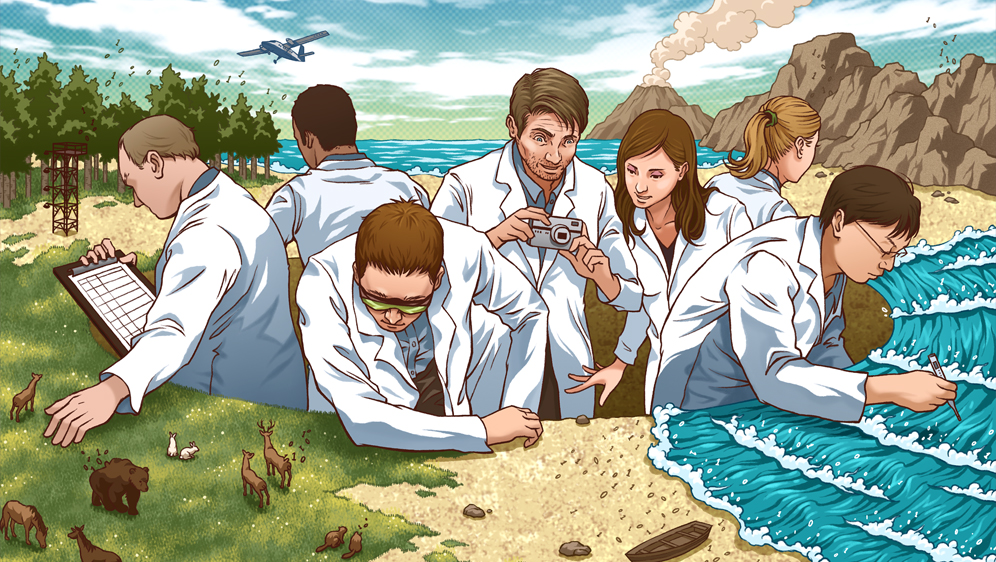
Yuta Onoda for Quanta Magazine
Introduction
Seven years ago, when David Schimel was asked to design an ambitious data project called the National Ecological Observatory Network, it was little more than a National Science Foundation grant. There was no formal organization, no employees, no detailed science plan. Emboldened by advances in remote sensing, data storage and computing power, NEON sought answers to the biggest question in ecology: How do global climate change, land use and biodiversity influence natural and managed ecosystems and the biosphere as a whole?
“We don’t understand that very well,” Schimel said.
Splitting his time at first between the new project and his role as a senior scientist at the National Center for Atmospheric Research, Schimel said he was surprised by the magnitude of the challenge, by the “sheer number of different measurements required to address the key science questions.” Before any observatories could be erected or staff members hired, decisions had to be made about where to take measurements, what to measure, how to measure it and how to generate meaningful data.
Schimel began to explore site options across the country and to assemble NASA-inspired “tiger teams” that could develop rigorous scientific methodologies and data-processing requirements. The final plan called for hiring dozens of scientists with disparate backgrounds; building more than 100 data-collection sites across the continental United States, Alaska, Hawaii and Puerto Rico; recording approximately 600 billion raw measurements per year for 30 years; and converting the raw data into more user-friendly “data products” to be made freely available to scientists and the public. Building the observatory network is projected to take four more years and cost $434 million, and millions more will be needed to cover annual operating expenses.
In 2007, Schimel became NEON’s chief scientist and first full-time employee. “I’ve been interested in processes at the continental scale for a long time and it’s always been a data-starved activity,” he said. “The opportunity to actually design a system to collect the right data at that scale was irresistible.”
Across the sciences, similar analyses of large-scale observational or experimental data, dubbed “big science,” offer insights into many of the greatest mysteries. What is dark matter, and how is it distributed throughout the universe? Does life exist, or is it capable of existing, on another planet? What are the connections between genetic markers and disease? How will the Earth’s climate change over the next century and beyond? How do neural networks form thoughts, memories and consciousness?
