A Map of Human History, Hidden in DNA
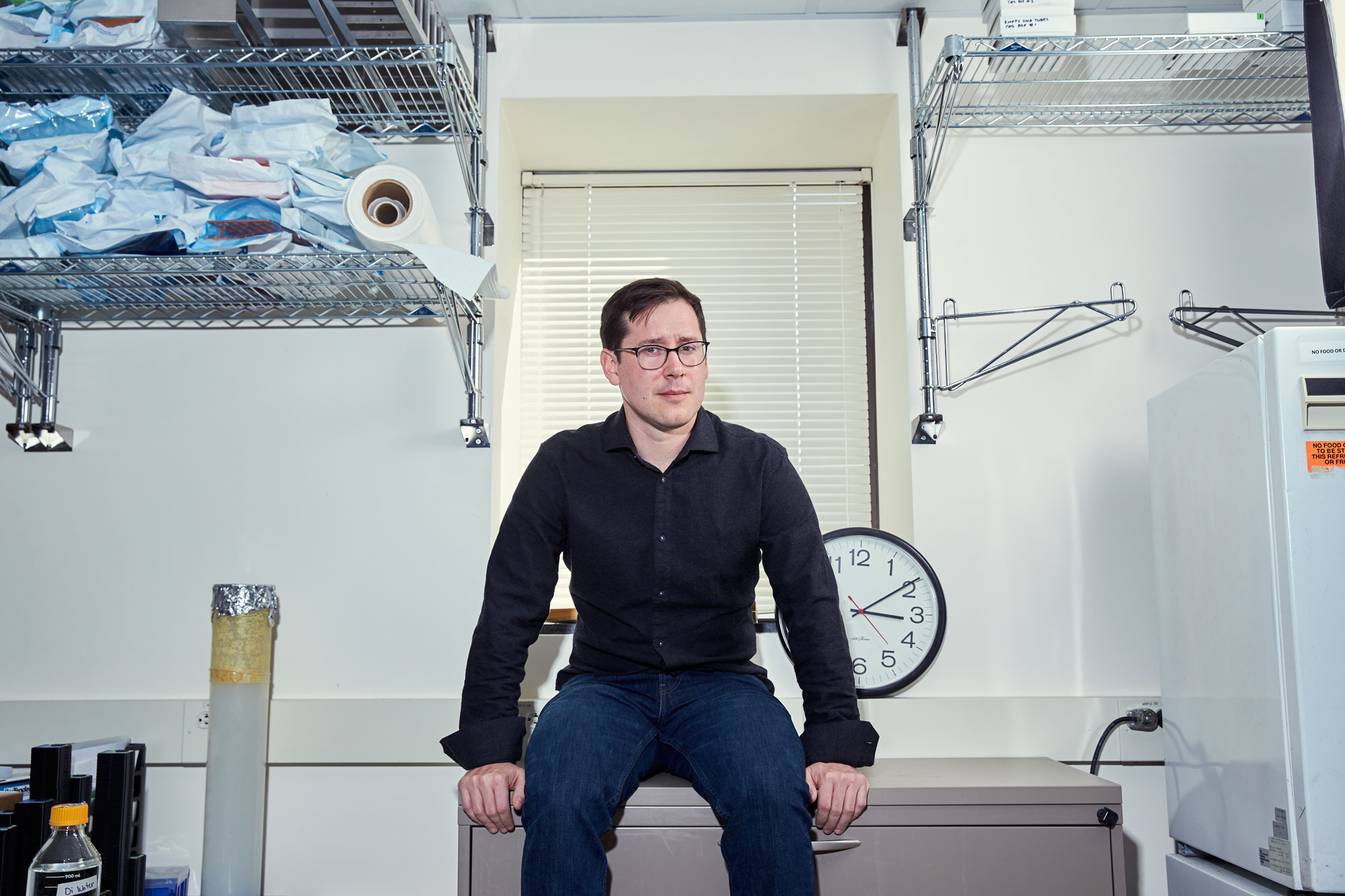
Saverio Truglia for Quanta Magazine
Introduction
Ask John Novembre to recall a fun time, and he might tell you about a recent weeklong hackathon. He and his students and postdocs set aside their daily obligations to stay up late eating takeout and crunching data.
