New AI Tools Predict How Life’s Building Blocks Assemble
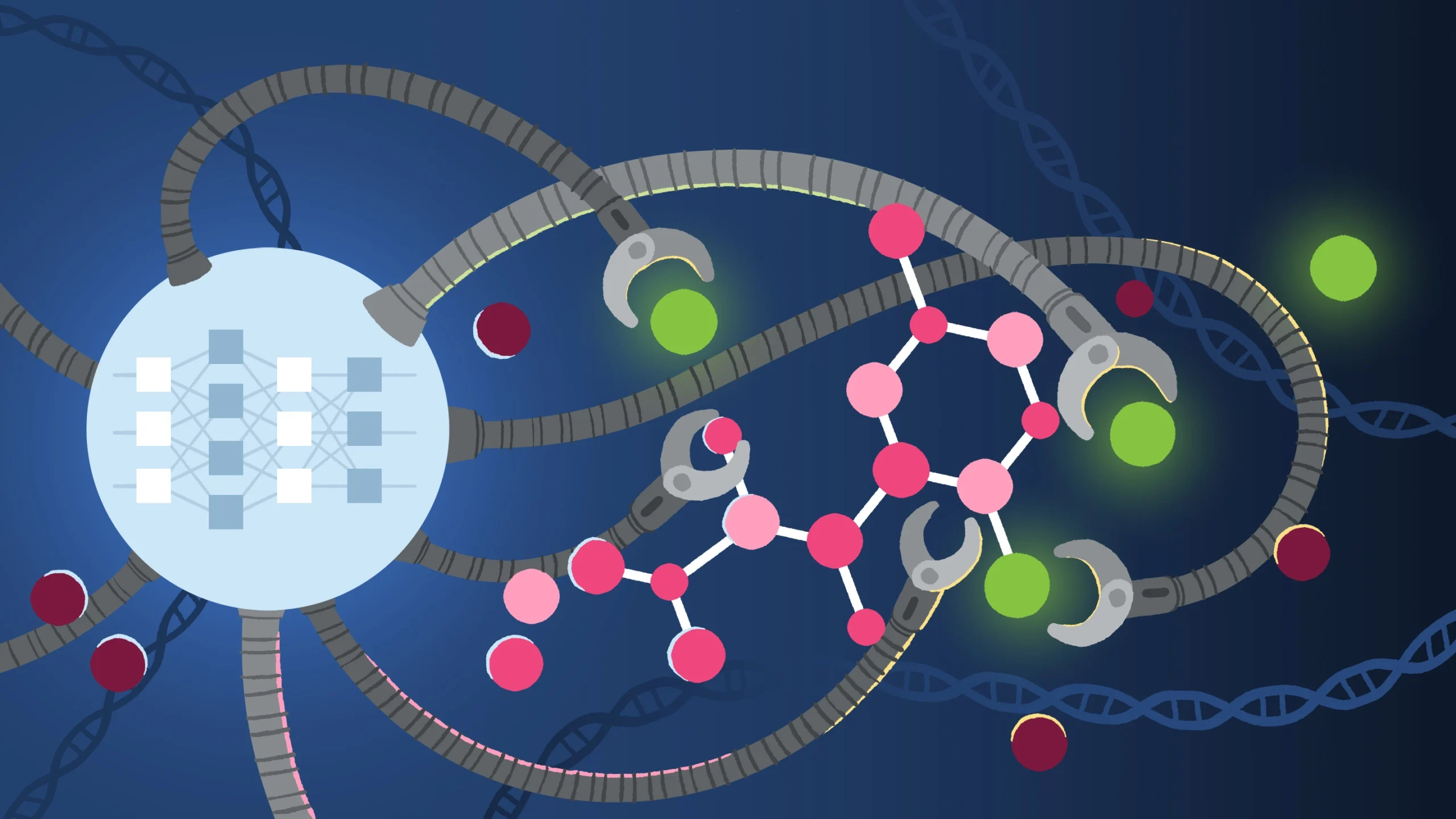
New AI systems can predict the shapes and structures of not only proteins, but also the complexes they form with DNA, RNA and other biomolecules.
