Deep Learning Poised to ‘Blow Up’ Famed Fluid Equations
Introduction
For more than 250 years, mathematicians have been trying to “blow up” some of the most important equations in physics: those that describe how fluids flow. If they succeed, then they will have discovered a scenario in which those equations break down — a vortex that spins infinitely fast, perhaps, or a current that abruptly stops and starts, or a particle that whips past its neighbors infinitely quickly. Beyond that point of blowup — the “singularity” — the equations will no longer have solutions. They will fail to describe even an idealized version of the world we live in, and mathematicians will have reason to wonder just how universally dependable they are as models of fluid behavior.
But singularities can be as slippery as the fluids they’re meant to describe. To find one, mathematicians often take the equations that govern fluid flow, feed them into a computer, and run digital simulations. They start with a set of initial conditions, then watch until the value of some quantity — velocity, say, or vorticity (a measure of rotation) — begins to grow wildly, seemingly on track to blow up.
Yet computers can’t definitively spot a singularity, for the simple reason that they cannot work with infinite values. If a singularity exists, computer models might get close to the point where the equations blow up, but they can never see it directly. Indeed, apparent singularities have vanished when probed with more powerful computational methods.
Such approximations are still important, however. With one in hand, mathematicians can use a technique called a computer-assisted proof to show that a true singularity exists close by. They’ve already done it for a simplified, one-dimensional version of the problem.
Now, in a preprint posted online earlier this year, a team of mathematicians and geoscientists has uncovered an entirely new way to approximate singularities — one that harnesses a recently developed form of deep learning. Using this approach, they were able to peer at the singularity directly. They are also using it to search for singularities that have eluded traditional methods, in hopes of showing that the equations aren’t as infallible as they might seem.
The work has launched a race to blow up the fluid equations: on one side, the deep learning team; on the other, mathematicians who have been working with more established techniques for years. Regardless of who might win the race — if anyone is indeed able to reach the finish line — the result showcases how neural networks could help transform the search for new solutions to scores of different problems.
The Disappearing Blowup
The equations at the center of the new work were written down by Leonhard Euler in 1757 to describe the motion of an ideal, incompressible fluid — a fluid that has no viscosity, or internal friction, and that cannot be squeezed into a smaller volume. (Fluids that do have viscosity, like many of those found in nature, are modeled instead by the Navier-Stokes equations; blowing those up would earn a $1 million Millennium Prize from the Clay Mathematics Institute.) Given the velocity of each particle in the fluid at some starting point, the Euler equations should predict the flow of the fluid for all time.
But mathematicians want to know whether in some situations — even though nothing might seem amiss at first — the equations could eventually run into trouble. (There’s reason to suspect this might be the case: The ideal fluids they model don’t behave anything like real fluids that are just the slightest bit viscous. The formation of a singularity in the Euler equations could explain this divergence.)
In 2013, a pair of mathematicians proposed just such a scenario. Since the dynamics of a full three-dimensional fluid flow can get impossibly complicated, Thomas Hou, a mathematician at the California Institute of Technology, and Guo Luo, now at the Hang Seng University of Hong Kong, considered flows that obey a certain symmetry.
In their simulations, a fluid rotates inside a cylindrical cup. The fluid in the top half of the cup swirls clockwise, while the bottom half swirls counterclockwise. The opposing flows lead to the formation of other complicated currents that cycle up and down. Soon enough, at a point along the boundary where the opposing flows meet, the fluid’s vorticity explodes.
Merrill Sherman/Quanta Magazine
While this demonstration provided compelling evidence of a singularity, without a proof it was impossible to know for sure that it was one. Before Hou and Luo’s work, many simulations proposed potential singularities, but most of them disappeared when tested later on a more powerful computer. “You think there is one,” said Vladimir Sverak, a mathematician at the University of Minnesota. “Then you put it on a bigger computer with much better resolution, and somehow what seemed like a good singularity scenario just turns out to not really be the case.”
That’s because these solutions can be finicky. They’re vulnerable to small, seemingly trivial errors that can accumulate with each time step in a simulation. “It’s a subtle art to try to do a good simulation on a computer of the Euler equation,” said Charlie Fefferman, a mathematician at Princeton University. “The equation is so sensitive to tiny, tiny errors in the 38th decimal place of the solution.”
Still, Hou and Luo’s approximate solution for a singularity has held up against every test thrown at it so far, and it has inspired a great deal of related work, including full proofs of blowup for weaker versions of the problem. “It’s by far the best scenario for singularity formation,” Sverak said. “Many people, including myself, believe that this time it’s a real singularity.”