Much of the recent data frenzy — from the physical and life sciences to the user-generated content aggregated by Google, Facebook and Twitter — has come in the form of largely unstructured streams of digital potpourri that require new, flexible databases, massive computing power and sophisticated algorithms to wring out bits of meaning from them, said Matt LeMay, a former product manager at the URL shortening and bookmarking service Bitly.
But “big data is not magic,” he cautioned while teaching a database workshop this summer in Lower Manhattan. It doesn’t matter how much data you have if you can’t make sense of it.
For projects like NEON, interpreting the data is a complicated business. Early on, the team realized that its data, while mid-size compared with the largest physics and biology projects, would be big in complexity. “NEON’s contribution to big data is not in its volume,” said Steve Berukoff, the project’s assistant director for data products. “It’s in the heterogeneity and spatial and temporal distribution of data.”
Big Plans for Big Ecology
The National Ecological Observatory Network plans to begin collecting ecological data across the United States (including Alaska, Hawaii and Puerto Rico) by 2017.
Data Collection Sites: 106.
Data: 600 billion raw measurements per year.
Project Duration: Approximately 30 years.
Scientists: 66.
Estimated Construction Cost: $434 million.
Unlike the roughly 20 critical measurements in climate science or the vast but relatively structured data in particle physics, NEON will have more than 500 quantities to keep track of, from temperature, soil and water measurements to insect, bird, mammal and microbial samples to remote sensing and aerial imaging. Much of the data is highly unstructured and difficult to parse — for example, taxonomic names and behavioral observations, which are sometimes subject to debate and revision.
And, as daunting as the looming data crush appears from a technical perspective, some of the greatest challenges are wholly nontechnical. Many researchers say the big science projects and analytical tools of the future can succeed only with the right mix of science, statistics, computer science, pure mathematics and deft leadership. In the big data age of distributed computing — in which enormously complex tasks are divided across a network of computers — the question remains: How should distributed science be conducted across a network of researchers?
“Machines are not going to organize data science research,” said Bin Yu, a statistician at the University of California, Berkeley, who works on high-dimensional data problems. “Humans have to lead the way.” But, she said, “no one knows who is leading data science right now.”
Describing universities as “very siloed,” Yu said the goal is not merely interdisciplinary research, but rather to reach a state of “transdisciplinary research,” without walls or divisions.
Big science projects “can’t be dealt with by one person,” said Jack Gilbert, an environmental microbiologist at Argonne National Laboratory who has helped NEON develop standards for analyzing soil samples and plans to utilize its data when it comes online. “We need to work together. It’s too big a problem.”
Big ‘Bad’ Science
Ecology has traditionally involved small, localized studies that examine how organisms interact with their surroundings. But in grappling with the foundational questions on a regional or global scale, the microsystems approach brings to mind the old Indian parable in which six blind men feel different parts of an elephant to determine its shape. In John Godfrey Saxe’s popular retelling, the men come to wildly divergent conclusions, that the elephant is like a wall, spear, snake, tree, fan or rope.
“We were missing key pieces of information and not getting the big picture,” said Andrea Thorpe, 37, a plant ecologist who pursued smaller-scale studies on invasive species before joining NEON last year as its assistant director for terrestrial ecology.
Although smaller studies provide much-needed depth and detail at a local level, they also tend to be limited to a specific set of questions and reflect an investigator’s particular methodology, which can make results more difficult to reproduce or reconcile with broader models.
“You can’t escape the fact that there are some really big impacts happening to the ecosystem that can’t be studied with short-term, smaller studies,” Thorpe said.
Macrosystems, or “big,” ecology, as Schimel calls it, becomes possible with standardized, broad-scale data. He says that having large, rich data sets enables scientists to incorporate the complexity and variability of the real world into their models of large-scale phenomena, rather than to “peanut butter over” them with simplified models.