Adam Nickel for Quanta Magazine
Introduction
Proteins are the molecular machines that sustain every cell and organism, and knowing what they look like will be critical to untangling how they function normally and malfunction in disease. Now researchers have taken a huge stride toward that goal with the development of new machine learning algorithms that can predict the folded shapes of not only proteins but other biomolecules with unprecedented accuracy.
In a paper published today in Nature, Google DeepMind and its spinoff company Isomorphic Labs announced the latest iteration of their AlphaFold program, AlphaFold3, which can predict the structures of proteins, DNA, RNA, ligands and other biomolecules, either alone or bound together in different embraces. The findings follow the tail of a similar update to another deep learning structure-prediction algorithm, called RoseTTAFold All-Atom, which was published in March in Science.
While the previous versions of these algorithms could predict protein structures — a remarkable achievement in itself — they didn’t go far enough to dispel the mysteries of biological processes because proteins rarely act alone. “Every time I would give an AlphaFold2 talk, I could almost guess what the questions were going to be,” said John Jumper, who leads the AlphaFold team at Google DeepMind. “Someone was going to raise their hand and say, ‘Yes, but my protein interacts with DNA. Can you tell me how?’” Jumper would have to admit that AlphaFold2 didn’t know the answer.
But AlphaFold3 might. Along with other emerging deep learning algorithms, it goes beyond proteins to a more challenging, and more relevant, biological landscape that includes the vast diversity of molecules interacting in cells.
“Now you’re getting at all the complex interactions that matter in biology,” said Brenda Rubenstein, an associate professor of chemistry and physics at Brown University who was not involved with either study. “You’re starting to get more of the bigger picture.”
Understanding those interactions is “fundamental to biological function,” said Paul Adams, a molecular biophysicist at Lawrence Berkeley National Laboratory who was also not involved in either study. “Both groups have made significant progress in addressing [this].”
Both algorithms have limitations, but they have the potential to evolve into even more powerful prediction tools. In the coming months, scientists will begin to test them, and in doing so they will reveal how useful these algorithms might be.
AI Advances in Biology
Deep learning is a flavor of machine learning that’s loosely inspired by the human brain. These computer algorithms are built using complex networks of informational nodes (called neurons) that form layered connections with one another. Researchers provide the deep learning network with training data, which the algorithm uses to adjust the relative strengths of connections between neurons to produce outputs that get ever closer to training examples. In the case of protein artificial intelligence systems, this process leads the network to produce better predictions of proteins’ shapes based on their amino-acid sequence data.