To fully prove blowup, mathematicians need to show that, given the approximate singularity, a true one exists nearby. They can rewrite that statement — that a real solution lives in a sufficiently close neighborhood of the approximation — in precise mathematical terms, and then show that it’s true if certain properties can be verified. Verifying those properties, however, requires a computer once again: this time, to perform a series of computations (which involve the approximate solution), and to carefully control the errors that might accumulate in the process.
Hou and his graduate student Jiajie Chen have been working toward a computer-assisted proof for several years now. They’ve refined the approximate solution from 2013 (in an intermediate result they have not yet made public), and are now using that approximation as the foundation for their new proof. They’ve also shown that this general strategy can work for problems that are easier to solve than the Euler equations.
Now another group has joined the hunt. They’ve found an approximation of their own — one that closely resembles Hou and Luo’s result — using a completely different approach. They’re currently using it to write their own computer-assisted proof. To obtain their approximation, though, they first needed to turn to a new form of deep learning.
Glacial Neural Networks
Tristan Buckmaster, a mathematician at Princeton who is currently a visiting scholar at the Institute for Advanced Study, encountered this new approach purely by chance. Last year, Charlie Cowen-Breen, an undergraduate in his department, asked him to sign off on a project. Cowen-Breen had been studying ice sheet dynamics in Antarctica under the supervision of the Princeton geophysicist Ching-Yao Lai. Using satellite imagery and other observations, they were trying to infer the viscosity of the ice and predict its future flow. But to do that, they relied on a deep learning approach that Buckmaster hadn’t seen before.
Unlike traditional neural networks, which get trained on lots of data in order to make predictions, a “physics-informed neural network,” or PINN, must satisfy a set of underlying physical constraints as well. These might include laws of motion, energy conservation, thermodynamics — whatever scientists might need to encode for the particular problem they’re trying to solve.
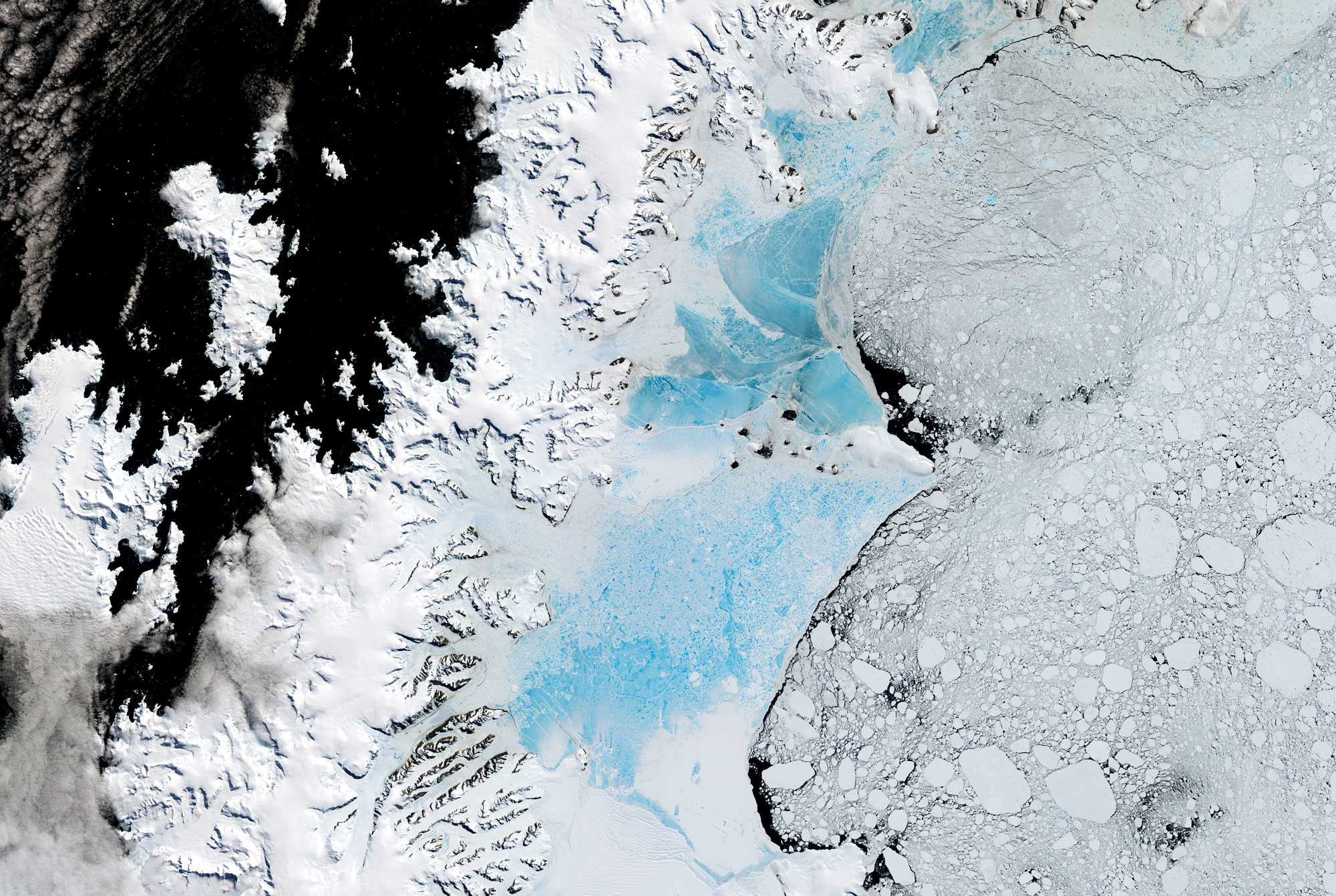
New work on the blowup of the Euler equations began in an unlikely place — with geophysicists studying ice sheet dynamics in Antarctica. Their research required a deep learning approach that later proved useful in more theoretical settings.