Ecologists first delved into the world of big data about 50 years ago with the International Biological Program, which cut across scientific disciplines and involved dozens of countries in an attempt to model large-scale systems. It is beloved by the pioneers and supporters of international partnerships but was roundly criticized at the time by traditional biologists who were skeptical of big data modeling and titanic collaborations. Although the project paved the way for newer collaborative efforts like NEON, some of the criticisms have lingered.
In 1969, Thomas Rosswall joined the Swedish tundra biome section of the IBP as a 28-year-old microbial ecologist. At a time when little coordinated research existed in biology, he said, the challenge was to get the microbiologists to work with the botanists and the hydrologists to work with the meteorologists. And the Cold War meant that outside scientists couldn’t visit the Russian sites. Instead, the Russians shared pictures of their work.
Rosswall, a former executive director of the International Council for Science who is now retired, said his IBP work shaped his career as an international scientist. The tundra project was a particularly close-knit community, he said. “We were also young and rather naïve, and maybe that was good,” he said. “We didn’t have preconceived ideas on how things should be done.”
The idealistic vision was met with sharp criticism. Some biologists thought that money was being wasted on big new ecosystem science projects that didn’t yet have a solid theoretical foundation. In part, Rosswall said, the critics thought he and his colleagues “were too young and got too much money.”
“This was far more money than had been spent on ecological research,” said Paul Risser, a plant ecologist and research cabinet chair at the University of Oklahoma who worked on the IBP effort to study grassland ecosystems. “People were used to getting $50,000 to $60,000 grants, and here was millions of dollars going to IBP.”
Critics also said the large-scale, data-driven models wouldn’t work. And many didn’t. But those failures helped shape future projects, showing scientists the need to build larger databases and to incorporate metadata — data about the handwritten data that filled notebooks during the IBP — into their projects.
The IBP also lacked modern remote-sensing technologies, not to mention today’s computing power, databases, digital storage, telecommunications and Internet. “IBP worked on big data before we really had the tools,” Risser said.
And some traditional, free-spirited ecologists chafed at the idea of joining a structured program that wouldn’t allow them to choose their own research topics or use their own methodologies. “The research was very orchestrated, and most ecologists weren’t used to working in regimented environments,” Risser said. However, Risser pointed out that the project “spawned a whole generation of graduate students who were used to working across disciplines and with mathematical modeling.”
Despite the IBP’s shortcomings, some of its data sets and models are still in use today. And its legacy lives on in the open collaborations and methodologies of today’s big ecology projects, including NEON, the Long Term Ecological Research Network, which has been running since 1980, and the Data Observation Network for Earth, which provides a platform for the sharing and archiving of global ecological data.
And after 50 years, the criticisms have softened. “It’s part of the process,” Rosswall said. He is excited to see increased collaboration between Arctic research stations, many of which originated with the IBP. “We really shaped the basis for the development of how you could and should do field research,” he said.
Now Rosswall is busy helping to develop a plan for a new big ecology project: a Swedish version of NEON.
Come Together
Schimel’s philosophy for NEON was partly shaped 30 years ago by his experience as a research assistant with a team that originated with IBP’s grassland program. His career was just beginning, and already he was sharing lab space and resources with chemists, plant scientists and microbiologists. “For me, the shock was that everywhere did not work that way,” he said. “The IBP was ahead of its time — in its attitude towards data and models as products, towards teamwork and leadership, as opposed to individual insight as the way to do science.”
Of the 66 researchers on NEON’s staff, there are “no two people who do the same thing,” said Berukoff, 36. With a background in computing, software engineering, engineering, astrophysics and “stitching together data from different disciplines,” he felt the project “was sort of a natural fit.”
But working on a diverse team means researchers must be willing to listen and learn. “People often think they’re talking about the same thing when they’re not,” Berukoff said. “Or they’re talking about the same thing and they’re talking about it in two different ways.”
While these differences present opportunities to learn about other fields, they “can also be frustrating because of this impedance mismatch between what is being said and heard,” he said. “Bridging that gap is central to the success of a project.”
