How AI Revolutionized Protein Science, but Didn’t End It
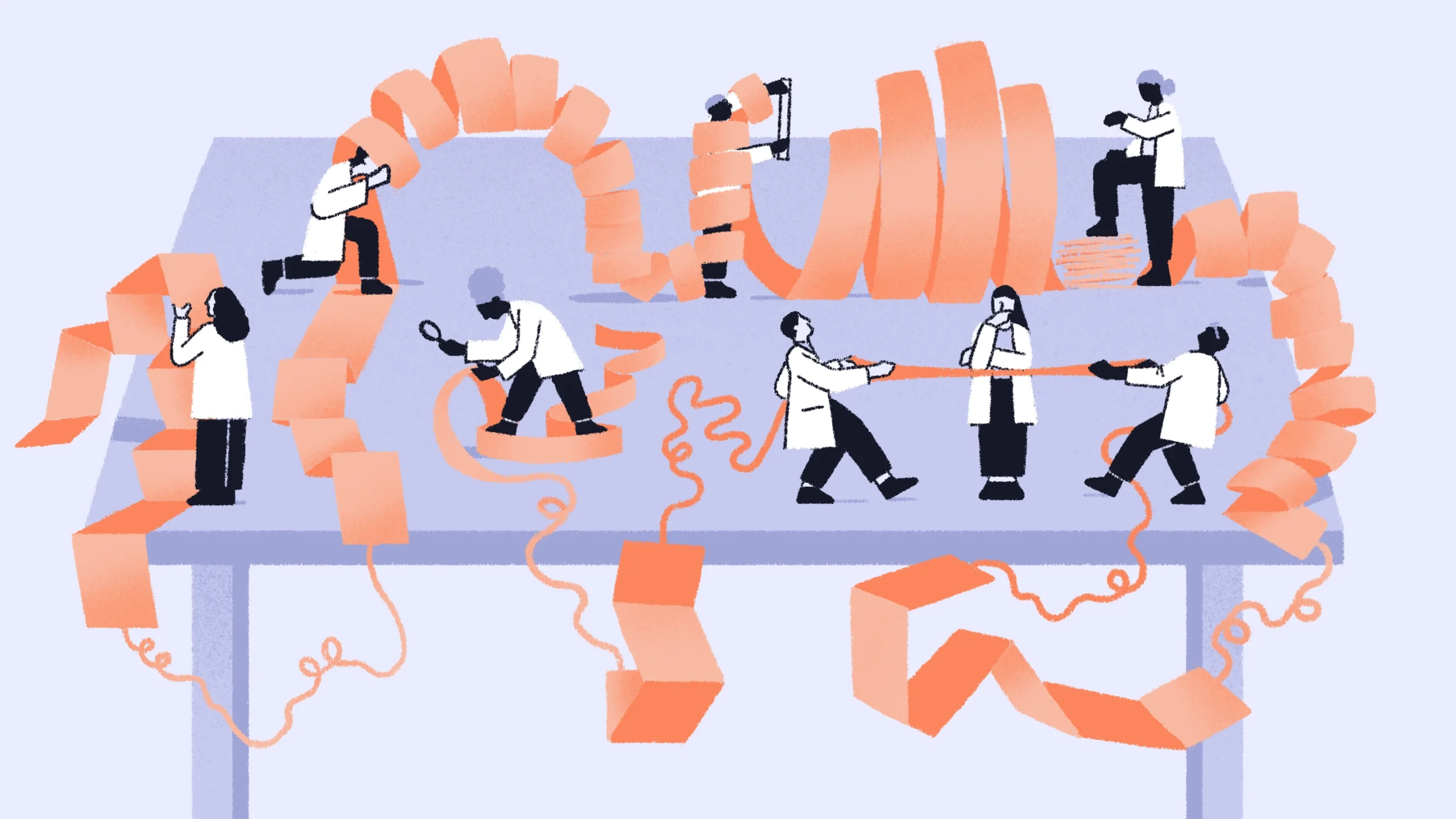
How does a one-dimensional string of molecules fold correctly into its innate three-dimensional shape? This question, known as the protein folding problem, was recently solved by artificial intelligence.
Fran Pulido for Quanta Magazine
Introduction
In December 2020, when pandemic lockdowns made in-person meetings impossible, hundreds of computational scientists gathered in front of their screens to watch a new era of science unfold.
They were assembled for a conference, a friendly competition some of them had attended in person for almost three decades where they could all get together and obsess over the same question. Known as the protein folding problem, it was simple to state: Could they accurately predict the three-dimensional shape of a protein molecule from the barest of information — its one-dimensional molecular code? Proteins keep our cells and bodies alive and running. Because the shape of a protein determines its behavior, successfully solving this problem would have profound implications for our understanding of diseases, production of new medicines and insight into how life works.
At the conference, held every other year, the scientists put their latest protein-folding tools to the test. But a solution always loomed beyond reach. Some of them had spent their entire careers trying to get just incrementally better at such predictions. These competitions were marked by baby steps, and the researchers had little reason to think that 2020 would be any different.
They were wrong about that.
That week, a relative newcomer to the protein science community named John Jumper had presented a new artificial intelligence tool, AlphaFold2, which had emerged from the offices of Google DeepMind, the tech company’s artificial intelligence arm in London. Over Zoom, he presented data showing that AlphaFold2’s predictive models of 3D protein structures were over 90% accurate — five times better than those of its closest competitor.
In an instant, the protein folding problem had gone from impossible to painless. The success of artificial intelligence where the human mind had floundered rocked the community of biologists. “I was in shock,” said Mohammed AlQuraishi, a systems biologist at Columbia University’s Program for Mathematical Genomics, who attended the meeting. “A lot of people were in denial.”
But in the conference’s concluding remarks, its organizer John Moult left little room for doubt: AlphaFold2 had “largely solved” the protein folding problem — and shifted protein science forever. Sitting in front of a bookshelf in his home office in a black turtleneck, clicking through his slides on Zoom, Moult spoke in tones that were excited but also ominous. “This is not an end but a beginning,” he said.
Proteins are molecules that come in hundreds of millions of different shapes. Each one serves a particular biological function, from carrying oxygen through the blood to sparking chemical reactions. The function is typically defined by its shape or structure.
Quanta Magazine; source: RCSB PDB
When Google’s public relations machine churned the news out to the world, the media went wild. Headlines claimed that AlphaFold2 “will change everything.” Protein biologists who had spent their entire careers investigating the structures of single proteins feared that they would lose their jobs. Some claimed that AlphaFold2 would revolutionize drug development; now that biologists could quickly learn proteins’ shapes, they could create new medicines that could target them. Others pushed back, arguing that the results were mostly hype and little would change.
Moult could barely comprehend the news himself. He ended the conference with the question on everyone’s mind: “What now?”
That was three and a half years ago. It’s finally possible to start answering his question.
AlphaFold2 has undeniably shifted the way biologists study proteins. However, while AlphaFold2 is a powerful prediction tool, it’s not an omniscient machine. It has solved one part of the protein folding problem very cleverly, but not the way a scientist would. It has not replaced biological experiments but rather emphasized the need for them.
Perhaps AlphaFold2’s biggest impact has been drawing biologists’ attention to the power of artificial intelligence. It has already inspired new algorithms, including ones that design new proteins not found in nature; new biotech companies; and new ways to practice science. And its successor, AlphaFold3, which was announced in May 2024, has moved to the next phase of biological prediction by modeling the structures of proteins in combination with other molecules like DNA or RNA.
“It’s the biggest ‘machine learning in science’ story that there has been,” AlQuraishi said.
However, there are still massive gaps that artificial intelligence hasn’t filled. These tools can’t simulate how proteins change through time or model them in the context in which they exist: within cells. “AlphaFold changed everything and nothing,” said Paul Adams, a structural biologist who develops algorithms to model the structures of biomolecules at Lawrence Berkeley National Laboratory.
This is the story of how Jumper’s team at Google DeepMind pulled off their coup in protein science, and what it means for the future of artificial intelligence in biology.
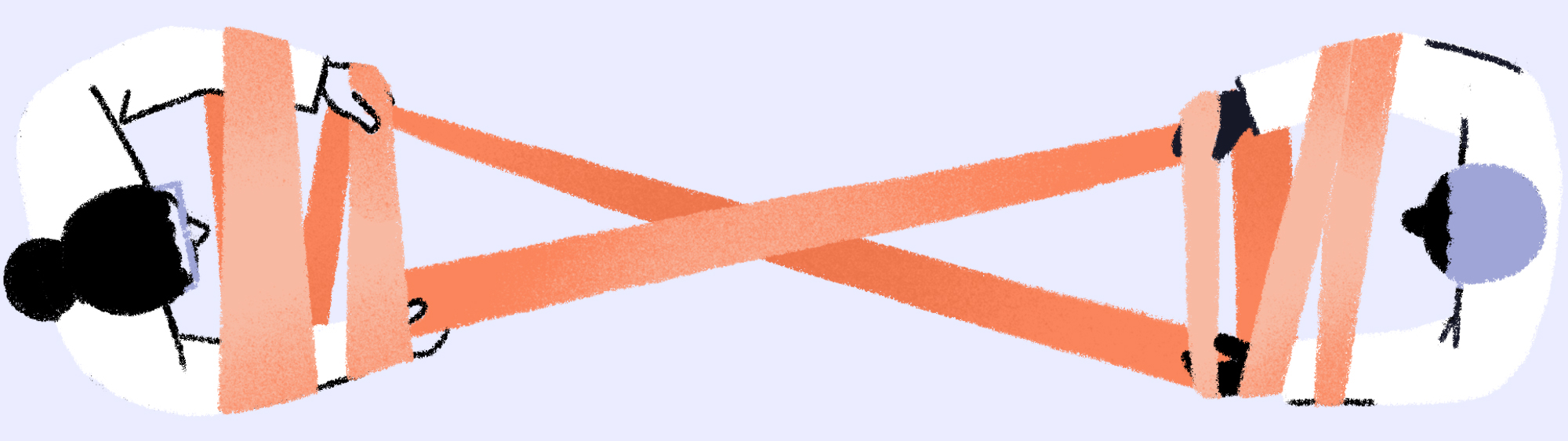
Part 1
Formulating the Problem
A piece of origami paper is little more than pressed wood pulp until it’s folded in specific ways; then it becomes something new. A few precise crimps and flips, and it’s a fortune teller, a paper device that can predict your future. Take the same piece of paper, change a few of the folding steps, and now it’s a winged crane, granting good fortune to its recipient.
Similarly, a long string of amino acid molecules has no function until it spontaneously folds into its innate shape, which biologists call its structure. A protein’s structure determines how it binds to or otherwise interacts with other molecules, and therefore defines its role in a cell.
Mark Belan for Quanta Magazine
There are a couple hundred million known proteins on the planet and many more unknown ones. They do it all: Hemoglobin and myoglobin ferry oxygen around the muscles and body. Keratin gives structure to hair, nails and skin. Insulin enables glucose to move into cells to be converted into energy. Proteins can take on a seemingly infinite number of shapes to match the seemingly infinite number of jobs they do in life.
“Right from the atom all the way to ecosystems, [protein structure] is kind of a lingua franca,” AlQuraishi said. “It’s where everything happens.”
A cell makes proteins by daisy-chaining small molecules called amino acids into long polypeptide strings. The amino acids it chooses depends on the underlying set of instructions provided to it by DNA. Within a fraction of a second of its creation, a polypeptide string bends, buckles and folds precisely into the protein’s final three-dimensional shape. Once off the molecular assembly line, it scurries along to do its biological work.
If proteins didn’t perform this folding process exceedingly well, cascades of disasters would tumble through the body. An incorrectly folded or unraveled protein can lead to toxicity and cell death. Many diseases and disorders, such as sickle cell anemia, are caused by misfolded proteins. And misfolded proteins can aggregate into clumps that are hallmarks of neurodegenerative diseases like Alzheimer’s and Parkinson’s.
Yet no one really knows specifically how protein folding happens. How does the sequence information in these simple molecular chains encode a protein’s complex shape? This is the “most profound question that we can ask,” said George Rose, a biophysics professor emeritus at Johns Hopkins University.
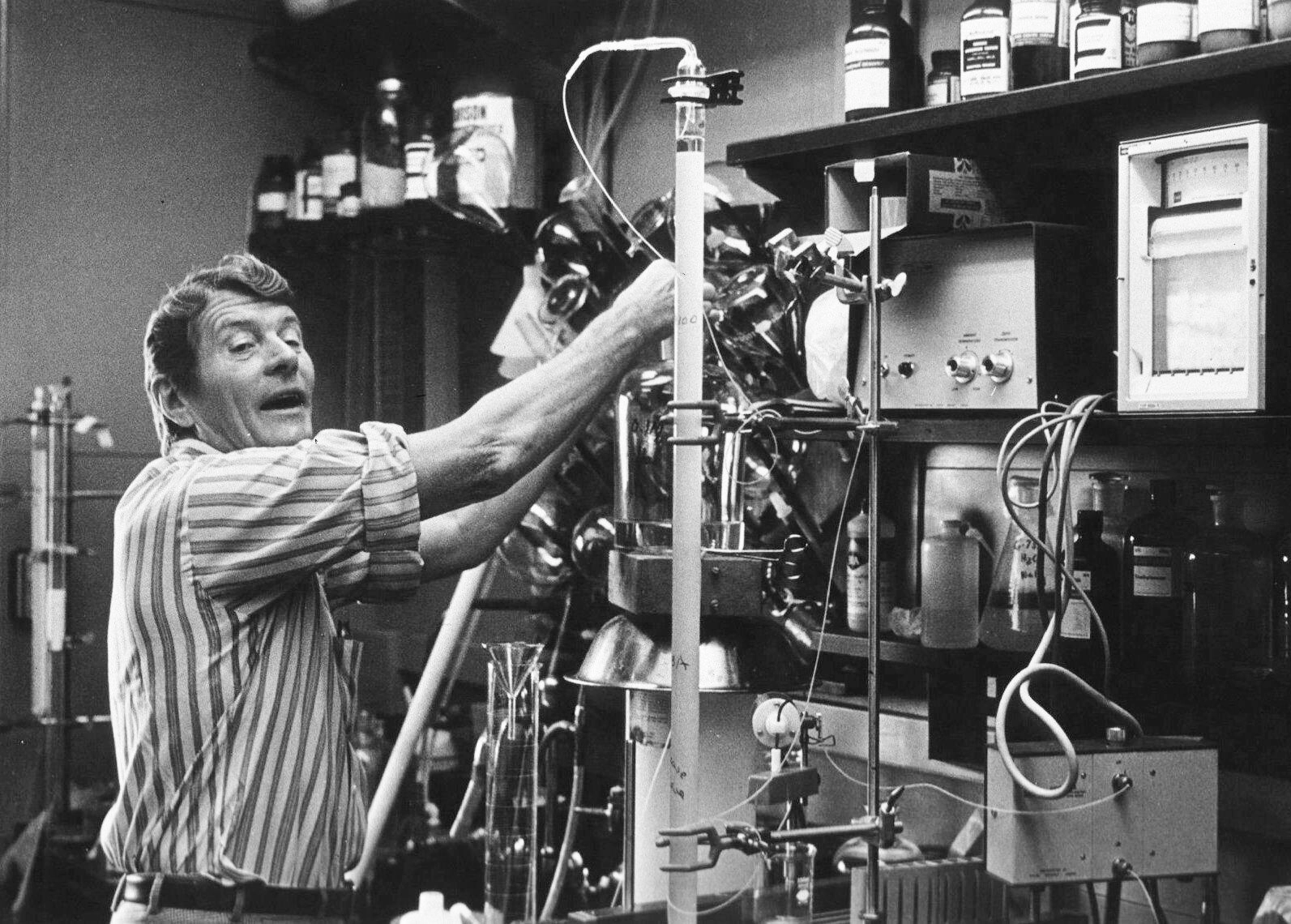
In the 1950s, the biochemist Christian Anfinsen conducted experiments which suggested that a string of amino acids contains an internal code telling it how to fold into a protein — and that there should be a way to predict the protein’s shape from that code. This hypothesis is known as Anfinsen’s dogma.