This isn’t to say that Novembre, a computational biologist, is purely a computer geek (although he’ll admit that’s part of his identity). Yes, his whiteboard-lined walls at the University of Chicago are adorned with symbols and graphs and equations — embryos of the clever algorithms and computational tricks that allow him to wrest meaning from some of the largest genomic data sets in the world. But that’s just a glimpse of what he does.
Born into a military family, Novembre grew up moving from place to place, spending three years in Uruguay, where his mother is from. His early exposure to human differences — “I loved geography, I loved languages, I loved history” — morphed into a deep curiosity about evolution and genetic diversity. His work reflects a broad ambition: to understand how populations vary in time and space. He studies how humanity’s genetic code shifts and mixes as groups expand, shrink, migrate, mingle, evolve and die out.
His mathematical chops have served him well in this endeavor. His innovative analyses and new ways of visualizing complex data reveal the genetic signatures of ancestry and the surprising connections between genes and geography. In 2015, at the age of 37, Novembre won a MacArthur fellowship — the so-called “genius grant” — which recognizes “talented individuals who have shown extraordinary originality” and “promise for important future advances based on a track record of significant accomplishment.”
Yet for all his accolades, Novembre comes across as genuinely humble, quoting colleagues’ papers the way English majors quote Dickinson and Yeats. Hanging above his desk, within eyeshot of his dry-erase brainstorms, is an old map he thinks is from the 1600s — a constant reminder that any human attempt to model the world will always be a “very imperfect representation.”
Quanta Magazine spoke with Novembre about the motivations behind his work, the challenges of using DNA to interpret the past, and the racial legacy of genetics research. An edited and condensed version of the conversation follows.
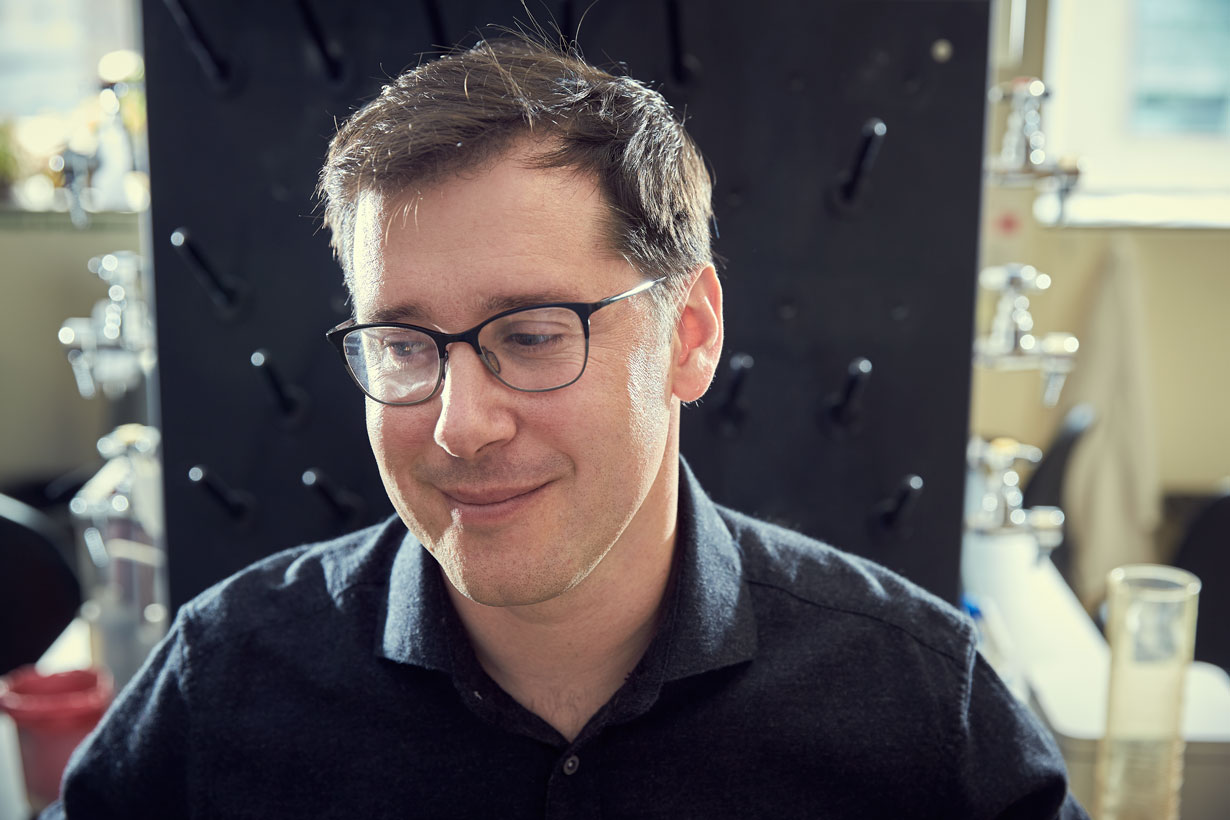
Saverio Truglia for Quanta Magazine
QUANTA MAGAZINE: What got you thinking about genetic diversity as a computational problem?