AlphaFold2, released in 2021, was a breakthrough for deep learning in biology. It unlocked an immense world of previously unknown protein structures, and has already become a useful tool for researchers working to understand everything from cellular structures to tuberculosis. It has also inspired the development of additional biological deep learning tools. Most notably, the biochemist David Baker and his team at the University of Washington in 2021 developed a competing algorithm called RoseTTAFold, which like AlphaFold2 predicts protein structures from sequence data.
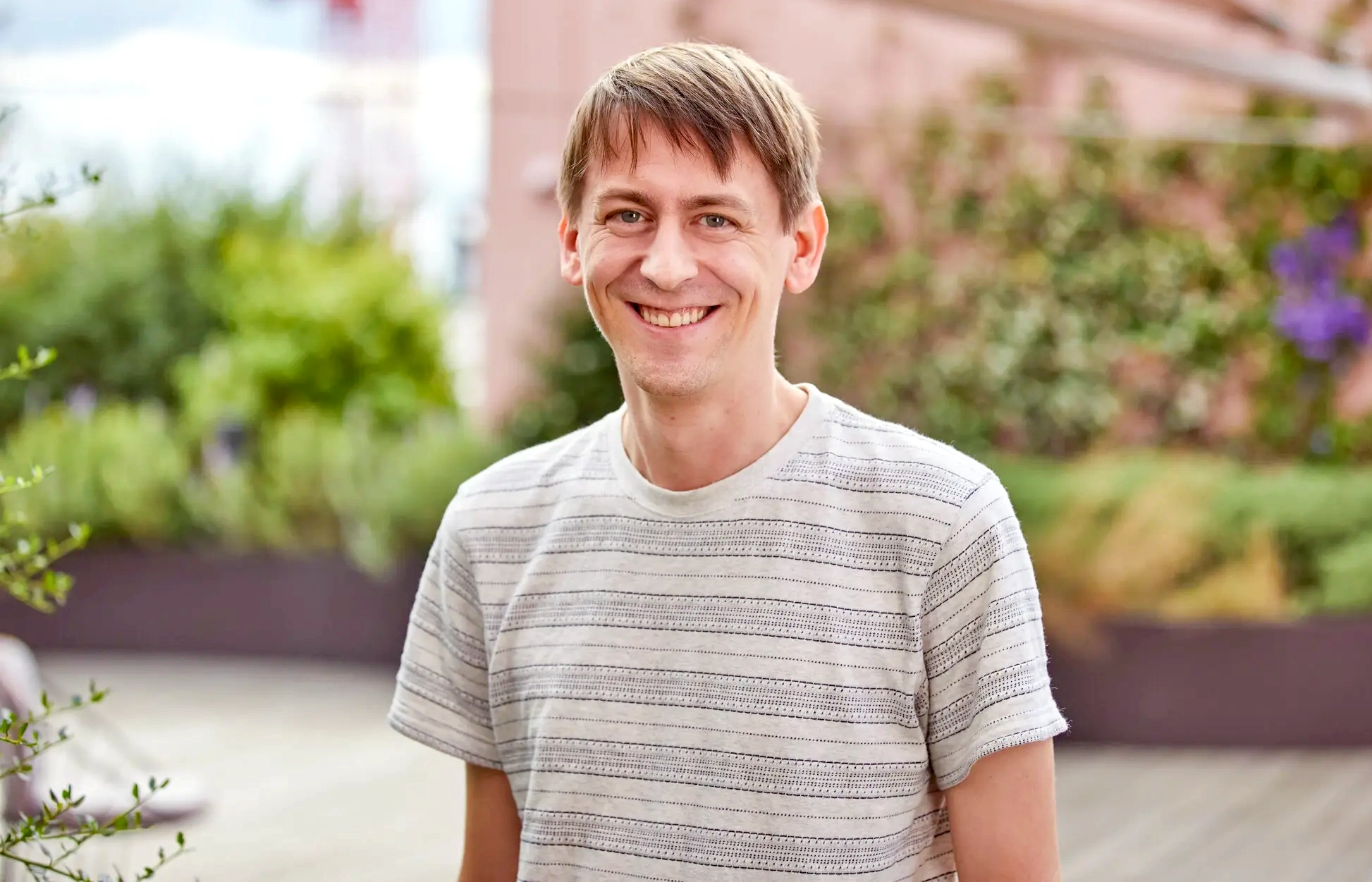
John Jumper, who joined Google DeepMind in 2017, led the team that produced AlphaFold3, which can predict the structures of biomolecular complexes. “I can’t wait to see what [researchers] do with AlphaFold3,” he said.
Google DeepMind
Since then, both algorithms have been updated with new features. RoseTTAFold Diffusion could be used to design new proteins that don’t exist in nature. AlphaFold Multimer could look at the interaction of multiple proteins. “But what we left unanswered,” Jumper said, “was: How do proteins talk to the rest of the cell?”
The success of the first iterations of protein-predicting deep learning algorithms rested on the availability of good training data: around 140,000 validated protein structures that had been deposited over 50 years into the Protein Data Bank. Increasingly, biologists have also deposited the structures of small molecules, DNA, RNA and their combinations. In this expansion of AlphaFold’s algorithm to include more biomolecules, “the biggest unknown,” Jumper said, was whether there’d be enough data to enable the algorithm to accurately predict complexes of proteins with these other molecules.
Apparently there was. At the end of 2023, Baker and then Jumper released the preliminary versions of their new AI tools, and since then they have subjected their algorithms to peer review.
Both AI systems address the same question, but the underlying architectures of their deep learning methods differ, said Mohammed AlQuraishi, a systems biologist at Columbia University who is not involved in either system. Jumper’s team used a process called diffusion — the technology that powers most non-text-based generative AI systems, such as Midjourney and DALL·E, which generate art based on text prompts, AlQuraishi said. Instead of predicting the molecular structure directly and then improving it, this type of model first produces a blurry image and refines it in an iterative fashion.