PBH Images/Alamy
Scientists were probing this question as far back as the 1930s. But efforts really took off in the mid-1950s when the biochemist Christian Anfinsen added proteins to chemical solutions that either unfolded them by breaking their bonds or caused them to fold incorrectly. Then he observed what they did next. Anfinsen saw that the unfolded or misfolded proteins could spontaneously refold into their correct structures. His findings, which later won him a Nobel Prize, demonstrated that proteins form their 3D shapes from an internal code — the one written by their string of amino acids.
Anfinsen hypothesized that there should, therefore, be a way to predict a protein’s shape from the sequence of its amino acids. This became generally known as the protein folding problem.
Once its polypeptide chain is assembled, a protein can fold into its structure within a thousandth of a second — a timescale that perplexed the molecular biologist Cyrus Levinthal. In his 1969 paper “How to Fold Graciously,” Levinthal calculated that if a protein were to try out every possible folding option, it would take an impossibly long time to assemble. Clearly, he mused, something must send the protein down the right folding pathway more directly.
Over time, the protein folding problem has forked into new kinds of problems. Now three main questions are posed: Can a protein’s structure be predicted from its amino acid sequence? What is the folding code? And what is the folding mechanism?
These questions started to burrow into scientists’ brains in the early 1960s when the first experimentally determined protein structures became available. Max Perutz and John Kendrew, two biologists at the University of Cambridge, grew proteins into crystals, bombarded them with X-rays and measured how the rays bent — a technique known as X-ray crystallography. By doing so, they determined the 3D structures of hemoglobin and myoglobin. It took them more than two decades. They shared a Nobel Prize for their discoveries.
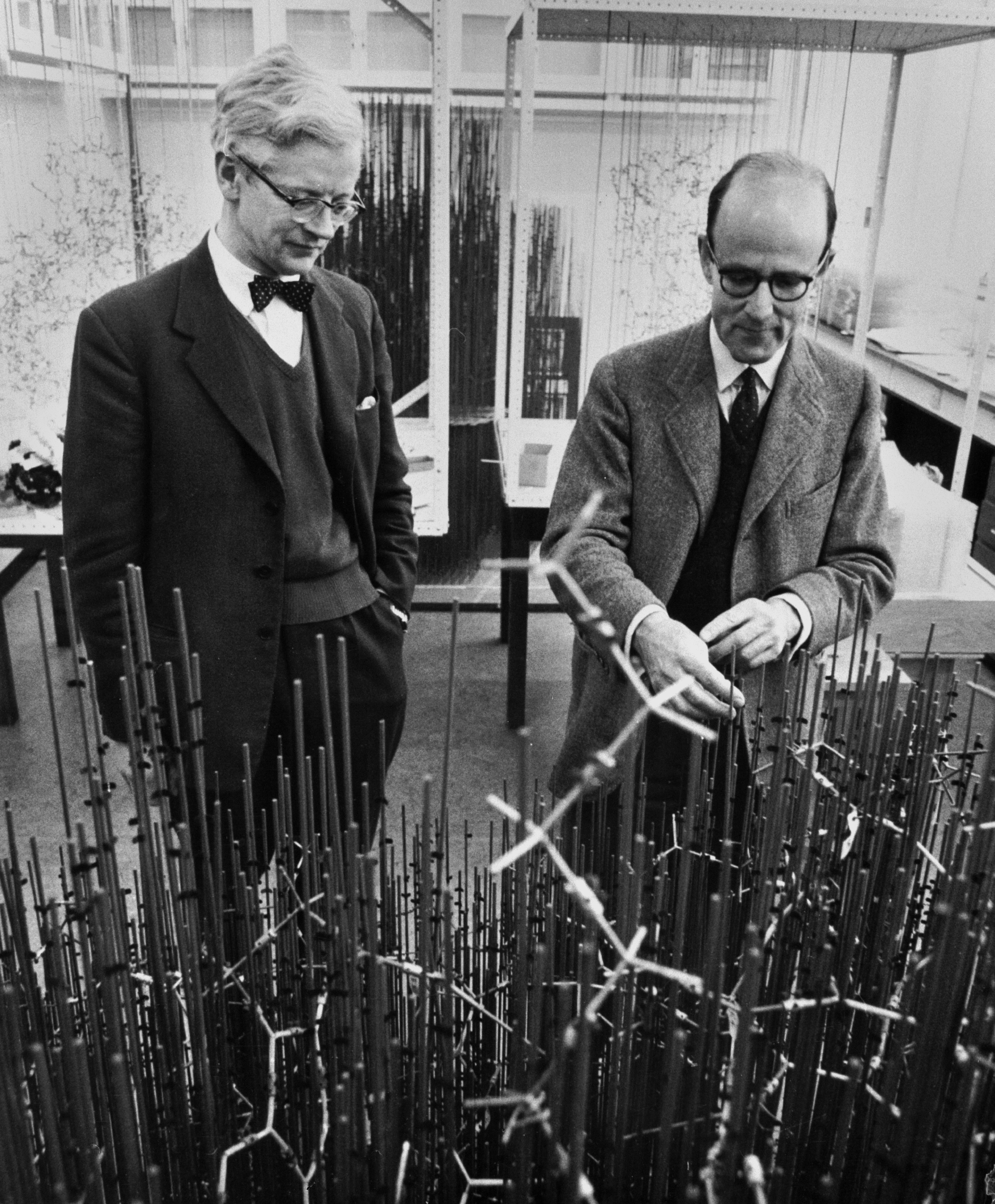
John Kendrew (left) and Max Perutz (right) meticulously uncovered the structures of hemoglobin and myoglobin using X-ray crystallography. Then they built physical models using balls (atoms) and sticks (chemical bonds).
MRC Laboratory of Molecular Biology
Since then, innumerable researchers have strived to understand not just what different proteins look like, but how they come to look that way. “It’s a very human thing to want to know what things look like, because then you can understand how they function,” said Helen Walden, a structural biologist at the University of Glasgow. Some looked into the chemistry of the problem, others into the physics. Experimentalists reconstructed protein shapes through painstaking lab work. Computational biologists hunted for clues with models and simulations, which they programmed and reprogrammed with different combinations of algorithmic rules.
As more structures came along, the protein science community needed a way to organize and share them. In 1971, the Protein Data Bank was founded as an archive for protein structures. Freely available, the data bank became a dependable tool for anyone who needed to know the structure of a protein to probe a biological question.
When the Protein Data Bank opened, it held the structures of seven proteins. By the time Google DeepMind used it to train AlphaFold2 nearly 50 years later, it held more than 140,000 — each laboriously decoded by the scientists known as structural biologists.
The Experimentalists’ Agony
Starting in the mid-1970s, every few months Janet Thornton reliably received a package in the mail. Inside was a 12-inch magnetic tape containing data about new protein structures deposited in the Protein Data Bank. A biophysicist at the University of Oxford, Thornton would eagerly rip open the package so she could analyze the new structures nearly as soon as they were discovered. The first tape Thornton received had only 20 structures on it.
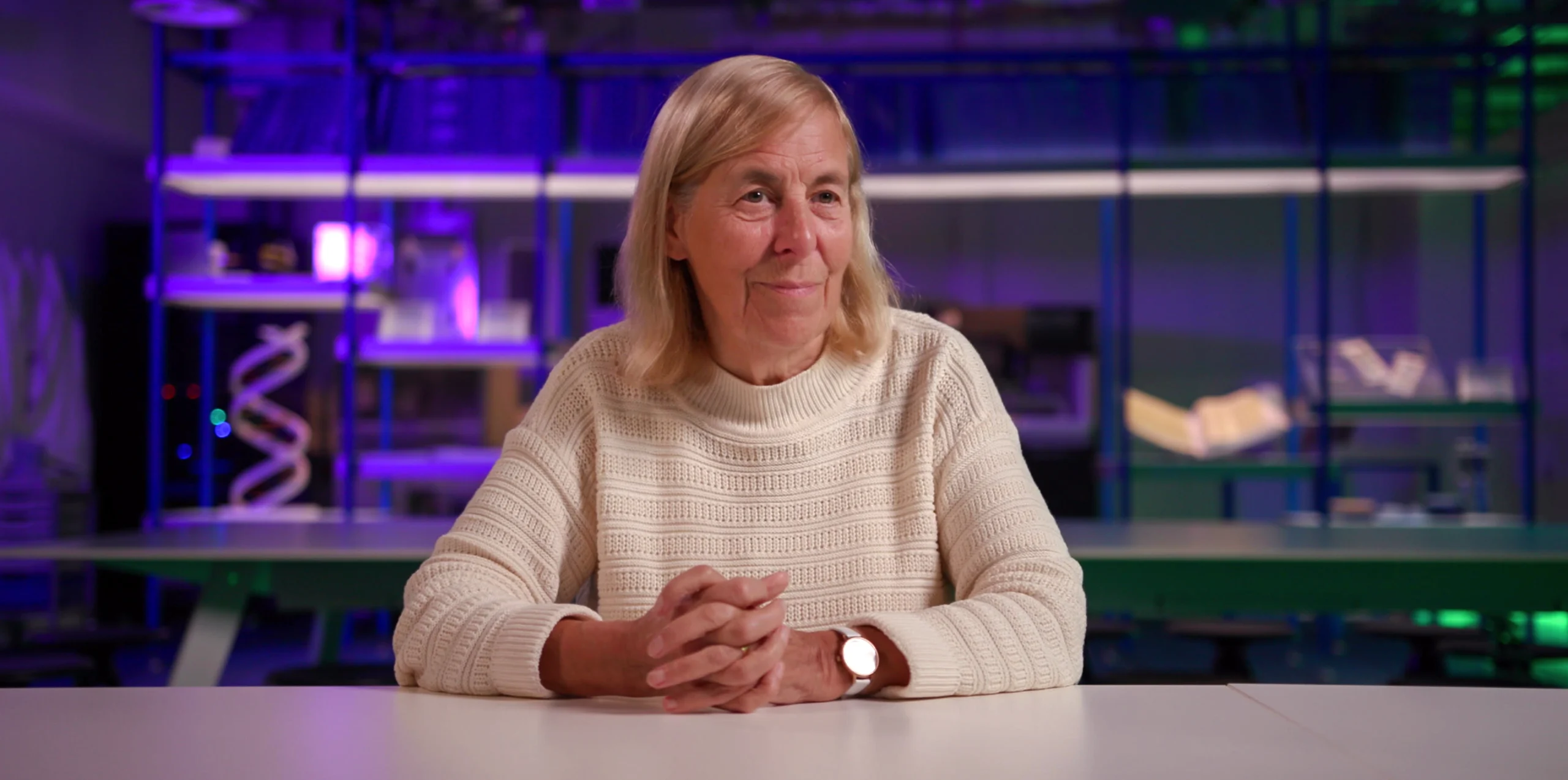
“I had many students who said, ‘I want to come and solve the protein folding problem,’” said Janet Thornton, a structural biologist who retired from the European Molecular Biology Laboratory last year. “But I didn’t have any new ideas, frankly, about how to do that.”
Jeff Dowling, EMBL-EBI
Every one of those proteins represented years of work. Often a doctoral candidate would spend their four or more years in graduate school crystallizing a single protein, collecting data from it or interpreting that data to figure out the folded structure.
Oxford’s department of biophysics was, at the time, one of the world’s centers for X-ray crystallography. There, in 1965, David Phillips, one of the pioneers of protein crystallography, first determined the structure of an enzyme: lysozyme, which the immune system uses to attack bacteria. Using X-ray crystallography, Oxford biophysicists created maps of proteins’ electron density; the areas in which electrons congregated were likely to contain an atom. Thornton and her colleagues printed these electron density maps onto plastic sheets and stacked them one on top of another to create a “contour map” of the protein’s geography, she said.
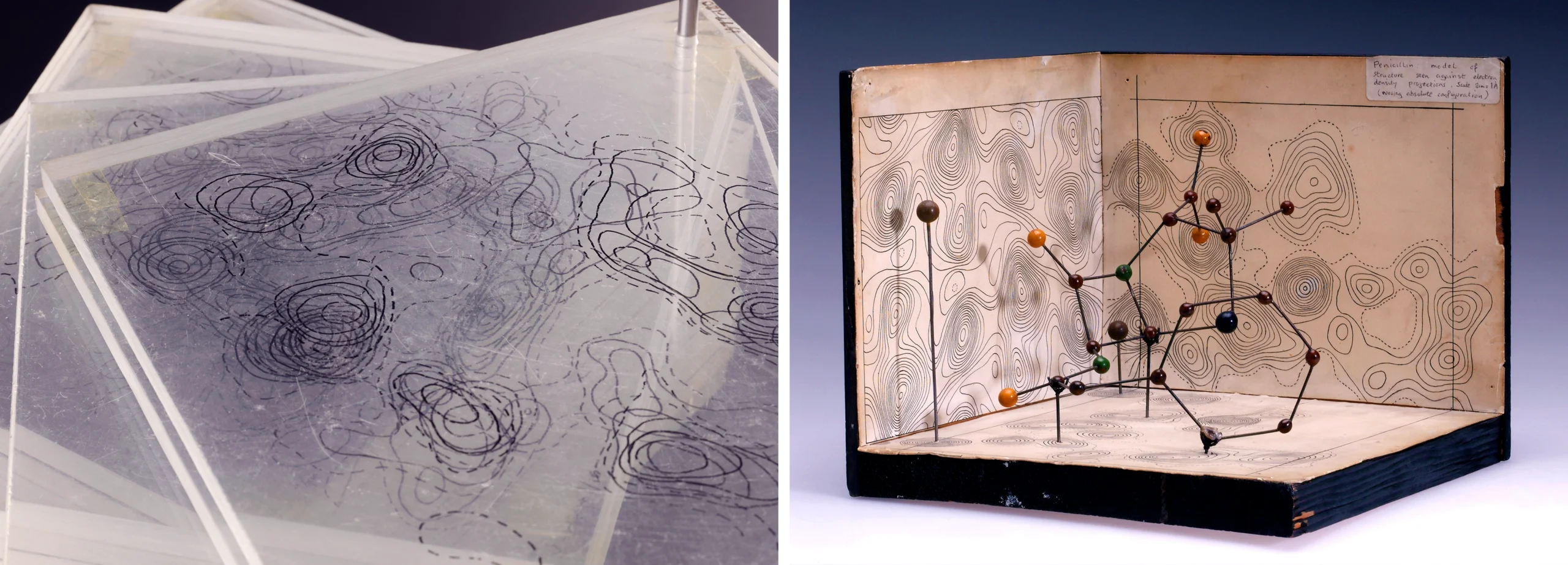
X-ray crystallography helps scientists build electron density maps, which visualize where electrons congregate and therefore where atoms likely sit in a molecule. By stacking the maps on top of one another (left), scientists can deduce the structure of a protein or another molecule like penicillin (right).