JOHN NOVEMBRE: For me, the path starts pretty far back. In high school, I was a bit of a computer programming nerd. But in my classes, I was learning about the genetic code, which was completely mesmerizing. Then in college, I got a chance to do a summer research internship at Stanford, where I heard a talk by a student who had interned in Luigi Luca Cavalli-Sforza’s lab. What they do — what they’ve become famous for — is to look at variations in human genes, how they’re distributed across the globe, and what they can tell us about human history. That was fascinating to me.
I went back to my home campus, and I found a lab working on the population genetics of Quercus gambelii, the Gambel oak. I learned just how difficult a lot of the analysis tools were to use, and how much math and computation is involved in analyzing genetic data. All of a sudden I realized, “Wait a minute. Here’s this thing I really love — programming — so why don’t I combine these two passions?” My day-to-day activity became tinkering with computers, but my larger end is something that intellectually fascinates me, which is understanding genetic variation and how it changes through time.
Early in your career, you made waves by uncovering deficiencies in a common statistical tool known as principal component analysis (PCA). How did this discovery further your work in genetics?
What PCA does is, it takes an individual’s genetic data and boils it down to just a few numbers. In learning about how this method works — its strengths and its weaknesses — I understood that the patterns it produces could reflect spatial structure in population data.
I was hoping to get access to genetic data from a region of the world where there’s dense sampling, so that I could see what variation looks like at a continuous scale, where populations kind of blend into one another. And it turned out I was very lucky in that I got invited to join a collaboration with Carlos Bustamante, [then] at Cornell, to analyze one of the largest collections of [genomic data] being applied to human populations. The full data set was 3,192 European individuals. A large fraction of the sample had answered an ancestry questionnaire to say where their grandparents came from, and based on that, we saw we had samples from roughly 37 different origins across Europe.
So what did you learn?
When we applied PCA, right away we saw this major pattern: There was a striking resemblance between where individuals are located in genetic space and their geography — where their grandparents came from. That’s really remarkable given how closely related human individuals are. Most geneticists wouldn’t have thought you could tease apart very fine-scale structure within continental scales.
How fine-scale are we talking about?
Let’s say I took an individual and hid their geographic location and then tried to put them back on a map. How well could I do? When we did this, we could often get within a few hundred kilometers. Even when we looked at German-speaking Swiss versus French-speaking Swiss versus Italian-speaking Swiss, we could see shifts in the genetic distribution.
I’m surprised that my grandparents’ geographic coordinates could have such a notable effect on my genetics, given how often humans migrate. How do you explain this influence?
This is something I want to stress: The effect on your genetics is actually incredibly small. It’s just that we’re looking at so many locations in the genome that we can pick up very small effects. This is the magic of big data: Very subtle patterns become detectable. So it’s not that where your grandparents live has a huge impact on your genetics. It’s actually a very, very minor effect. But when you have hundreds of thousands of measurements, you can start to pick out that an individual seems to come from one location versus another.
What are your thoughts on the ethics of commercial ancestry tests?
I advise for Ancestry.com — their DNA branch — so I’m very sensitive to the challenges of communicating results. On the one hand, projects like our genetic map of Europe show the tremendous potential and power of these tools for learning about ancestry. But then there’s also the immense complexity of it: What does it really mean to talk about where an individual is from? We can talk about where our parents and our grandparents are from, or we can go very far back into the past when we all came from Africa. And we can have different ideas about origin, in terms of geographic location versus some kind of cultural or ethnic population.