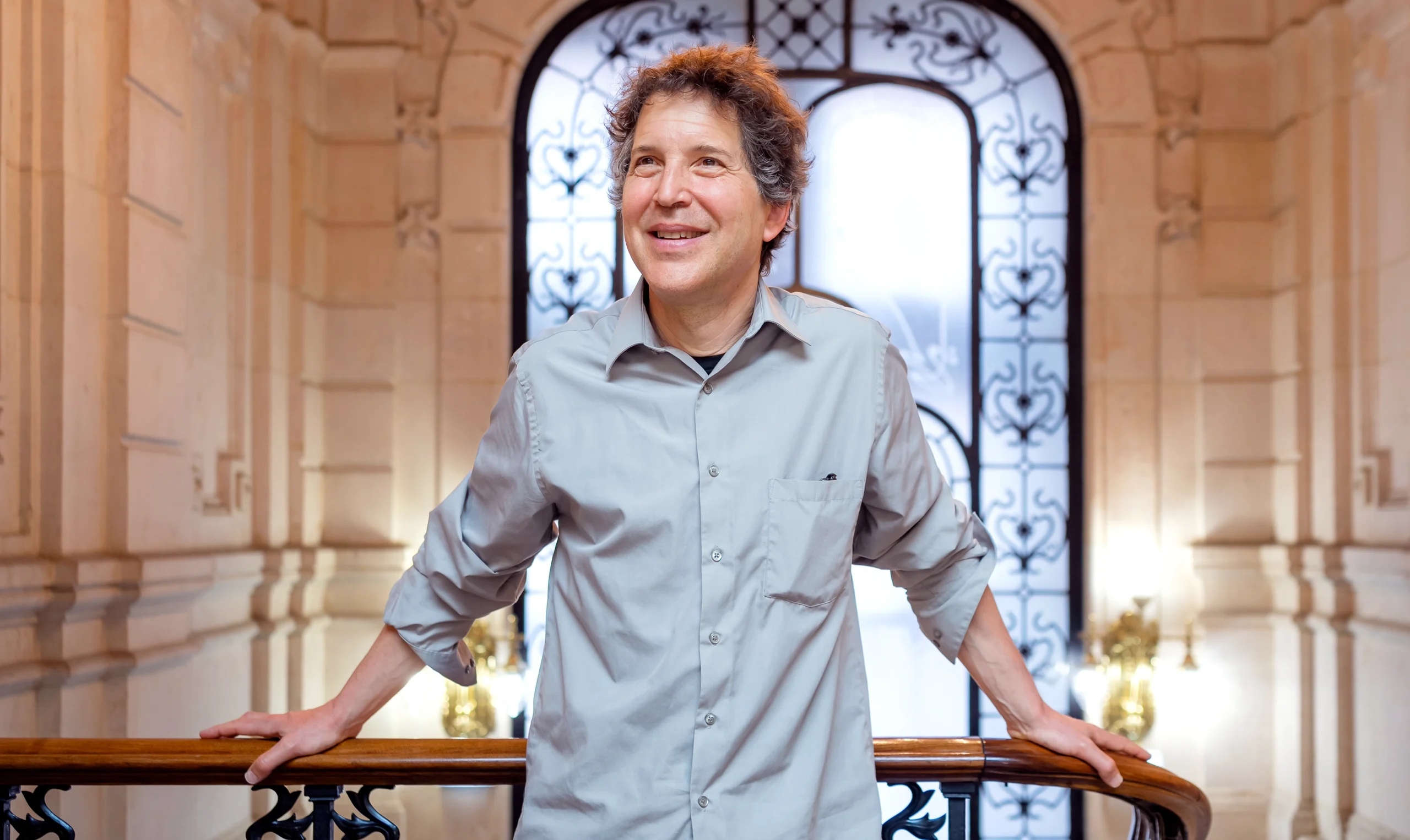
The biochemist David Baker has spearheaded the development of RoseTTAFold, a leading protein-prediction AI system. He released an update that models biomolecular complexes just a couple of months before Google DeepMind released theirs.
