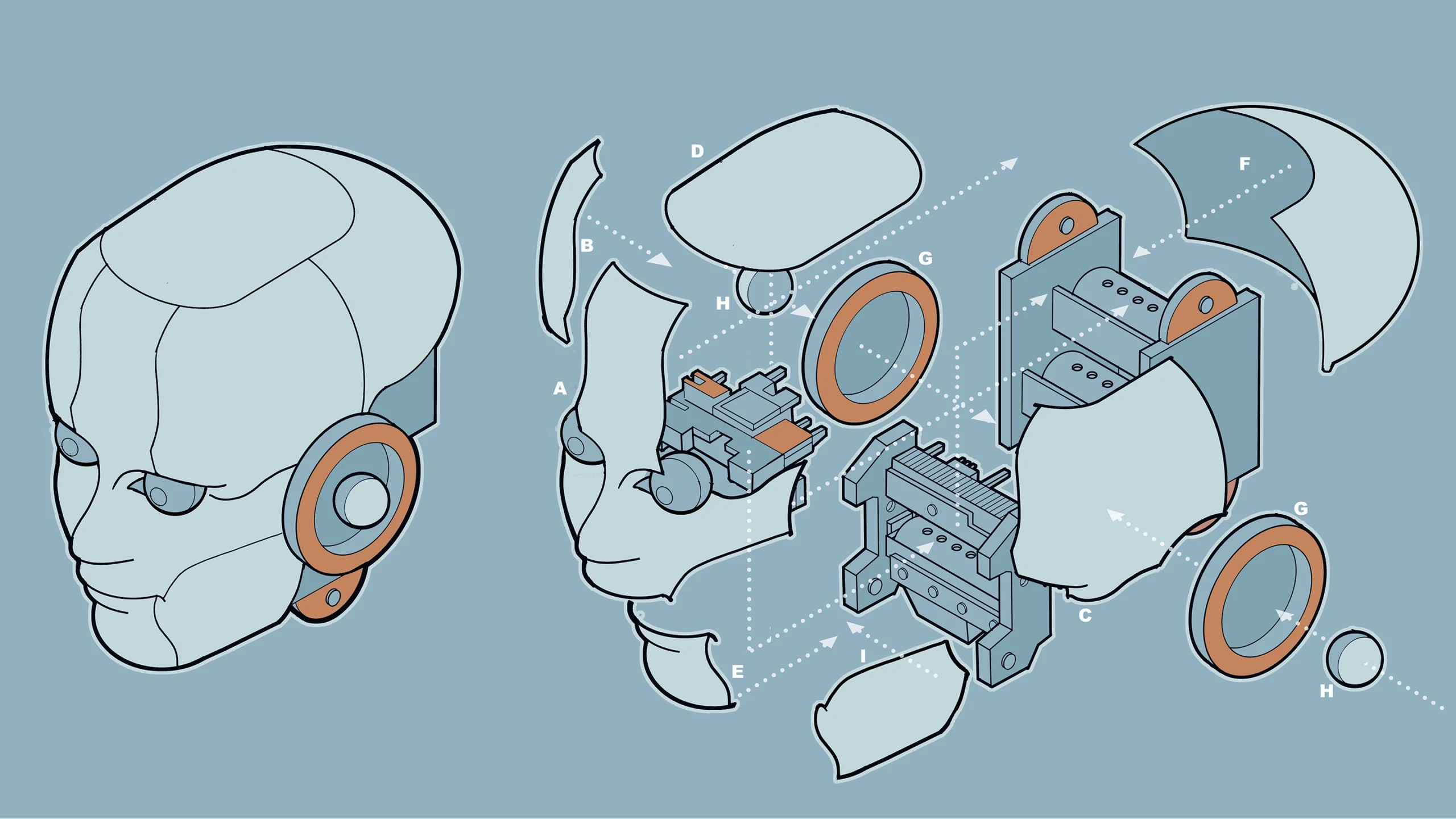
Son of Alan for Quanta Magazine
Introduction
By now, many people think they know what machine learning is: You “feed” computers a bunch of “training data” so that they “learn” to do things without our having to specify exactly how. But computers aren’t dogs, data isn’t kibble, and that previous sentence has way too many air quotes. What does that stuff really mean?