Science Museum Group
Then they converted the maps into physical models. They placed their plastic maps into a Richards box, named for the Oxford biophysicist Frederic Richards, who invented the device in 1968. Inside a Richards box, an angled mirror reflected the maps into a workspace, allowing the scientists to see exactly where each atom was located relative to others. Then they physically built their model out of balls and sticks.
This method was cumbersome and restrictive. In 1971, Louise Johnson, who would go on to become an eminent crystallographer, was modeling phosphorylase, which at 842 amino acids was at the time the largest protein anyone had worked on. To model it, Johnson had to climb a ladder into a two-story Richards box, which Oxford constructed especially for her project.
Once a model was complete, scientists used a ruler to measure the distances between atoms to come up with coordinates for the protein structure. “It was archaic,” Thornton said. Then they fed the coordinates into a computer. The computerized version looked like a dense forest, she said, with atoms clumped together in a jumble. Only when Thornton looked at the structure through 3D glasses could she start to see the protein’s topology.
“It was a very torturous process,” Thornton said. “It’s amazing that it got done.”
Mark Belan for Quanta Magazine
Year by agonizing year, they did it. Once researchers were confident in their protein structure, they submitted it to the Protein Data Bank. By 1984, 152 proteins had been deposited. In 1992, that number climbed to 747.
While the experimentalists toiled on with their physical models, another faction of protein biologists — the computational scientists — took a different approach. But as they pondered Anfinsen’s insight that a protein’s structure should be predictable from its amino acid sequence, they got a bit overconfident.
Writing Their Own Rules
As an undergraduate in the early 1960s, John Moult planned to become a physicist. Then he learned about the protein folding problem. “Somebody came and gave a lecture about biology being too important to leave to the biologists,” he said, “which I arrogantly took seriously.” Captivated, he took his career in a different direction.
After he graduated, Moult went into protein crystallography. He decoded the structures of several proteins, including beta-lactamase, a bacterial enzyme that destroys penicillin, and received his doctorate in molecular biophysics at Oxford in 1970. But as he started his postdoc, he tired of the experimentalist approach and began to drift toward the growing field of protein computation. Computational biologists, as opposed to experimentalists, wrote computer algorithms to try and prove that Anfinsen was right: that they could feed a program a string of amino acids to generate a correct protein structure.
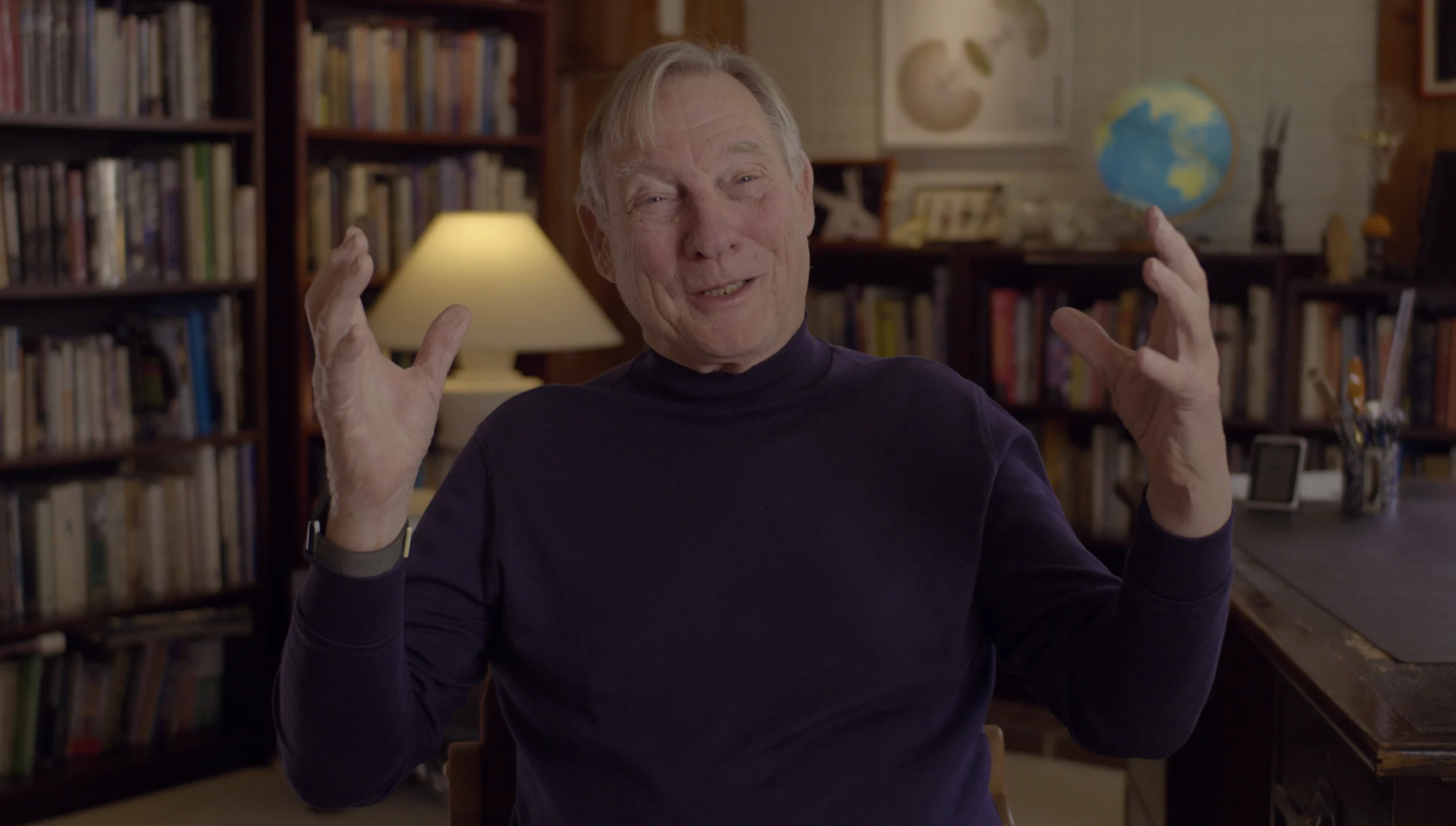
John Moult co-founded the Critical Assessment of Structure Prediction (CASP) experiment to force himself and other computational biologists to test their computer models of proteins against experimentally determined protein structures.
Umit Gulsen for Quanta Magazine
The transition from biological experiments to computation was an uneasy one. Moult was used to the slow, careful work of solving a single protein structure. In his new field, computational papers regularly claimed to have solved the protein folding problem and related sub-problems.
Moult was dubious. “The things that were being published in that area were not as rigorous as I was used to,” he said. “This is not because we’re all a load of crooks in this field. It’s because if you’re doing this sort of computational work, you’re doing it in a virtual world.”
In a virtual world, computationalists wrote their own rules when the rules of the natural world didn’t work. They designed their algorithms so that atoms stuck together in a certain way or the protein always folded to the right or the left. Over time, the models drifted farther away from reality. It’s hard to maintain rigor in a world where you have complete control, Moult said.
Still, he could see the value of both sides. Experimentalists worked precisely but slowly; computationalists worked quickly but were so removed from biophysical realities that they were often wrong.
There must be a way, he thought, to bring the best of both approaches together.
The Stamping Begins
In the early 1990s, Moult and his colleague Krzysztof Fidelis had an idea for bringing discipline to the field’s chaos. They set up a community science experiment that they called the Critical Assessment of Structure Prediction, or CASP.
The idea was simple. As CASP’s organizers, Moult and Fidelis would publish a list of amino acid sequences for proteins whose structures had been recently solved and supplied to them by experimentalists, but for which the results hadn’t yet been published. Then computational groups around the world would try their best to predict the protein’s structure using whatever method they wanted. An independent group of scientists would assess the models by comparing their answers to the experimentally confirmed structures.
The idea took off. CASP soon became a proving ground for computational approaches to the protein folding problem. These were the days before artificial intelligence, when computational approaches involved simulating molecular physics. It was a chance for scientists to put their thinking to the test in a public trial against their peers. “It wasn’t supposed to be a competition,” Thornton said. “But it actually has turned out to be a competition.”
Every two years, scientists gathered at the Asilomar conference center, an old chapel near Monterey, California, that used to be a Methodist retreat. During these conferences, the organizers announced the competition’s results and the computationalists gave talks about their methods and approaches. Moult encouraged attendees to stamp their feet on the wooden floors if they didn’t like what they were hearing.
“There was, at the beginning, quite a lot of stamping,” he said.
It was “almost like a drum,” recalled David Jones, a professor of bioinformatics at University College London who studied under Thornton. The biologists stamped if the talks got bogged down in details. They stamped if claims were overblown. They stamped if speakers were repetitive or too much in the weeds. But it was friendly stamping, Jones said: “It wasn’t nasty.”
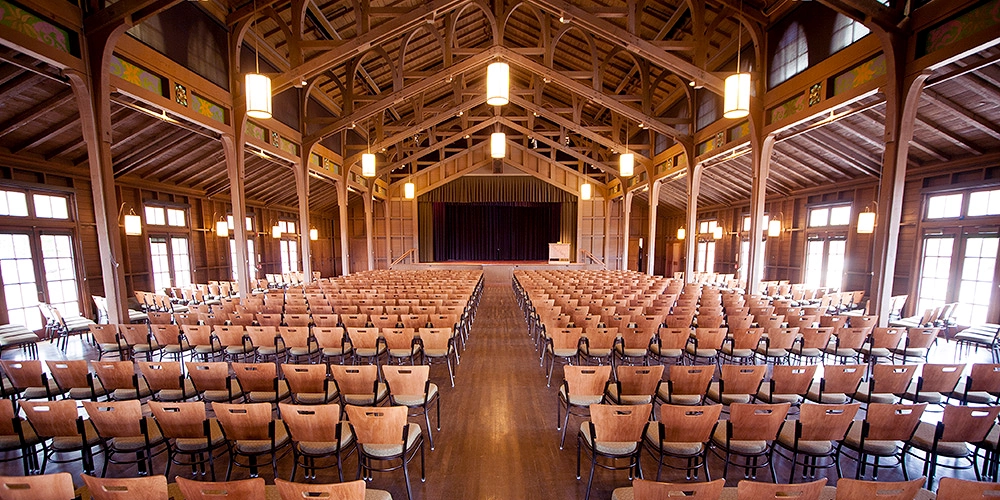
The early CASP conferences were held at the Asilomar conference center in Monterey, California. When attendees stamped on the wooden floors, it sounded like a drum.
Aramark Destinations
Whatever the reason, when the echoes of stamping started ringing in a speaker’s ears, it was embarrassing. “Thank God I never got stamped on,” Jones said. One year, he and his colleagues presented a computational method called threading, in which amino acid sequences were woven through known protein structures to search for a fit. They didn’t do too badly. “We were quite pleased. … It was all downhill after that,” Jones recalled, laughing. “No, it was fun.”
There was a lot of excitement back then, said Silvio Tosatto, a professor of bioinformatics at the University of Padua. “People thought that they could become millionaires because they had the right algorithm, and some other people thought that they would immediately win the Nobel Prize.”
Neither of those things happened during the early years. When asked what the CASP submissions were like during that time, Moult paused. “‘Random’ is a good word,” he said.
Some methods performed better than expected, such as “homology modeling,” which compared the structures of known proteins to deduce the structures of unknown ones. Others were a dead loss. Most structure predictions were “tortured-looking objects,” Moult said.
“I was loving seeing them fail,” joked Anastassis Perrakis, a structural biologist at the Netherlands Cancer Institute and Utrecht University who gave experimentally determined structures to CASP organizers for use in the competition. “It’s not rivalry, but we like to tease each other in science.”
Through this process, clear leaders emerged. In 1996, after the second CASP wrapped up, a young man named David Baker asked Jones to share a taxi to the airport. Baker had seen Jones’ talk and was working on his own computational model. He didn’t have it ready for this CASP, but he wanted to chat about it. Jones listened to his ideas in the cab and never expected to see him again.
At the next competition in 1998, Baker blew the doors wide open with his algorithm Rosetta. He became “the man to beat,” Jones said.
Algorithms like Rosetta modeled interactions between the atoms of amino acid molecules to predict how they would fold. They “showed that you actually could predict protein structure,” Baker said. “But it wasn’t good enough or accurate enough to be useful.”
In 2008, humans were still beating the computers. Baker, who by then was running his own lab at the University of Washington, created a free online computer game called Foldit, in which players folded strings of amino acids into protein structures. In a paper published in Nature, his team reported that human Foldit players outperformed Rosetta in modeling proteins.
But the human lead wouldn’t last long. In the early 2010s, important breakthroughs in a concept known as co-evolution propelled the field forward and would later turn out to be critical for artificial intelligence. The idea, which had been around for decades, was straightforward: By comparing closely related sequences of amino acids in hundreds to thousands of proteins, scientists could identify the amino acids that had mutated — and, importantly, determine whether they’d mutated in step with others. If two amino acids changed together, they were likely linked in some way. “You start to be able to say, ‘Well, these two things are probably close together in space,’” said Adams, the structural biologist at Berkeley Lab.