I’d say we’re still in the early days of really nailing this problem of using genetic data from today to interpret the past. We’re still facing the complexity of real biological systems and populations, which resist some of our attempts to use very simple models of history.
In what ways has your work influenced how you think about race?
It’s very clear that genetics research has a difficult and dark history. But it’s been exciting to be part of a new generation doing this kind of work in a time when diversity is much more appreciated and understood and valued — and when we have the data to make it even more clear just how poorly conceived racial worldviews have been.
Are you thinking of a particular example?
A very powerful one for me was being part of some of the first teams to look at genome-wide data taken from multiple human populations. You can sort the genome by what regions vary the most across human populations and then ask, “OK, what genes are near those locations, and what do we know about them?”
If you do this exercise, you will see, at the very extreme top of the list, variants that are involved in skin pigmentation, in eye color, in hair color. So it’s an empirical fact that the things we use to see differences in each other are outliers in the human genome. Your average set of genes in the human genome is much more similar globally.
You analyzed the first whole-genome sequences of three gray wolf species and compared them to the genomes of three dog species. What did you discover?
That was a big surprise. We were thinking we might find that all three of the dog lineages are most closely related to one of the three wolf lineages. They might all be related to the Israeli wolf, for instance, because maybe dogs were domesticated in the Middle East. Or maybe there were two domestications of dogs, and the dingo would be related to the Chinese wolf while the basenji was related to the Croatian wolf.
But what we saw was that the three dogs were most closely related to each other but not embedded within the genealogy of the three wolves. Our hypothesis is that there was a wolf lineage that dogs were domesticated from that has since gone extinct. The story’s gotten incredibly complicated, and I think the final chapter’s not written yet.
Are you a dog person?
Not particularly, no. I would say my motivation was primarily to try to solve this larger challenge for the whole field, which is: How do we use DNA sequences today as a record of the past? You can swap out the species names for me, and it’s still interesting. It’s still a fun problem.
How has your approach to analyzing genetic data evolved over time?
There’s been increasing movement in my work toward data visualization. Your eye can actually process a large amount of information and interpret complex patterns. With the right visualization tools, you gain a more direct and intuitive understanding of the major features of the data and how they reflect biological processes.
Can you give an example?
One of the tools we’ve developed is a method that tells us where in a landscape there is more or less gene flow — in other words, how individuals are moving between populations. Our analysis infers areas where there’s more genetic difference than you’d expect per unit of geographic distance, and other areas where there’s less. So we’re able to produce a geographic map that is colored in brown and blue to represent areas of low and high migration.
For example, we looked at genetic data from more than 1,000 elephants sampled across Africa. With our approach, you feed in the data with no prior knowledge, and you get this map of migration rates. You see this brown barrier down Central Africa marking where there’s low migration and a blue corridor in the east where there’s high migration. Of course, if you know the ecology, you understand: “Oh! That’s the forest elephants on one side and the savannah elephants on the other.”
Have you applied this method to other populations?
Yes. When we run it on the human data from Europe, for instance, we see it infers a brown area of reduced migration between the U.K. and France, representing essentially the English Channel. We see a lot of blue — high migration — in the North Sea because of historical connections there, such as Viking contacts between Scandinavia and the U.K. Then we see brown diffusely around Switzerland and Austria, which we think represents the Alps.
Did you get any puzzling results, such as areas of low or high migration that don’t seem to jibe with the landscape?
I’m more surprised by how often the genetics do align with the geological features. You take a bunch of living individuals and extract a molecule from their bodies and start comparing them to one another, and you can see that the Alps are a feature of our planet. It’s kind of wild.
How have the questions you’re researching changed from when you started out?
The data types have changed, and the scale of the data has changed. For my Ph.D., I worked on trying to learn what I could from a single genetic variant that was observed in 71 populations. Now it’s completely routine to have data sets with millions of variants. I couldn’t have imagined that we’d be where we are today back then. So that’s an incredible game-changer, but the core question is still the same: How do we use mathematics and statistical models to interpret population genetic data?
What are the big remaining problems you hope to solve?
I think one holy grail is a method that would allow you to infer how migration rates and population sizes change through time and space. It would be a very complete description of a population and its demographic history.