NASA’s Earth Observatory
Injecting physics into the neural network serves several purposes. For one, it allows the network to answer questions when very little data is available. It also enables the PINN to infer unknown parameters in the original equations. In a lot of physical problems, “we know roughly how the equations should look like, but we don’t know what the coefficients of [certain] terms should be,” said Yongji Wang, a postdoctoral researcher in Lai’s lab and one of the new paper’s co-authors. That was the case for the parameter that Lai and Cowen-Breen were trying to determine.
“We call it hidden fluid mechanics,” said George Karniadakis, an applied mathematician at Brown University who developed the first PINNs in 2017.
Cowen-Breen’s request got Buckmaster thinking. The classical methods for solving the Euler equations with a cylindrical boundary — as Hou, Luo and Chen had done — involved painstaking progressions through time. But because of that dependence on time, they could only get very close to the singularity without ever reaching it: As they crept closer and closer to something that might look like infinity, the computer’s calculations would get more and more unreliable, so that they couldn’t actually look at the point of blowup itself.
But the Euler equations can be represented with another set of equations that, through a technical trick, sweep time aside. Hou and Luo’s 2013 result wasn’t just notable for pinning down a very precise approximate solution; the solution they found also seemed to have a particular kind of “self-similar” structure. That meant that as the model evolved through time, its solution followed a certain pattern: Its shape at a later time looked a lot like its original shape, only bigger.
That feature meant that mathematicians could focus on a time before the singularity occurred. If they zoomed in on that snapshot at the right rate — as if they were looking at it under a microscope with an ever-adjusting magnification setting — they could model what would happen later, right up to the point of the singularity itself. Meanwhile, if they re-scaled things in this way, nothing would actually go terribly wrong in this new system, and they could eliminate any need to deal with infinite values. “It’s just approaching some nice limit,” Fefferman said, and that limit represents the occurrence of the blowup in the time-dependent version of the equations.
“It’s easier to model these [re-scaled] functions,” Sverak said. “And so, if you can describe a singularity by using a [self-similar] function, it’s a big advantage.”
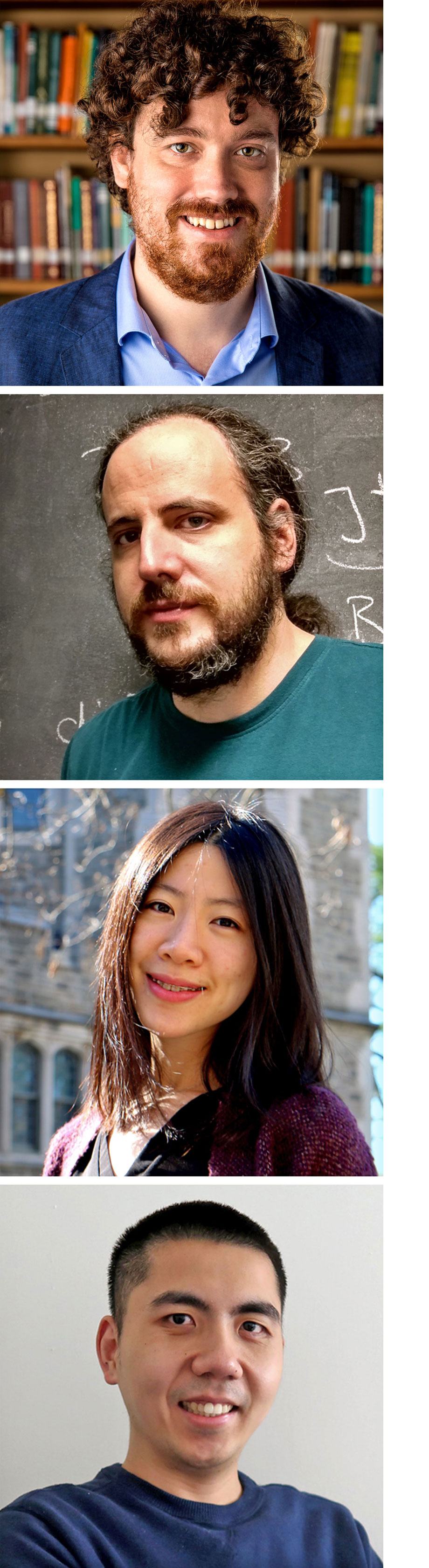
From top to bottom: The mathematicians Tristan Buckmaster and Javier Gómez-Serrano teamed up with the geophysicists Ching-Yao Lai and Yongji Wang to use physics-informed neural networks to study the blowup of the Euler equations.