Mark Belan for Quanta Magazine
But until the early 2010s, such predictions of which amino acids were in contact were dismal. Their accuracy hovered between 20% and 24%. Then scientists noticed that their statistical methods were introducing errors, suggesting that some amino acids were in contact when they weren’t. Later, Moult learned that statisticians had been keenly aware of this kind of error for decades. When you looked back, he said, you’d think, “How could I be so stupid?”
Computational biologists cleaned up the statistical tools. By 2016, the accuracy of contact prediction had shot up to 47%. Two years later, it reached 70%. Baker’s algorithm built on this success: In 2014, Rosetta produced two protein structures so accurate that a CASP assessor thought Baker might have solved the protein folding problem.
The co-evolution insights were “fantastic,” Adams said. Without using machine learning, co-evolution was “one of the big things that came that really pushed that field forward.”
However, it got the field only so far. Co-evolution required an abundance of similar proteins to compare, and experimentalists weren’t solving protein structures fast enough to supply computationalists’ needs.
The years flowed by in a punctuated equilibrium, Moult said, using a term from evolutionary biology. Sometimes it felt as though no good ideas had evolved for a billion years — and then something exciting would happen.
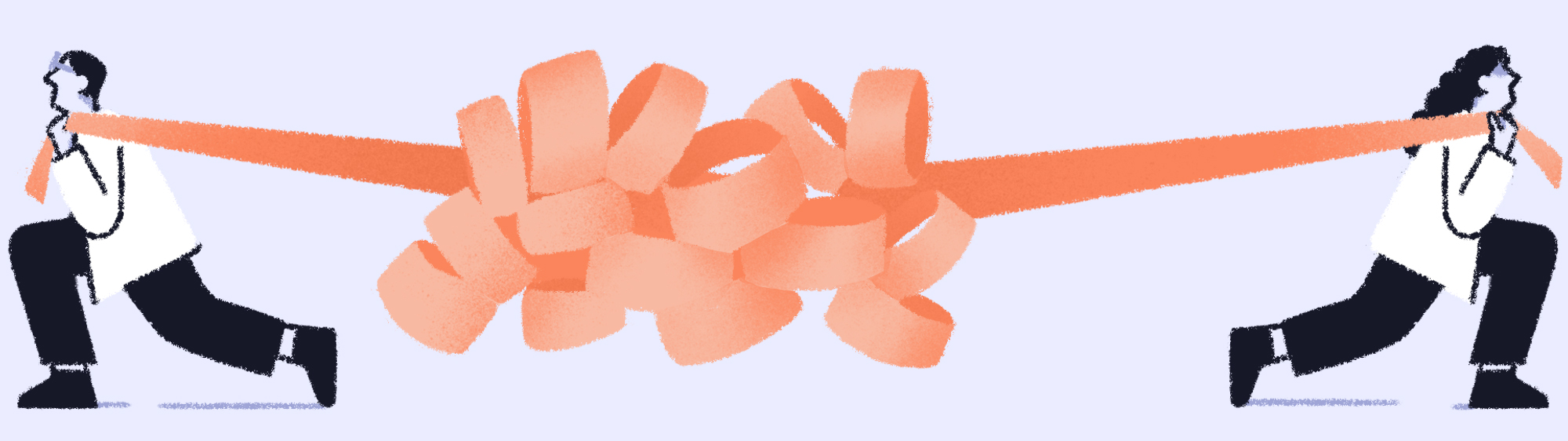
Part 2
Off the Deep End
In 2016, David Jones caught a glimpse of the future in a new paper in Nature. Researchers from Google DeepMind, an artificial intelligence team based in London, detailed how their algorithm, which used a method known as deep learning, had beaten a human champion at an ancient board game called Go.
Jones was amazed. “Things are happening,” he recalled thinking at the time. “I’m really going to have to get into this deep learning.”
Deep learning is a flavor of artificial intelligence loosely inspired by the human brain. In your brain, molecular information is sent across an interconnected web of brain cells called neurons. Neurons have little arms called dendrites that grab molecules dispatched by neighboring neurons which tell the receiving neuron either to fire and propagate a signal or not to fire.
“If enough activity comes at that neuron, then that neuron is going to fire,” said Michael Littman, a computer science professor at Brown University. That results in another wave of molecules being released to the next neuron.
In the 1950s, computer scientists realized that they could wire electronic bits together to create “neural networks.” Every unit in the neural network is a node, which researchers likened to a neuron: A neuron receives information from other neurons, then calculates whether to fire toward the next ones. In neural networks, information propagates across multiple layers of neurons to produce a particular outcome, like recognizing a dog in an image.
Mark Belan for Quanta Magazine
The more layers of neurons you have, the more intricate calculations you can perform. But early neural networks were made up of only two layers. In the 1990s, that number increased to three, and it stayed there for two decades. “We could not figure out how to reliably create networks that were deeper than that,” Littman said.
Structural biologists, including Jones and Moult, had tried using neural networks in protein science since the 1990s, but the limitations of shallow networks and sparse data held them back. Then, in the early 2010s, computer scientists learned how to better structure neural networks to allow reliable training of more layers. Networks deepened to 20, 50, 100 and then thousands of layers. “To distinguish that from the way we were doing it in the ’90s, people started to call it ‘deep learning,’” Littman said. “Because if machine learning people are good at one thing, it’s making up sexy names.”
Deep learning transformed artificial intelligence, leading to algorithms that excelled at recognizing features in photos or voices — and, it turns out, at beating humans in games.
In March 2016, when DeepMind co-founder Demis Hassabis was in Seoul watching his AI system AlphaGo beat a human world champion in the ancient game of Go, he flashed back to playing Foldit as an undergraduate. He wondered: If DeepMind researchers could write an algorithm to mimic the intuition of Go masters, couldn’t they write one to mimic the intuition of Foldit gamers, who knew nothing about biology but could fold proteins?
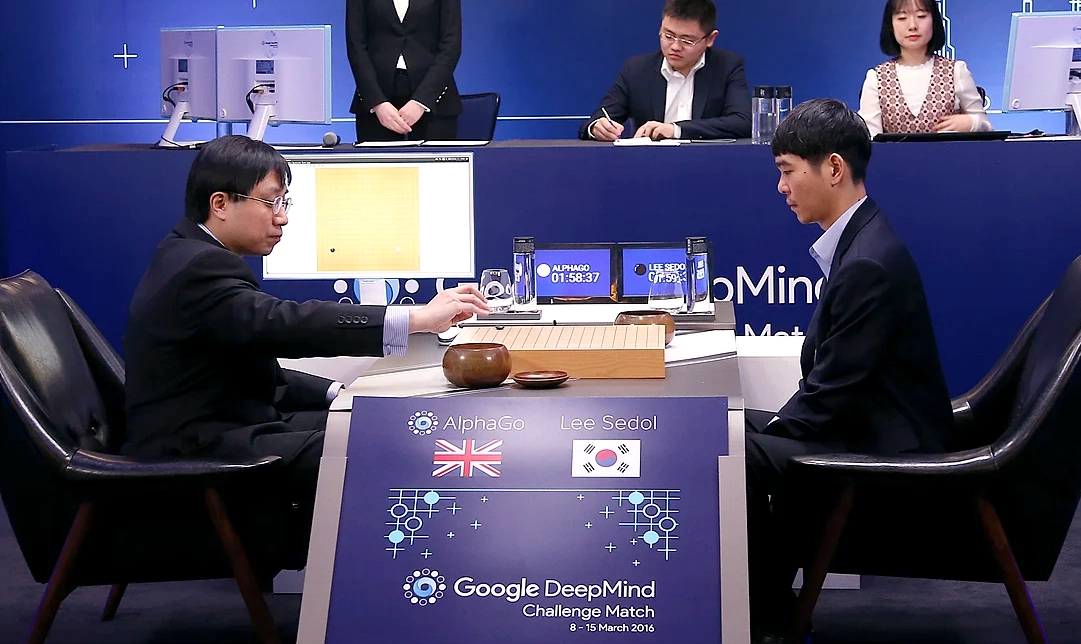
In 2016, AlphaGo, an AI system by Google DeepMind, defeated Lee Sedol (right), the world champion in the ancient game of Go. Its ability to mimic human intuition drew biologists’ attention to the potential power of deep learning in protein science.
Google DeepMind
Jinbo Xu, a professor at the Toyota Technological Institute at Chicago, also recognized the potential of using deep learning to attack the protein folding problem. He was inspired by what these networks were doing in image recognition. By then, computer scientists had had great success with convolutional networks, which program deep learning algorithms to break images into pieces and identify patterns between them. Xu brought this technique to protein folding. He used a mathematical object called a matrix to represent which amino acids were close together in space, then fed the data into a convolutional network as an image. The algorithm looked for patterns among these images to predict the 3D coordinates of the atoms that make up a protein.
In 2016, Xu posted a preprint of this work on arxiv.org (it was later published in PLOS Computational Biology) that “was quite influential” for the field, Moult said. It showed people “the sort of things you might do with deep learning.”
Before long, protein structure groups started dabbling in deep learning. AlQuraishi and his team were the first to develop an approach that could directly predict protein structure exclusively with neural networks, in what’s called an “end-to-end” method — it just didn’t work very well. Others wondered how they could dip their toes into a new approach that felt so momentous.
“I didn’t know exactly what I wanted to do with deep learning, but I realized I needed to be doing deep learning,” Jones said.
He had started to write grant applications to find his way in when he received an email from Google DeepMind. They asked Jones about the CASP competition and offered help. “I just assumed they meant: We’ve got lots of computer power,” Jones said.
After he met them, it became obvious that Google had larger ambitions. But to pull them off, the tech giant would need more scientific brainpower.
A New Player on the Field
In 2016, when Jones started working as a consultant for Google DeepMind on a project that would later be known as AlphaFold, John Jumper was completing his doctorate in theoretical chemistry at the University of Chicago.
As a teenager, Jumper had taught himself how to program computers. He also had a knack for physics. So when it came time to go to college, he decided to study math and physics even though his parents, both engineers, were worried he’d never be able to find a job.
“I thought I was going to be a ‘laws of the universe’ physicist all the way through,” Jumper said. “I’ve always loved this notion of discovering something true of the universe.”
As an undergraduate at Vanderbilt University, he joined a collaboration with researchers at the Fermi National Accelerator Laboratory to study the strange properties of subatomic particles called quarks. One day, when he was sitting at a lunch table with the researchers, he got some sour news. “So, this experiment that we’re working on — when’s it going to turn on?” Jumper recalled asking them. One of the professors said that he’d probably retire first. The other, a bit older, said he might not live to see it.
“I wanted to do science in a little shorter timeframe than that,” Jumper said. After finishing undergrad, he started a doctoral program in theoretical condensed matter physics — and quickly dropped out. He had gotten a job at D.E. Shaw Research, a New York company that, at the time, was creating basic simulations of proteins. By understanding how proteins move and change, they hoped to better understand the mechanisms of various ailments like lung cancer.
It was the first time Jumper grasped the potential significance of his work. “It’s about health and extending people’s lives,” he said. For the next three years, Jumper modeled protein movements on the company’s supercomputers, which they had built specifically to simulate molecules faster. “I was doing more simulation on a Tuesday of some weeks than I was going to do in my entire Ph.D.,” he said.
In 2011, he gave graduate school another shot, this time studying theoretical chemistry at the University of Chicago. He was still interested in protein structure and movement. But he was frustrated by the slow pace of academia. “I no longer had this access to this custom computer hardware” he had used at D.E. Shaw, Jumper said. He wondered if he could use artificial intelligence — “at the time we called it statistical physics” — to reach a level of quick simulation that otherwise required advanced machines. He began dabbling in machine learning and neural networks.
During this time, he also started thinking about the protein folding problem. He suspected that the problem should be solvable with the training data available in the Protein Data Bank — by 2012, it contained more than 76,000 protein structures.
“I believed that the data were sufficient,” Jumper said. But “the ideas weren’t.”
In 2017, Jumper heard a rumor that Google DeepMind was getting into protein structure prediction. He had just completed his doctorate, using machine learning to simulate protein folding and dynamics. He applied for a job as a research scientist.
“The project was still secret,” he said. If he raised the subject of protein folding in an interview, the DeepMind team changed the subject. “You can only do that so many times before I’m pretty sure what you’re doing,” Jumper said.
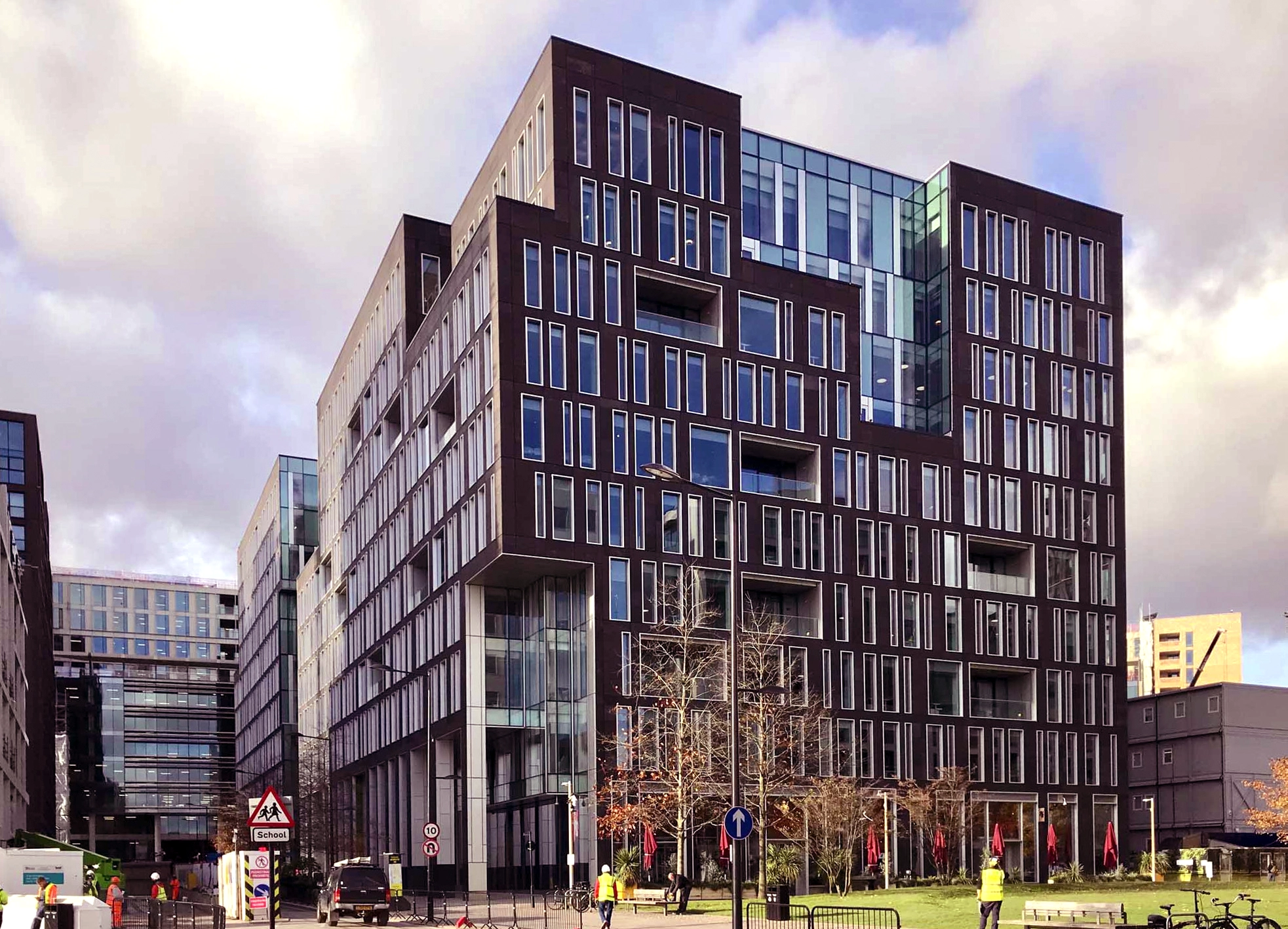
Google DeepMind is headquartered in London.