Machine learning is a subfield of artificial intelligence, which explores how to computationally simulate (or surpass) humanlike intelligence. While some AI techniques (such as expert systems) use other approaches, machine learning drives most of the field’s current progress by focusing on one thing: using algorithms to automatically improve the performance of other algorithms.
Here’s how that can work in practice, for a common kind of machine learning called supervised learning. The process begins with a task — say, “recognize cats in photos.” The goal is to find a mathematical function that can accomplish the task. This function, which is called the model, will take one kind of numbers as input — in this case, digitized photographs — and transform them into more numbers as output, which might represent labels saying “cat” or “not cat.” The model has a basic mathematical form, or shape, that provides some structure for the task, but it’s not likely to produce accurate results at first.
Now it’s time to train the model, which is where another kind of algorithm takes over. First, a different mathematical function (called the objective) computes a number representing the current “distance” between the model’s outputs and the desired result. Then, the training algorithm uses the objective’s distance measurement to adjust the shape of the original model. It doesn’t have to “know” anything about what the model represents; it simply nudges parts of the model (called the parameters) in certain mathematical directions that minimize that distance between actual and desired output.
Once these adjustments are made, the process restarts. The updated model transforms inputs from the training examples into (slightly better) outputs, then the objective function indicates yet another (slightly better) adjustment to the model. And so on, back and forth, back and forth. After enough iterations, the trained model should be able to produce accurate outputs for most of its training examples. And here’s the real trick: It should also maintain that performance on new examples of the task, as long as they’re not too dissimilar from the training.
Using one function to repeatedly nudge another function may sound more like busywork than “machine learning.” But that’s the whole point. Setting this mindless process in motion lets a mathematical approximation of the task emerge automatically, without human beings having to specify which details matter. With efficient algorithms, well-chosen functions and enough examples, machine learning can create powerful computational models that do things we have no idea how to program.
Classification and prediction tasks — like identifying cats in photos or spam in emails — usually rely on supervised machine learning, which means the training data is already sorted in advance: The photos containing cats, for example, are labeled “cat.” The training process shapes a function that can map as much of the input onto its corresponding (known) output as possible. After that, the trained model labels unfamiliar examples.
Unsupervised learning, meanwhile, finds structure within unlabeled examples, clustering them into groups that are not specified in advance. Content-recommendation systems that learn from a user’s past behavior, as well as some object-recognition tasks in computer vision, can rely on unsupervised learning. Some tasks, like the language modeling performed by systems like GPT-4, use clever combinations of supervised and unsupervised techniques known as self- and semi-supervised learning.
Finally, reinforcement learning shapes a function by using a reward signal instead of examples of desired results. By maximizing this reward through trial and error, a model can improve its performance on dynamic, sequential tasks like playing games (like chess and Go) or controlling the behavior of real and virtual agents (like self-driving cars or chatbots).
To put these approaches into practice, researchers use a variety of exotic-sounding algorithms, from kernel machines to Q-learning. But since the 2010s, artificial neural networks have taken center stage. These algorithms — so named because their basic shape is inspired by the connections between brain cells — have succeeded at many complex tasks once considered impractical. Large language models, which use machine learning to predict the next word (or word fragment) in a string of text, are built with “deep” neural networks with billions or even trillions of parameters.
But even these behemoths, like all machine learning models, are just functions at heart — mathematical shapes. In the right context, they can be extremely powerful tools, but they also have familiar weaknesses. An “overfitted” model fits its training examples so snugly that it can’t reliably generalize, like a cat-recognizing system that fails when a photo is turned upside-down. Biases in data can be amplified by the training process, leading to distorted — or even unjust — results. And even when a model does work, it’s not always clear why. (Deep learning algorithms are particularly plagued by this “interpretability” problem.)
Still, the process itself is easy to recognize. Deep down, these machines all learn the same way: back and forth, back and forth.