(top to bottom): Dan Komoda, Institute for Advanced Study; Concepción Serrano; Matthew Edwards; Sang He
The problem is that for this to work, the mathematicians don’t just have to solve the equations (now written in self-similar coordinates) for the usual parameters, such as velocity and vorticity. The equations themselves also have an unknown parameter: the variable that governs the rate of magnification. Its value has to be just right to ensure that the solution to the equations corresponds to a blowup solution in the original version of the problem.
The mathematicians would have to solve the equations forward and backward simultaneously — a difficult if not impossible task to achieve using traditional methods.
But finding those kinds of solutions is exactly what PINNs were designed for.
The Road to Blowup
In retrospect, Buckmaster said, “it seems like an obvious thing to do.”
He, Lai, Wang and Javier Gómez-Serrano, a mathematician at Brown University and the University of Barcelona, established a set of physical constraints to help guide their PINN: conditions related to symmetry and other properties, as well as the equations they wanted to solve (they used a set of 2D equations, rewritten using self-similar coordinates, that are known to be equivalent to the 3D Euler equations at points approaching the cylindrical boundary).
They then trained the neural network to search for solutions — and for the self-similar parameter — that satisfied those constraints. “This method is very flexible,” Lai said. “You can always find a solution as long as you impose the correct constraints.” (In fact, the group showcased that flexibility by testing the method on other problems.)
The team’s answer looked a lot like the solution that Hou and Luo had arrived at in 2013. But the mathematicians hope that their approximation paints a more detailed picture of what’s happening, since it marks the first direct calculation of a self-similar solution for this problem. “The new result specifies more precisely how the singularity is formed,” Sverak said — how certain values will blow up, and how the equations will collapse.
“You’re really extracting the essence of the singularity,” Buckmaster said. “It was very difficult to show this without neural networks. It’s clear as night and day that it’s a much easier approach than traditional methods.”
Gómez-Serrano agrees. “This is going to be part of the standard toolboxes that people are going to have at hand in the future,” he said.
Once again, PINNs have revealed what Karniadakis called “hidden fluid mechanics” — only this time, they made headway on a far more theoretical problem than the ones PINNs are usually used for. “I haven’t seen anybody use PINNs for that,” Karniadakis said.
That’s not the only reason mathematicians are excited. PINNs might also be perfectly situated to find another type of singularity that’s all but invisible to traditional numerical methods. These “unstable” singularities might be the only ones that exist for certain models of fluid dynamics, including the Euler equations without a cylindrical boundary (which are already much more complicated to solve) and the Navier-Stokes equations. “Unstable things do exist. So why not find them?” said Peter Constantin, a mathematician at Princeton.
But even for the stable singularities that classical techniques can handle, the solution the PINN provided for the Euler equations with a cylindrical boundary “is quantitative and precise and has a much better chance of being made rigorous,” Fefferman said. “Now there’s a road map [toward a proof]. It will take a lot of work. It will take a lot of skill. I imagine it will take some originality. But I don’t see that it will take genius. I think it’s doable.”
Buckmaster’s group is now racing against Hou and Chen to get to the finish line first. Hou and Chen have a head start: According to Hou, they have made substantial progress over the past couple years toward improving their approximate solution and completing a proof — and he suspects that Buckmaster and his colleagues will have to refine their approximate solution before they will get their own proof to work. “There’s very little margin for error,” Hou said.
That said, many experts hope that the 250-year quest to blow up the Euler equations is nearly at an end. “Conceptually, I think … that all the important parts are in place,” said Sverak. “It’s just very hard to nail down the details.”
Update: April 12, 2022
This article was updated to include Tristan Buckmaster’s affiliation with the Institute for Advanced Study.