Buildington
In October 2017, he arrived at DeepMind’s London office. With Jones’ help as a consultant, the team was already deep into the development of AlphaFold. “It was a great fun time where we were just throwing ideas at the wall,” Jones said. “Eventually, a good core idea emerged, and they ran with it.”
To train their algorithm, the DeepMind team used more than 140,000 structures from the Protein Data Bank. They fed this information into a convolutional network, but didn’t change much about the AI architecture itself. It was “standard machine learning,” Jumper said.
By the spring of 2018, AlphaFold was ready to join CASP and compete against bona fide protein scientists. “It’s a bit like Formula One racing,” Jones reflected. “You think you’ve built the best car, but you just don’t know what the other teams have built.” The stakes felt high. The DeepMind team debated whether they should compete anonymously; they didn’t want to risk humiliation.
“Nobody wants to fail,” Jones said. In academia, it’s part of the job; you fail, and you move on because you don’t have a choice. “But obviously if you’re a multibillion-dollar tech company, it’s not a good look if you tried to do something and failed.”
They ultimately decided to submit their results under the Google DeepMind name. A few months before the December meeting, Jones heard from CASP’s organizers. They suggested that the DeepMind team come along to the meeting because AlphaFold had performed really well.
Their victory wasn’t massive — they were about 2.5 times better at predicting protein structures compared to the next-best team — but their win made an impression. “It was clear something interesting had happened,” Moult said.
Rebooting the Algorithm
The win should have energized the DeepMind team. But they knew they weren’t close to solving the protein folding problem. Hassabis had gathered them a few months earlier. “Are we going to go after solving this or not?” Jumper recalled him saying. “If not, let’s find problems that we can have this really, really big impact on.”
“We had this moment where we really decided: We are going to go after solving it,” Jumper said. They went back to the drawing board.
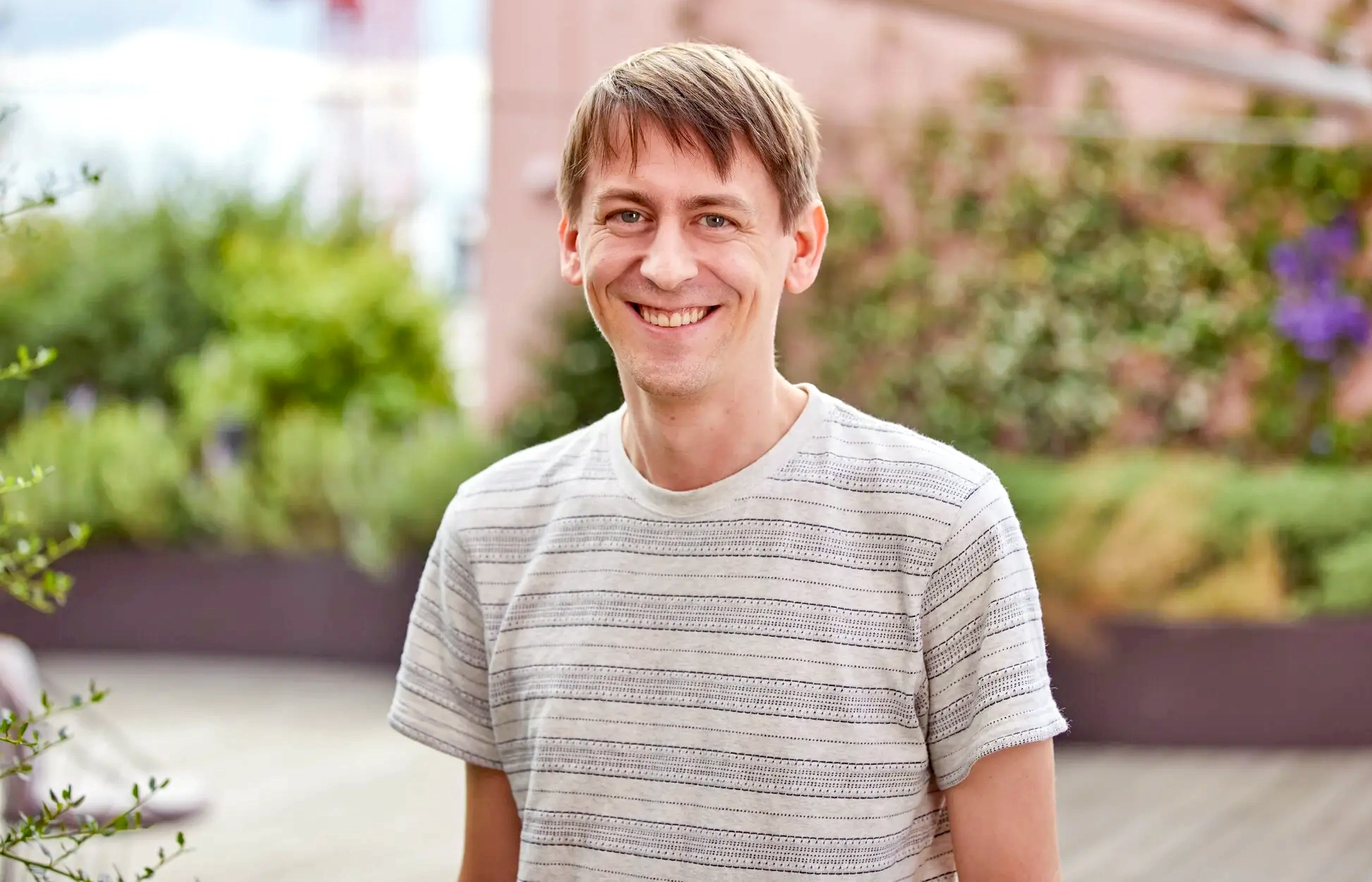
John Jumper suspected that biologists had studied enough protein structures to solve the protein folding problem. “I believed that the data were sufficient,” said Jumper, who started working at Google DeepMind in 2017. But “the ideas weren’t.”
Google DeepMind
With his diverse background in physics, chemistry, biology and computation, Jumper brought original insights to brainstorming sessions. Soon, he was leading the team, which had grown from six to 15 people. “There was something very unique going on,” said Raphael Townshend, who interned at Google DeepMind in 2019 and later founded Atomic AI, an AI-driven biotech company.
In academia, experts are often siloed from one another, each pursuing independent projects with little collaboration. At DeepMind, experts in statistics, structural biology, computational chemistry, software engineering and more worked together on the protein folding problem. They also had the massive financial and computational resources of Google behind them. “Things that would have taken me months to do as a Ph.D. student, I was doing in a single day,” Townshend said.
The London DeepMind office was high-energy, he said, and much of that energy was generated by Jumper. “He’s a true genius, I would say, and also a very humble person,” said the computer scientist Ellen Zhong, who interned at DeepMind in 2021 and is now an assistant professor at Princeton University. “He was beloved by the team.”
Under Jumper’s leadership, AlphaFold was reconstructed. DeepMind designed a new type of transformer architecture — a type of deep learning that has “powered basically every single machine learning breakthrough that’s happened in the last five years,” Townshend said. The neural network tweaked the strength of its connections to create more accurate representations of the data, in this case protein evolutionary and structure data. It ran that data through a second transformer to predict the 3D structure of a protein. The algorithm then honed the structure further by running it, together with some of the revised data, back through its transformers a few more times.
Mark Belan for Quanta Magazine
When they first started working on AlphaFold2, their algorithm was “terrible, but not as terrible as we expected,” Jumper said. “[It] made helices that kind of vaguely looked like a protein.” But as they honed it further, they noticed enormous increases in the efficiency and accuracy of their predictions.
“It was actually terrifying,” Jumper said. If it’s working too well, that usually means “you’re doing the wrong thing.” They checked, but there wasn’t a problem. It was simply working.
The team decided to run an internal experiment to see whether their system would be helpful to biologists. They identified roughly 50 papers published in high-end journals like Science, Nature and Cell that not only described a new protein structure but also generated insights about the protein’s function from the structure. They wanted to see if AlphaFold2 would stand up to the experimentalists’ laborious approach.
They entered the amino acid sequences. AlphaFold2 ran its prediction engine. For each sequence, it spat out a prediction close to the experimental structure presented in the papers. However, in the team’s view, it wasn’t accurate enough. The structures were missing key details that the experimentalists learned about their proteins. “You feel like you’ve finished the race and it’s like finding out that you’ve got the second half,” Jumper said.
The team further honed the system over the next six months, minor improvement by minor improvement. A few weeks before the protein candidates were released for the 2020 CASP competition, they performed another usefulness test. Jumper was satisfied. Google DeepMind submitted their predictions to CASP in the spring of 2020. And then they waited.
The Earthquake
In early summer, Moult received an email from a CASP assessor: “Look at this, it’s pretty impressive.” Attached to the email was a protein structure solved by Google DeepMind. Moult was indeed impressed, but he thought it was a one-off.
Then he got another email, and another. “That’s strange,” he recalled thinking. There were three, four, a whole slew of near-perfect protein predictions — and all from DeepMind. By the end of the summer, “we rapidly realized … something very, very extraordinary had happened,” Moult said.
CASP assessors score each submission by comparing the predicted protein structure to its proven experimental structure. A perfect score, in which the model and reality match atom by atom, is 100. Moult had always believed that anything above 90 would indicate that an algorithm had effectively solved a protein’s structure. Most of AlphaFold’s structures hit or surpassed the 90 mark.
A few months before the meeting, Moult called Jumper with the news. “I cursed out loud,” Jumper recalled. “My wife asked if I was OK.”
In December 2020, less than a year into the Covid-19 pandemic, Jumper presented AlphaFold2 over Zoom at the virtual CASP meeting.
Like the rest of the attendees, Jones watched from home. “I was just stuck … watching this unfold,” he said. “There’s no outlet because your colleagues aren’t nearby. … We’re all under lockdown so we can’t go anywhere.”
For anyone who wasn’t a neural network expert, the ideas were complex. Even so, the conclusions were clear. DeepMind had solved the structure prediction part of the protein folding problem. AlphaFold2 could accurately predict the structure of a protein from its amino acid sequence.
“Ugh, my favorite subject is dead,” Jones recalled thinking. “DeepMind shot it, and it’s the end.”
For years, Anastassis Perrakis had contributed unpublished experimental results to CASP for the competition. When he saw AlphaFold2’s results for a protein his team had sweated over, he thought, “Uh-oh.” AlphaFold2 had gotten it perfectly right.
Alone at home in lockdown, the scientists were united in thinking that the world of protein science had changed forever. As its inhabitants looked out across the new landscape, they had one question in mind: What now?
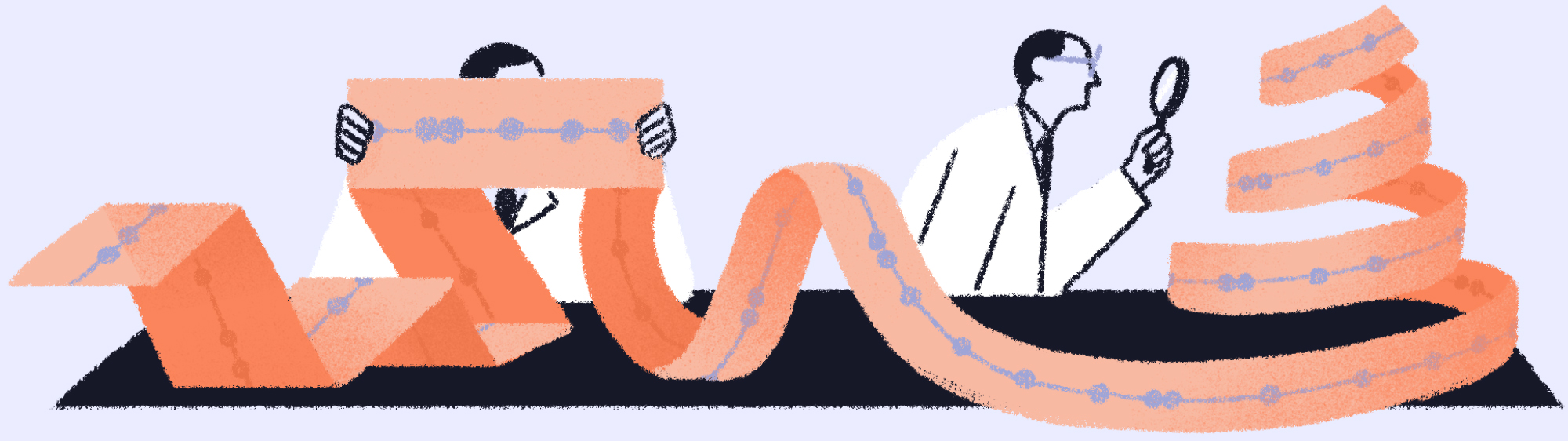
Part 3
Shock and Awe
Structural biology suddenly became unstructured.
At first there was “a lot of soul searching,” said Silvio Tosatto, who had competed in CASP since its earliest days. Some structural biologists feared their jobs would become obsolete. Others grew defensive and claimed that AlphaFold2 wasn’t accurate.
The computational biologists who had been trying to solve this problem, some for decades, found the moment bittersweet. In a blog post he wrote after CASP, AlQuraishi cited an attendee who described feeling like someone whose child had left home for the first time.