The Earth Microbiome Project, an international effort to map and study microbe samples collected around the globe, works with hundreds of principal investigators. “Occasionally, we come across people who don’t want to share the data or wonder what’s in it for them,” said Gilbert, 36, who has been with the project since 2010. “We tend to attract people who are like-minded. People who are not like-minded tend to stay clear.”
Many of the like-minded are younger researchers, who also tend to be “the ones with the skills to do this,” said Gilbert. “The majority of the scientific community is completely overwhelmed by data,” he said. “We need to adapt in order to keep ahead of the tidal wave.”
Part of the adjustment involves embracing “open science” practices, including open-source platforms and data analysis tools, data sharing and open access to scientific publications, said Chris Mattmann, 32, who helped develop a precursor to Hadoop, a popular open-source data analysis framework that is used by tech giants like Yahoo, Amazon and Apple and that NEON is exploring. Without developing shared tools to analyze big, messy data sets, Mattmann said, each new project or lab will squander precious time and resources reinventing the same tools. Likewise, sharing data and published results will obviate redundant research.
To this end, international representatives from the newly formed Research Data Alliance met this month in Washington to map out their plans for a global open data infrastructure.
Younger scientists have grown accustomed to producing and using open data and open-source tools and “are putting pressure on the ‘establishment’ to move rapidly to open publication,” said Schimel, 58. “Many are involved in questions that can’t plausibly be answered with the resources a single PI can control.”
In a professional survey conducted by NEON, “80 percent of the respondents who had their degrees less than 20 years were likely or very likely to use NEON’s open data,” Schimel said. “The oldest group was far less likely and less supportive. Accordingly, NEON’s outreach strategy has focused far less on engaging senior researchers and far more towards informing and involving the ‘uns’ (undergraduates to untenured).”
Yu, the Berkeley statistician, hopes that mathematicians and statisticians will become intellectual leaders in big science projects. But “mathematics is more focused on technical work and doesn’t encourage people to develop leadership skills,” she said. “If we don’t change our culture, that could happen, where they need you, but you won’t be there making important decisions.”
Engineers are used to working on teams focused on solving problems, said Yu, 50, but “mathematics tends to rank people linearly” to determine an individual pecking order. “The culture has to change to encourage and nurture young people to have a rewarding career. It’s up to the older people to do that.”
Yu advises math students to learn more computing skills. Her students have access to the supercomputer at Lawrence Berkeley National Laboratory, but some of them “don’t have the skills yet to use it,” she said. “They’re learning.”
After NEON entered its construction phase last year, Schimel, whose interests lie in research and science planning rather than construction and implementation, left to pursue his next big project. He became the lead scientist for carbon and climate at NASA’s Jet Propulsion Laboratory in Pasadena, Calif., where he is trying to use space-based observations to study carbon budgets and ecosystems globally.
“Agile scientists like Schimel are important to these projects,” Mattmann said. “He realizes that an emerging class of data scientists is really what’s needed.”
Mattmann, a senior computer scientist who works with Schimel at the Jet Propulsion Laboratory, described a wall that often exists between data management people and scientists. “If you have a CS degree, you’re classified as an IT person,” he said. “But in CS, often you’ll have studied the same math — you just apply it to different models.
“I feel I’m not an IT guy,” Mattmann said. “The big question is whether we should take trained computer scientists and teach them the hands-on bench science or whether we should take those physical and natural scientists and teach them CS.” A few years ago, he mostly hired computer scientists, but is now bringing in scientists and teaching them how to program.
Transforming scientists, mathematicians and computer scientists into hybrid data scientists is going to increase interest in math, engineering and technology in education, said Mattmann. “It’s all we have to compete with the Facebooks of the world. You can get paid a lot at Facebook to figure out who poked who, or you can use data science to understand water budgets to create a sustainable planet.”
The academic promotion system also “has to change to value cross-disciplinary research,” Yu said. “It’s hard to evaluate people on the boundaries, but that’s the most exciting part of science right now.”
This article was reprinted on Wired.com.