BBVA Foundation
From a technical standpoint, there’s not a huge jump from RoseTTAFold to RoseTTAFold All-Atom, AlQuraishi said. Baker didn’t massively change the underlying architecture of RoseTTAFold, but updated it to include known rules of biochemical interactions. The algorithm doesn’t use diffusion to predict biomolecular structures. However, Baker’s AI for designing proteins does. The latest iteration of this program, known as RoseTTAFold Diffusion All-Atom, can design new biomolecules in addition to proteins.
“The kind of dividends that could come from being able to apply generative AI technologies to biomolecules is only partially realized with protein design,” AlQuraishi said. “If we’re able to do as well with small molecules, that would be kind of amazing.”
Sizing Up the Competition
Side by side, AlphaFold3 appears to be more accurate than RoseTTAFold All-Atom. For example, in their analysis in Nature, the Google team found that their tool is about 76% accurate in predicting structures of proteins interacting with small molecules called ligands, compared to about 42% accuracy for RoseTTAFold All-Atom and 52% for the best alternative tools out there.
AlphaFold3’s structure-prediction performance is “very impressive,” Baker said, “and better than that of RoseTTAFold All-Atom.”
However, those testing figures are based on a limited data set that is not very challenging, AlQuraishi said. He doesn’t expect all protein-complex predictions to score so highly. And certainly the new AI tools aren’t yet powerful enough to support a robust drug-discovery program on their own, since that requires researchers to understand complex biomolecular interactions. Still, “it’s definitely promising,” he said, and meaningfully better than what existed previously.
Adams agrees. “If anybody’s going to claim that they can use this tomorrow to accurately develop drugs, I don’t buy that,” he said. “Both methods are still limited in their accuracy, [but] both are dramatic improvements on what was possible.”
They’ll be especially useful for creating rough predictions that can then be tested out computationally or experimentally. The biochemist Frank Uhlmann had the opportunity to pretest AlphaFold3 after running into a Google employee in a hallway of the Francis Crick Institute in London, where he works. He decided to look up a protein-DNA interaction that has been “really puzzling for us,” he said. AlphaFold3 spit out a prediction that they’re now experimentally testing in the lab. “We already got some new ideas that really might work,” Uhlmann said. “It’s an amazing discovery tool.”
Still, there is much to improve upon. When RoseTTAFold All-Atom predicts the structures of complexes of proteins and small molecules, it sometimes places the molecules in the correct pocket in a protein but not in the correct orientation. AlphaFold3 sometimes incorrectly predicts a molecule’s chirality — the distinct “left-handed” or “right-handed” geometric orientation of its structure. Occasionally it will hallucinate or create inaccurate structures.
And both algorithms still produce static images of proteins and their complexes. In a cell, proteins are dynamic and can change depending on their environment: They move around, rotate and go through different conformations. It will be challenging to address this, Adams said, mainly due to a lack of training data. “It would be great to have some concerted efforts to collect experimental data designed to inform these challenges,” he said.
One major change in Google’s new product is that it will not be open-source. When the team released AlphaFold2, they published the underlying code, which allowed biologists to reproduce and play with the algorithm in their own labs. But AlphaFold3’s code will not be publicly available.
“They do appear to describe the method in detail. But for the time being, at least, no one can run and use it like they did with [AlphaFold2],” AlQuraishi said. That is “a big step back. We will, of course, try to reproduce it.”
Google did, however, announce that they are taking steps to make the product accessible by offering a new AlphaFold server to biologists running AlphaFold3. Predicting biomolecular structures takes a ton of computing power: Even at a lab institute like Francis Crick, which hosts high-performing computing clusters, it takes about a week to spit out a result, Uhlmann said. Google’s more powerful servers, by comparison, can make a prediction in 10 minutes, he said, and scientists around the world will be able to use them. “It’s going to completely democratize protein-prediction research,” Uhlmann said.
The true impact of these tools won’t be known for months or years, as biologists begin to test and use them in research. And they will continue to evolve. What’s next for deep learning in molecular biology is “going up the biological complexity ladder,” Baker said, beyond even the biomolecule complexes predicted by AlphaFold3 and RoseTTAFold All-Atom. But if the history of protein-structure AI can predict the future, then these next-generation deep learning models will continue to help scientists reveal the complex interactions that make life happen.
“There’s so much more to be understood,” Jumper said. “It’s the beginning.”