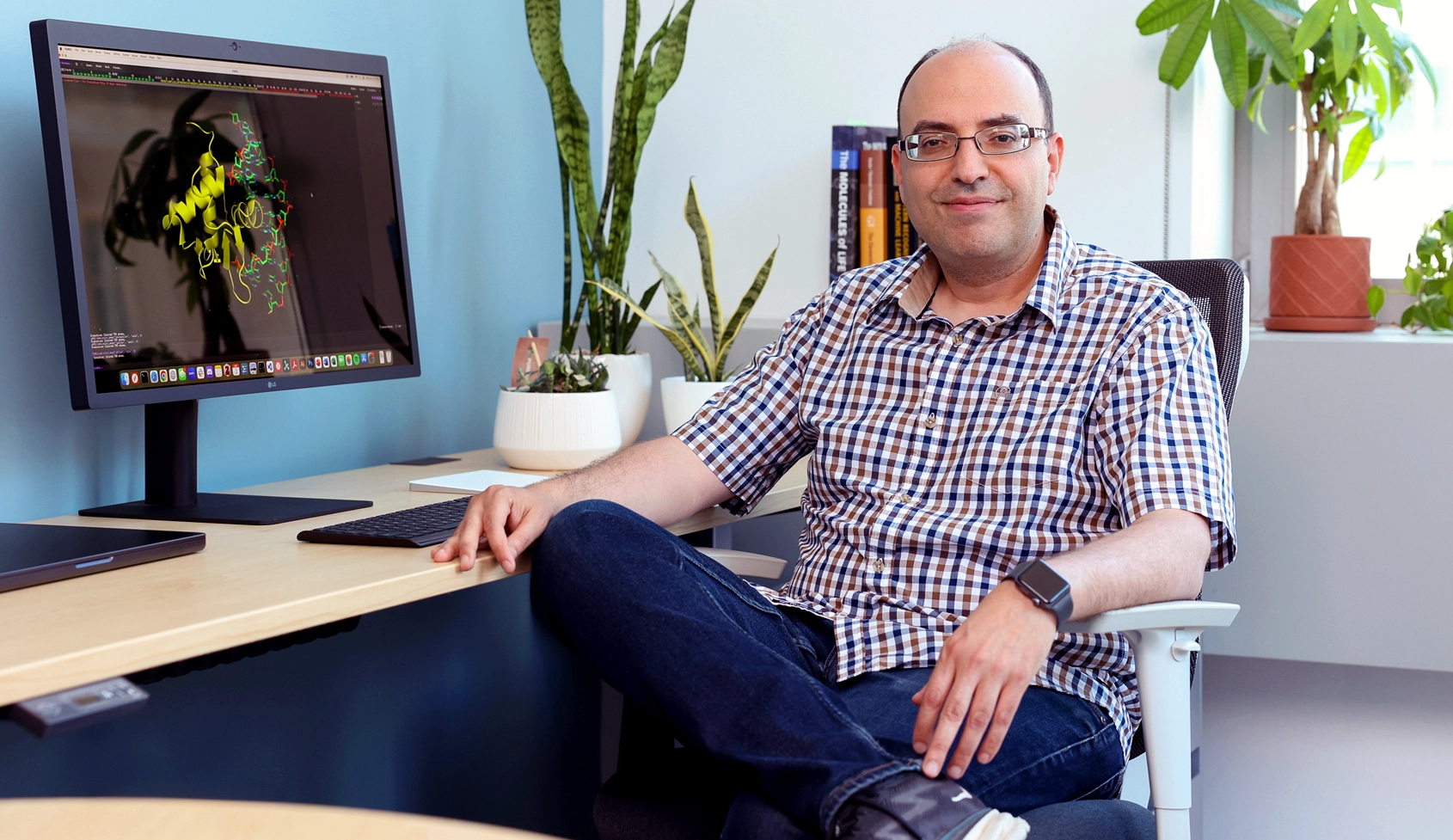
Mohammed AlQuraishi, a systems biologist at Columbia University’s Program for Mathematical Genomics, hopes that deep learning will be able to simulate an entire cell and all the structures and dynamics within it by 2040.
Nicole Pereira
But even amid their trepidation around this shiny new tool, many scientists were ecstatic. Those who didn’t do structural work used to have to collaborate with structural biologists to determine protein structures for their broader experiments. Now, they could just press a few buttons and get the structure on their own.
In the media, AlphaFold2 became the shiny new artificial intelligence breakthrough that would “change everything.” But it took months and years for scientists to tease apart what AlphaFold2 could and couldn’t do. Around six months after Jumper’s talk, Google DeepMind published their results and shared AlphaFold2’s underlying code. “When AlphaFold2 was out, the next day we were trying to install it in our GPU servers,” Perrakis said. Biologists began to play.
“I expected [AlphaFold2] to fall down,” Thornton said. “But actually my impression is that it’s been remarkably successful.”
It started to become clear that rather than being a threat, AlphaFold2 might be a catalyst for accelerating research. Rather than put structural biologists out of a job, it gave them a new tool to do their jobs better. “If you view a structural biologist simply as a technical specialist who works out the structure of proteins, then yes, of course structural biologists are out of the job,” Walden said. But that would be like saying that the Human Genome Project made genomicists obsolete because they could no longer publish a paper describing the sequence of a single gene.
In many cases, a structural biologist’s goal is to discover the function of a protein. With AlphaFold2, they could create a hypothesis within minutes rather than wait for months or years to work out a structure through experiments.
“This changes structural biology in many good ways, and not bad ways,” Adams said. “This only makes this a more exciting field to work in.”
However, it didn’t immediately result in all kinds of new drugs as some people had predicted — and researchers soon learned that the tool has its limitations. AlphaFold2 predictions aren’t perfect. They require experimental validation, Perrakis said. But “you can move much quicker to the actual study of the structures.” Now when his students start a new project, they first use AlphaFold2 to predict the structure of a particular protein. Then they conduct experiments to validate it.
Perrakis suspects that he and other researchers will continue to use X-ray crystallography to a degree. But to develop initial protein structures, many are starting to combine deep learning predictions with advanced electron microscope techniques such as cryo-EM, which involves flash-freezing biological samples and bombarding them with electrons. Then they can get to the interesting questions about what their proteins do. AlphaFold2 has “turbo-boosted” cryo-EM, AlQuraishi said.
Mark Belan for Quanta Magazine
That shift has already begun. In June 2022, a special issue of Science revealed the near-atomic structure of a human nuclear pore complex. This massive, complicated structure — built of 30 different proteins — had been a biological quandary for decades. The scientists used AlphaFold2 predictions to fill in gaps in the proteins’ structures left unsolved by cryo-EM.
Seeing that paper, in which other scientists used AlphaFold2 to make a biological breakthrough, was the “moment that I knew that [AlphaFold] really, really mattered,” Jumper said.
Discoveries like the nuclear pore complex dot the timeline of the last three years of protein science. Already, AlphaFold2 has predicted protein structures that have been used to study diseases and create new tools for drug delivery. “It’s been hugely helpful for us,” said Feng Zhang, a molecular biologist at the Broad Institute who used AlphaFold2 to engineer a molecular syringe to deliver drugs into human cells. Knowing a protein’s structure can also help develop drugs if researchers can identify molecules to latch onto a protein’s shape, for example, and change its behavior. While some studies have suggested that AlphaFold2 predictions aren’t as useful as experimental structures in this realm, others have shown that they work just as well. The full impact of AI tools on drug discovery is still unfolding.
Some biologists, however, are already looking beyond AlphaFold2’s use in discerning the structures and functions of known proteins and toward designing ones that don’t exist in nature — a technique pivotal for designing novel medicines.
The Next Frontier
Almost immediately after seeing Jumper’s talk at the 2020 CASP conference, Baker got back to work on his Rosetta algorithm. Google hadn’t yet shared AlphaFold2’s underlying source code. Still, “we started playing with some of the ideas that they introduced,” Baker said. On the same day that Google DeepMind published AlphaFold2 in Nature, he and his team announced RoseTTAFold, a highly accurate rival to AlphaFold. RoseTTAFold also uses deep learning to predict protein structures but has a very different underlying architecture than AlphaFold2.
“Once the scientific idea is out there, it’s possible for people, at least the ones who have enough resources, to reverse engineer it and try to build on top of that,” Tosatto said.
RoseTTAFold wasn’t alone. Other AlphaFold competitors, including Meta, crafted their own algorithms to address protein structure prediction or related problems. Some, including Townshend’s biotech startup Atomic AI, have expanded beyond proteins to use deep learning to understand RNA structures. However, in the realm of single-structure predictions, no one has been able to match AlphaFold’s accuracy so far, Thornton said. “I’m sure they will, but I think getting another … AlphaFold moment like that will be very difficult.”
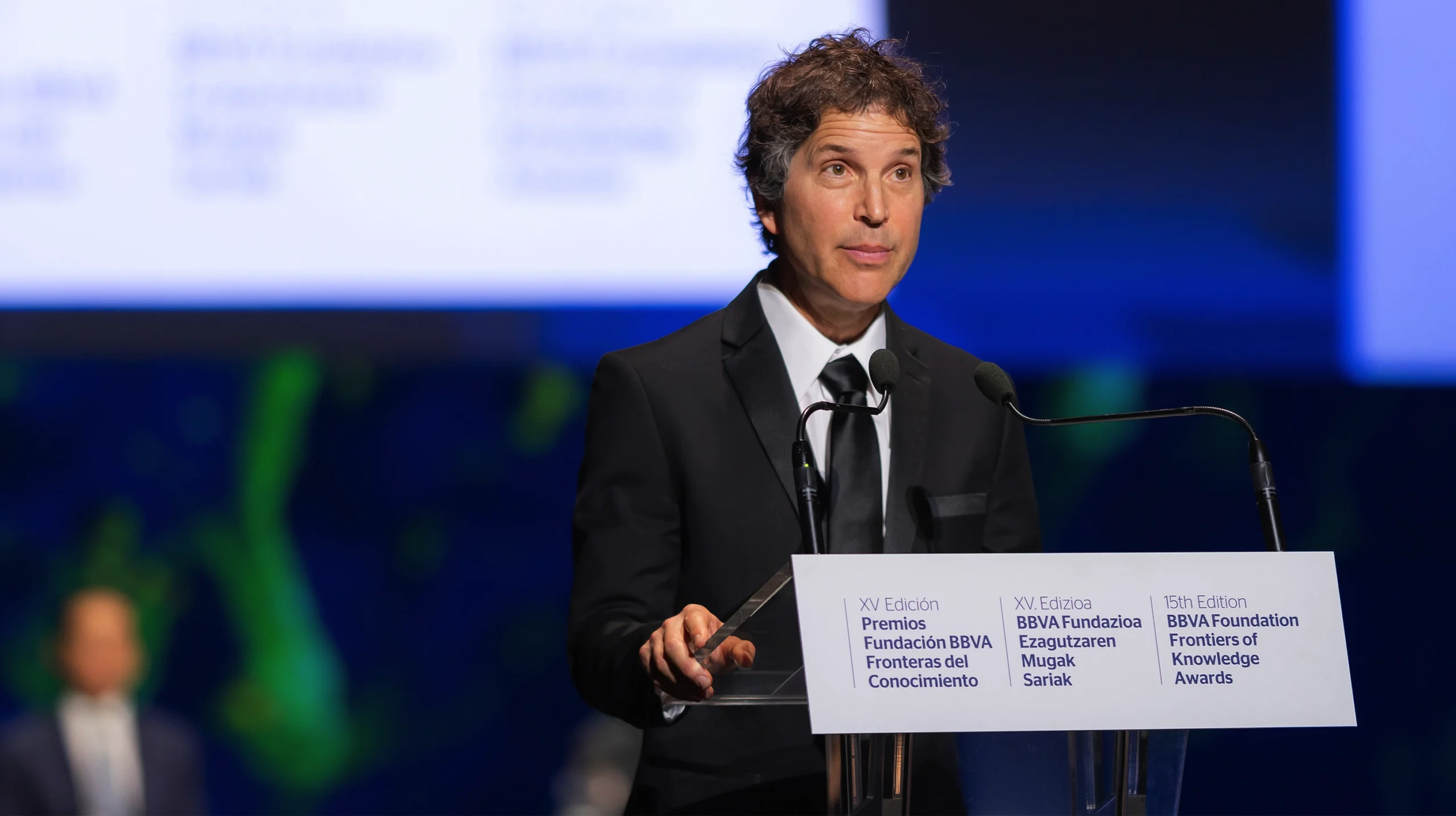
Last year, David Baker (pictured here), with John Jumper and Demis Hassabis, received the Frontiers of Knowledge Award in Biology and Biomedicine for their work revolutionizing the study and design of proteins with artificial intelligence.
BBVA Foundation
At least in public, Baker and Jumper have continued the tradition of productive competition established by CASP. “They might feel that I compete with them, but I feel like they’ve just been inspirational for us,” Baker said.
Jumper welcomes it. “It’s really important that people build on this science,” he said. “It would be sad to me if there was no intellectual lineage of AlphaFold.”
Baker is already evolving his program’s lineage to focus on a new frontier in protein science: protein design. Right now, biologists are confined to studying the proteins already invented by nature. Baker envisions a science in which they could design novel proteins — ones crafted specifically to harness sunlight, break down plastic, or form the basis of drugs or vaccines.
“The number of different types of protein structures or shapes in nature at the moment is quite limited,” said Danny Sahtoe, a structural biologist at the Hubrecht Institute in the Netherlands who did his postdoc under Baker. “In theory, more should be possible, and if you can have more shapes, that also means that you can have more functions.”
Protein design is essentially the “inverse protein folding problem,” said Baker, who directs the Institute for Protein Design at the University of Washington. Rather than feed an amino acid sequence to a deep learning algorithm and ask it to spit out a protein structure, a protein designer feeds a structure into an algorithm and asks it to spit out a sequence. Then, using that amino acid sequence, the designer builds the protein in the lab.
AlphaFold and RoseTTAFold by themselves can’t spit out these sequences; they are programmed to do the opposite. But Baker created a design-specific iteration of RoseTTAFold, known as RoseTTAFold diffusion or RF diffusion, based on its neural architecture.
The field of protein design has existed for a long time, but deep learning has accelerated it, Sahtoe said. It makes the process of designing realistic computer models of proteins “incredibly fast.” It used to take weeks or months for trained protein designers to create the backbone of a new protein. Now they can make one in days, sometimes even overnight.
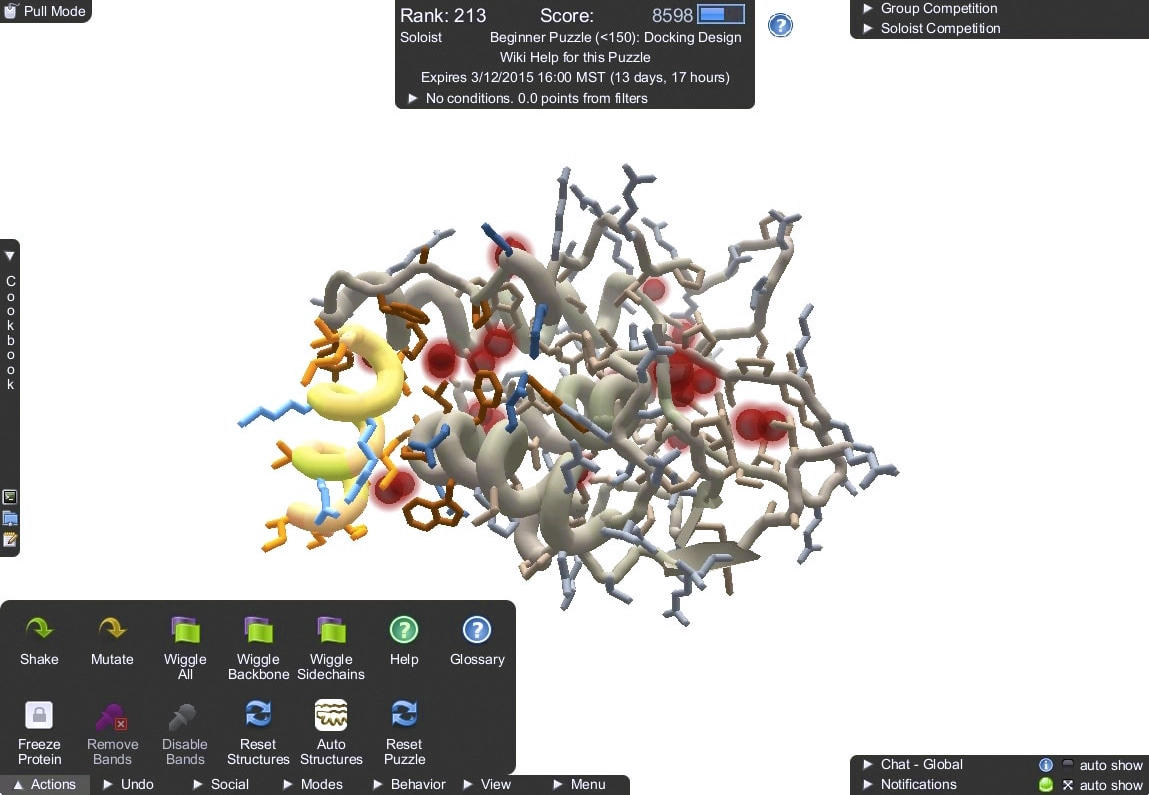
Foldit, an online game developed by Baker’s lab, has players predict protein structures.
Baker also updated Foldit to incorporate his obsession: Instead of building protein structures, players design proteins. It’s been productive. Baker’s lab has written papers on several of the player-designed proteins. One of the world’s top Foldit players is now a graduate student working with one of Baker’s colleagues at the University of Washington.
“Do we understand protein folding? Well, if we can design new sequences that fold up to new structures, then that shows we understand quite a lot about protein folding,” Baker said. “That, in a sense, you could view as a solution to the protein folding problem too.”
Trust Exercise
AlphaFold2’s success has undeniably shifted biologists’ attitudes toward artificial intelligence. For a long time, many experimental biologists distrusted computation. They understood that some machine learning approaches can make data appear better than it is. Then Google DeepMind demonstrated “unequivocally that you could do serious work with this,” AlQuraishi said. Any skepticism is now matched with: “Well, what about AlphaFold?”
“Biologists now believe our prediction results,” said Xu, the computational biologist who advanced convolutional networks. “Before, biologists always suspected if our prediction is reliable.”
Playing into this trust is a feature of the AlphaFold2 platform: It not only generates a 3D model of a protein, but also self-assesses the accuracy of its prediction by grading different parts of the structure on a confidence scale from zero to 100.
In July 2022, after Google DeepMind released the structure predictions of 218 million proteins — nearly all those known in the world — Adams decided to analyze AlphaFold2’s self-reports. He compared the predictions to the proteins’ solved structures and independently assessed their accuracy.
The “good news is that when AlphaFold thinks that it’s right, it often is very right,” Adams said. “When it thinks it’s not right, it generally isn’t.” However, in about 10% of the instances in which AlphaFold2 was “very confident” about its prediction (a score of at least 90 out of 100 on the confidence scale), it shouldn’t have been, he reported: The predictions didn’t match what was seen experimentally.
That the AI system seems to have some self-skepticism may inspire an overreliance on its conclusions. Most biologists see AlphaFold2 for what it is: a prediction tool. But others are taking it too far. Some cell biologists and biochemists who used to work with structural biologists have replaced them with AlphaFold2 — and take its predictions as truth. Sometimes scientists publish papers featuring protein structures that, to any structural biologist, are obviously incorrect, Perrakis said. “And they say: ‘Well, that’s the AlphaFold structure.’”
“Some people are overconfident — like, way overconfident — in what these deep learning models can do,” said Lauren Porter, an investigator at the National Institutes of Health. “We should use these deep learning models for as much as we can, but we also need to approach them with caution and humility.”
Jones has heard of scientists struggling to get funding to determine structures computationally. “The general perception is that DeepMind did it, you know, and why are you still doing it?” Jones said. But that work is still necessary, he argues, because AlphaFold2 is fallible.
“There are very large gaps,” Jones said. “There are things that it can’t do quite clearly.”
While AlphaFold2 is excellent at predicting the structures of small, simple proteins, it’s less accurate at predicting those containing multiple parts. It also can’t account for the protein’s environment or bonds with other molecules, which alter a protein’s shape in the wild. Sometimes a protein needs to be surrounded by certain ions, salts or metals to fold properly.
“At the moment, AlphaFold is a little bit of a ways away from being able to determine context,” Walden said. Her group has determined several structures experimentally that AlphaFold2 couldn’t predict.
There are also several types of dynamic proteins that AlphaFold2 predicts poorly but that are no less important in function. Shape-shifting proteins, also known as fold-switching proteins, are not static: Their shapes change as they interact with other molecules. Some fold into dramatically different shapes, despite having the same amino acid sequence. Fold-switching proteins “challenge the paradigm that sequences encode one structure,” Porter said, “because clearly they don’t.”
Compared to the hundreds of thousands of static, single-structure proteins that the DeepMind algorithm trained on, there are only about 100 examples of fold-switching proteins — although more surely exist. It’s perhaps no surprise, Porter said, that “generally speaking, these algorithms were made to predict a single fold.”
And then there are the proteins that flail about like an air dancer outside a car dealership. Intrinsically disordered proteins or protein regions lack a stable structure. They wiggle and re-form constantly. “They’ve been in many ways ignored simply because they were a little bit annoying,” said Kresten Lindorff-Larsen, a professor of computational protein biophysics at the University of Copenhagen. Around 44% of human proteins have a disordered region made up of at least 30 amino acids. “It’s a relatively large fraction of them,” Lindorff-Larsen said.
AlphaFold2 can predict when a region is likely to be intrinsically disordered — but it can’t tell you what that disorder looks like.
For his part, Jumper’s biggest frustration is that AlphaFold2 doesn’t register the difference between two proteins that vary by a single amino acid, known as a point mutation. Point mutations can “have quite dramatic effects, sometimes on structure and often on function of proteins,” he said. “AlphaFold is relatively blind” to them, in that it will produce the same structure for both sequences.
In September 2023 DeepMind released AlphaMissense, a deep learning algorithm that predicts the effects of such mutations. It can’t show the change to the structure, but it informs the user if the mutation might turn the protein pathogenic or introduce dysfunction based on similar mutations in known pathogenic proteins.
However, even if AlphaFold2 could predict all proteins perfectly, it would be far from modeling biological reality. That’s because in a cell, proteins never act alone.
Cellular Complexity
The insides of cells are complicated and chaotic. A cell’s external membrane envelops a biochemical environment densely crowded with molecular parts — proteins, signaling molecules, messenger RNA, organelles and more. Proteins bind to each other and to other molecules, which alters their forms and functions.
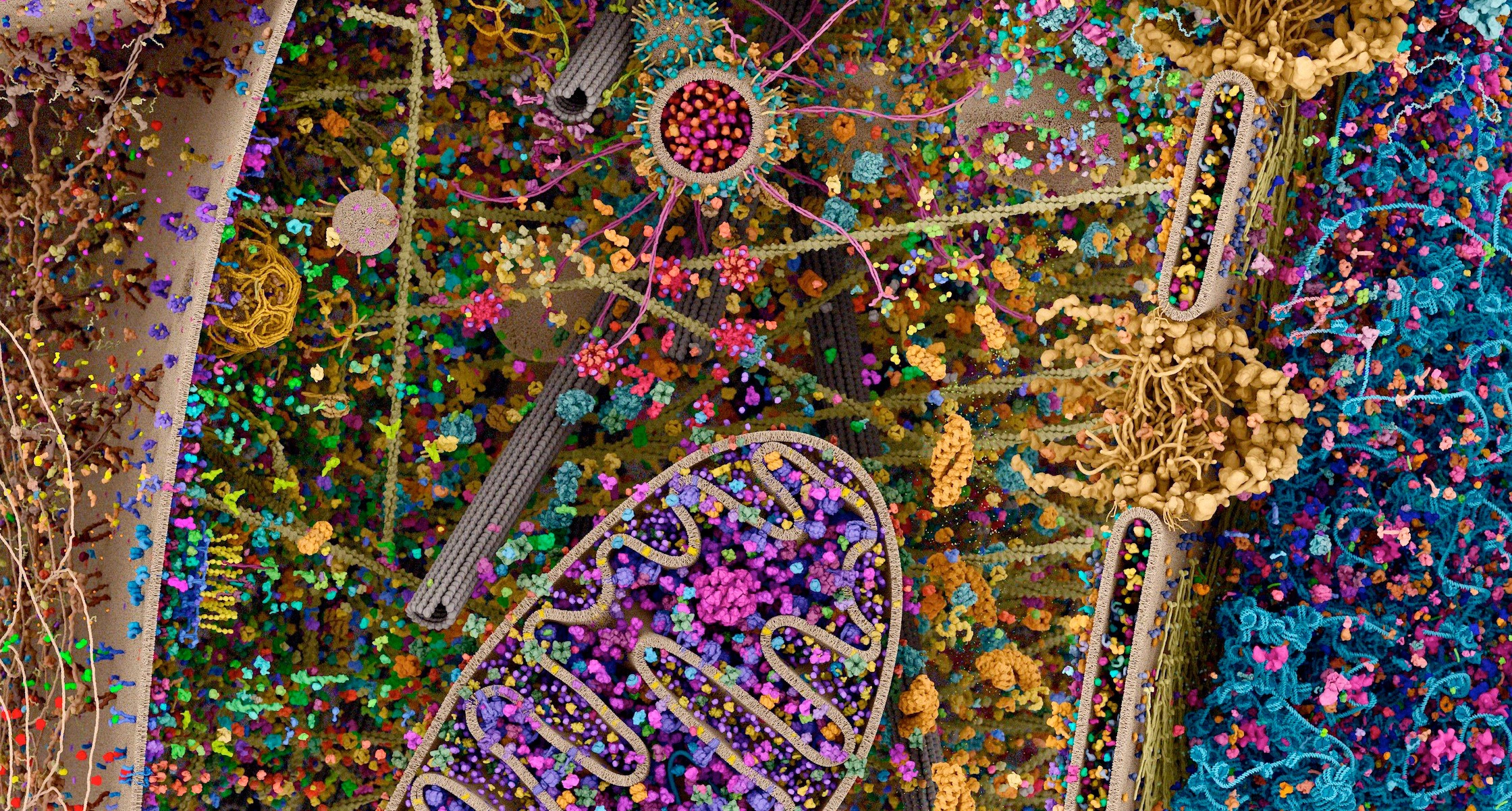
Proteins don’t work alone: They interact constantly with other molecules. This rendering of a cellular landscape is made of models of real proteins and other molecules in “an attempt to visualize the great complexity and beauty of the cell’s molecular choreography,” wrote its creator, Gael McGill.
Evan Ingersoll and Gaël McGill, PhD/Digizyme Inc
AlphaFold2’s ability to predict the structure of a single protein doesn’t get biologists close to understanding proteins in this intricate native environment. But that’s the direction the field is now heading. Protein science’s artificial intelligence giants, Google DeepMind and David Baker’s Institute for Protein Design, are now evolving their deep learning algorithms to predict the structures that proteins assume while interacting with other molecules.
In spring 2024, they both published papers describing similar developments in this area. Updates to their algorithms — launched with the new names AlphaFold3 and RoseTTAFold All-Atom — enable them to predict the structures of proteins bound to each other, DNA, RNA and other small molecules.
Biologists are just starting to test out these updates. So far, AlphaFold3 is much more accurate than RoseTTAFold All-Atom, AlQuraishi said — but it’s not as big of a leap as an “AlphaFold2 moment.” For some macromolecules, such as RNA structures, its accuracy remains below that of other physics-based systems and experiments.
Even so, the new algorithms are a step in the right direction. The interactions between proteins and other molecules are critical to their functioning in cells. To develop drugs that can dock onto proteins and alter their activity as desired, researchers need to understand what those complexes look like. It’s unlikely, though, that either algorithm will lead to new medicines anytime soon, Adams said. “Both methods are still limited in their accuracy, [but] both are dramatic improvements on what was possible.”
There is one other major change in DeepMind’s new product. AlphaFold2’s underlying code was open-source so that other researchers could study the algorithm and remake it for their own projects. However, rather than share AlphaFold3’s source code, Google has so far opted to protect it as a trade secret. “For the time being, at least, no one can run and use it like they did with [AlphaFold2],” AlQuraishi said.
Even before the release of AlphaFold3, researchers had been testing AlphaFold2 to see if it could provide useful information on proteins in different conformations. Brenda Rubenstein, an associate professor of chemistry and physics at Brown University, was interested in kinases, a type of protein that activates other proteins. Specifically, she wanted to understand the mechanism of a kinase that causes cancer so that she could develop more precise drugs against it. Her lab modeled the kinase’s structure using a physics-based approach, which maps the 3D coordinates of atoms using Newton’s laws. It took two and a half years.
“About a year ago, we said: Can we do this faster?” Rubenstein said. They tried using AlphaFold2 in a novel way. By feeding data about related proteins to the algorithm, she found that it could predict her kinase in different conformations with more than 80% accuracy.
Rubenstein’s is one of several labs finding that “if you poke AlphaFold in the right way, you get it to kind of spit out alternate conformations,” AlQuraishi said. “That’s been encouraging.”
AlQuraishi hopes that by 2040, deep learning will be able to simulate an entire cell and all the structures and dynamics within it. Getting there, however, will require leaps on both the experimental and computational sides.
An Outsider’s Take
For many biologists, AlphaFold2 was the breakthrough they had been waiting for. The goal of CASP had been to create computing tools that predict protein structure from sequence. Still, many can’t help but ask: Why was a relative newcomer able to crack the protein code when so many experts had struggled for decades?
The insights that Google DeepMind’s team of computer and protein scientists brought to the problem are undeniable. At the same time, the ground of protein science was fertile and ready to yield a deep learning revolution, AlQuraishi said. “These things don’t appear out of nowhere.”
By the time CASP 2020 came around, many researchers expected a breakthrough in structure prediction to come through artificial intelligence. “It was all heading in that direction,” Townshend said. But they didn’t expect it to come from a multibillion-dollar technology company, and they didn’t expect it so soon. Some said AlphaFold2 wasn’t a feat of new science but rather clever engineering. Some were surprised that David Baker’s algorithms didn’t take the trophy. Others were less surprised because of Google DeepMind’s unmatched resources.
Around 100 labs participate in CASP every year, and though they had begun to adopt AI technologies, they “probably didn’t have the expertise that DeepMind had, nor the computing power,” Thornton said. DeepMind “had access to basically unlimited computing power.”
She also speculated that Google’s lack of expertise in protein science may have freed them creatively. “They were single-minded,” Thornton said, and focused on building a great neural network. Protein biologists had baggage. As they worked on their AI tools, they wanted to capture the atomic-level molecular physics and chemistry involved in protein folding. DeepMind had a different approach: We will transform sequence data into a 3D structure, and it doesn’t matter how we get there.
“Rather than trying to solve the protein folding problem, which I think a lot of previous predictions tried to do, they actually just went with the brute force” of mapping out the atoms’ final positions in space, Walden said. “Rather interestingly, they have therefore probably solved the problem.”
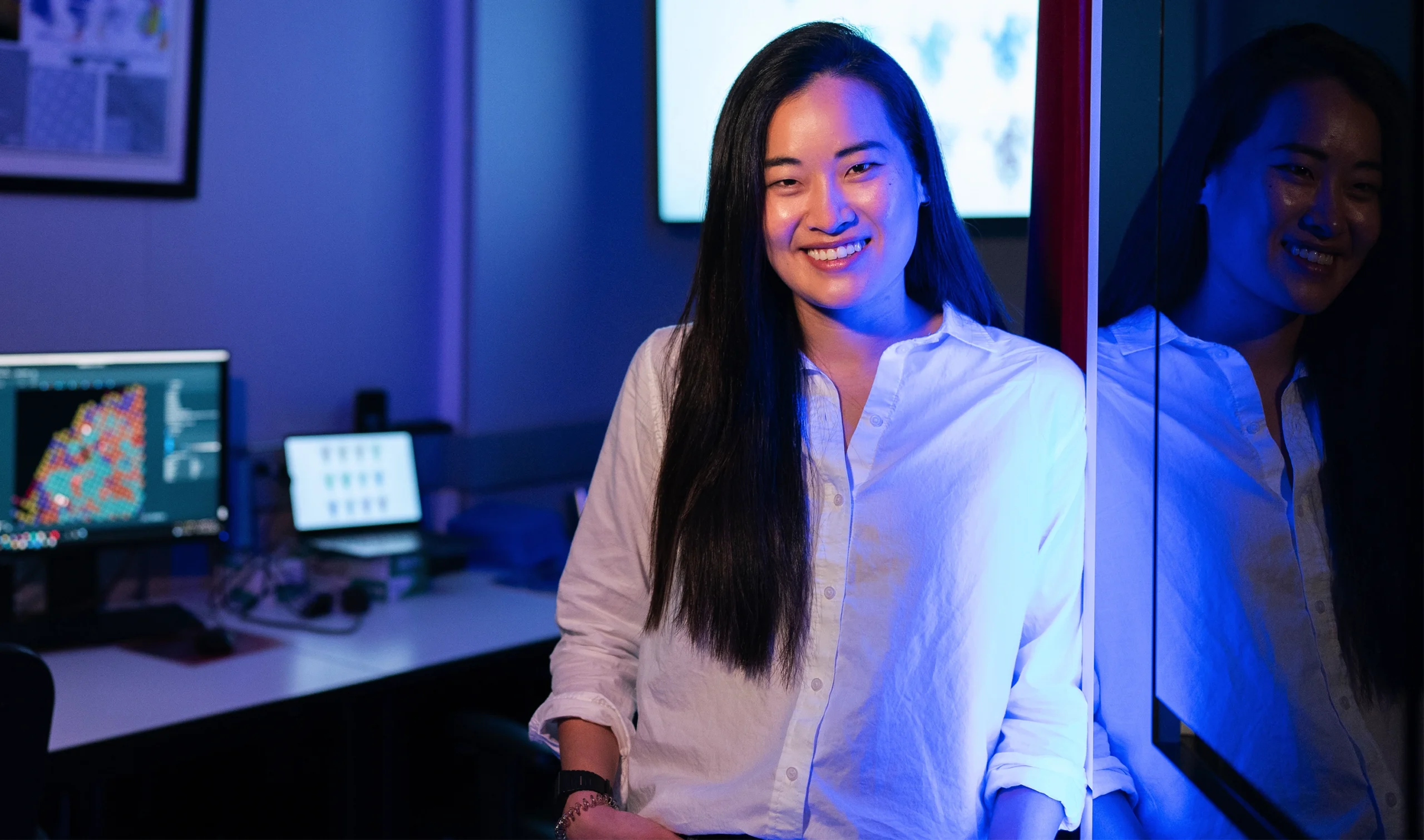
The Princeton University computer scientist Ellen Zhong, who was an intern on Google DeepMind’s AlphaFold2 team in 2021, pioneered the use of deep learning in combination with cryo-EM to study protein dynamics.
Tori Repp/Fotobuddy for Princeton University
To some biologists, that approach leaves the protein folding problem incomplete. From the earliest days of structural biology, researchers hoped to learn the rules of how an amino acid string folds into a protein. With AlphaFold2, most biologists agree that the structure prediction problem is solved. However, the protein folding problem is not. “Right now, you just have this black box that can somehow tell you the folded states, but not actually how you get there,” Zhong said.
“It’s not solved the way a scientist would solve it,” said Littman, the Brown University computer scientist.
This might sound like “semantic quibbling,” said George Rose, the biophysics professor emeritus at Johns Hopkins. “But of course it isn’t.” AlphaFold2 can recognize patterns in how a given amino acid sequence might fold up based on its analysis of hundreds of thousands of protein structures. But it can’t tell scientists anything about the protein folding process.
“For many people, you don’t need to know. They don’t care,” Rose said. “But science, at least for the past 500 years or so … has been involved with trying to understand the process by which things occur.” To understand the dynamics, mechanisms, functions and nature of protein-based life, Rose argued, you need the full story — one that deep learning algorithms can’t tell us.
To Moult, it doesn’t matter that the machine does something he doesn’t understand. “We’re all used to machines doing things we can’t. You know, I can’t run as fast as my car,” he said. To molecular biologists who are trying to study a protein and just need to know roughly what it looks like, how they get there doesn’t really matter.
But “until we know really how it works, we’re never going to have a 100% reliable predictor,” Porter said. “We have to understand the fundamental physics to be able to make the most informed predictions we can.”
“We keep moving the goalpost,” AlQuraishi said. “I do think that core problem has been solved, so now it’s very much about what comes next.”
Even as biologists continue to debate these topics, others are looking forward to a field that’s undeniably changed — and backward toward its recent past.
Sometimes Perrakis is hit by a wave of nostalgia for the old ways of doing things. In 2022, his team described an enzyme involved in modifying microtubules (giant, rod-shaped molecules that provide structures to cells) that they had determined using X-ray crystallography. “I realized that I’m never going to do that [again],” he said. “Having the first structure appearing after months of work was a very particular satisfaction.”
AlphaFold2 hasn’t made those experiments obsolete. On the contrary, it’s illuminated just how necessary they are. It has stitched together two historically disparate disciplines, launching a new and stimulating conversation.
The New World
Seventy years ago, proteins were thought to be a gelatinous substance, Porter said. “Now look at what we can see”: structure after structure of a vast world of proteins, whether they exist in nature or were designed.
The field of protein biology is “more exciting right now than it was before AlphaFold,” Perrakis said. The excitement comes from the promise of reviving structure-based drug discovery, the acceleration in creating hypotheses and the hope of understanding complex interactions happening within cells.
“It [feels] like the genomics revolution,” AlQuraishi said. There is so much data, and biologists, whether in their wet labs or in front of their computers, are just starting to figure out what to do with it all.
But like other artificial intelligence breakthroughs sparking across the world, this one might have a ceiling.
AlphaFold2’s success was founded on the availability of training data — hundreds of thousands of protein structures meticulously determined by the hands of patient experimentalists. While AlphaFold3 and related algorithms have shown some success in determining the structures of molecular compounds, their accuracy lags behind that of their single-protein predecessors. That’s in part because there is significantly less training data available.
The protein folding problem was “almost a perfect example for an AI solution,” Thornton said, because the algorithm could train on hundreds of thousands of protein structures collected in a uniform way. However, the Protein Data Bank may be an unusual example of organized data sharing in biology. Without high-quality data to train algorithms, they won’t make accurate predictions.
“We got lucky,” Jumper said. “We met the problem at the time it was ready to be solved.”
No one knows if deep learning’s success at addressing the protein folding problem will carry over to other fields of science, or even other areas of biology. But some, like AlQuraishi, are optimistic. “Protein folding is really just the tip of the iceberg,” he said. Chemists, for example, need to perform computationally expensive calculations. With deep learning, these calculations are already being computed up to a million times faster than before, AlQuraishi said.
Artificial intelligence can clearly advance specific kinds of scientific questions. But it may get scientists only so far in advancing knowledge. “Historically, science has been about understanding nature,” AlQuraishi said — the processes that underlie life and the universe. If science moves forward with deep learning tools that reveal solutions and no process, is it really science?
“If you can cure cancer, do you care about how it really works?” AlQuraishi said. “It is a question that we’re going to wrestle with for years to come.”
If many researchers decide to give up on understanding nature’s processes, then artificial intelligence will not just have changed science — it will have changed the scientists too.
Meanwhile, the CASP organizers are wrestling with a different question: how to continue their competition and conference. AlphaFold2 is a product of CASP, and it solved the main problem the conference was organized to address. “It was a big shock for us in terms of: Just what is CASP anymore?” Moult said.
In 2022, the CASP meeting was held in Antalya, Turkey. Google DeepMind didn’t enter, but the team’s presence was felt. “It was more or less just people using AlphaFold,” Jones said. In that sense, he said, Google won anyway.
Some researchers are now less keen on attending. “Once I saw that result, I switched my research,” Xu said. Others continue to hone their algorithms. Jones still dabbles in structure prediction, but it’s more of a hobby for him now. Others, like AlQuraishi and Baker, continue on by developing new algorithms for structure prediction and design, undaunted by the prospect of competing against a multibillion-dollar company.
Moult and the conference organizers are trying to evolve. The next round of CASP opened for entries in May. He is hoping that deep learning will conquer more areas of structural biology, like RNA or biomolecular complexes. “This method worked on this one problem,” Moult said. “There are lots of other related problems in structural biology.”
The next meeting will be held in December 2024 by the aqua waters of the Caribbean Sea. The winds are cordial, as the conversation will probably be. The stamping has long since died down — at least out loud. What this year’s competition will look like is anyone’s guess. But if the past few CASPs are any indication, Moult knows to expect only one thing: “surprises.”
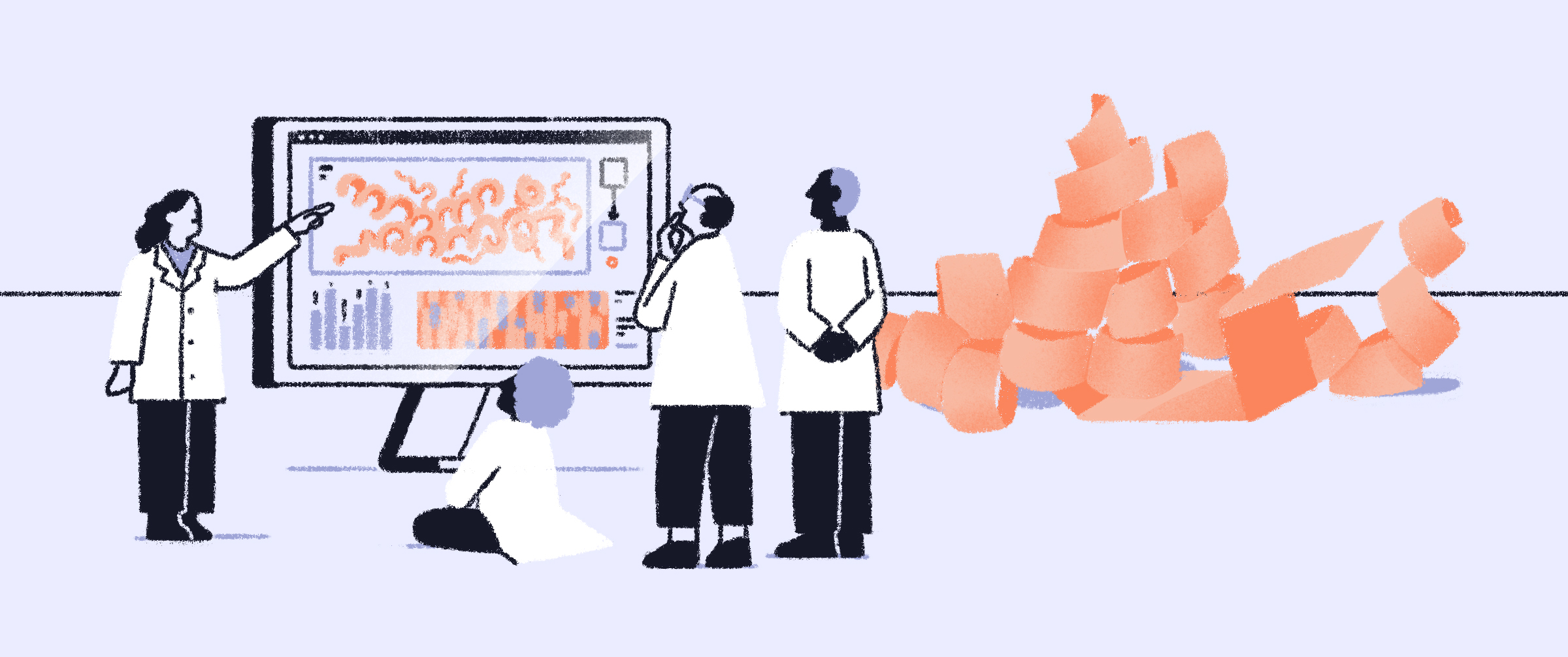