New Turmoil Over Predicting the Effects of Genes
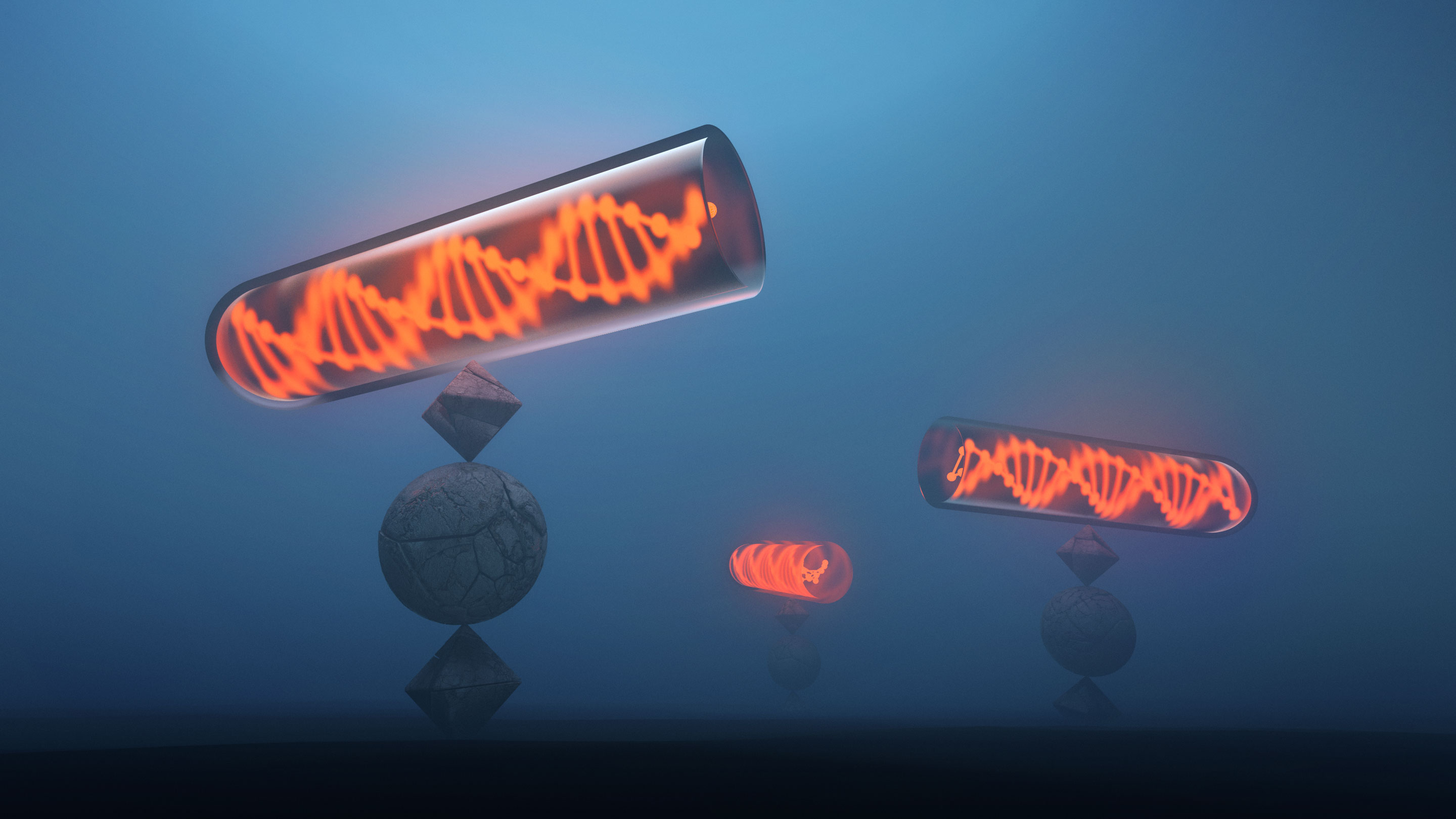
Olena Shmahalo/Quanta Magazine
Introduction
Various innovations in the field of genomics over the past few decades have given researchers hope that resolutions to long-lasting debates might finally be on the horizon. In particular, many have become optimistic about the prospects for disentangling the threads of “nature” and “nurture” — that is, about determining the extent to which genes alone can explain differences within and between populations.
But two recent studies are now calling some of the methods underlying those aspirations into question.
A key breakthrough was the recent development of genome-wide association studies (GWAS, commonly pronounced “gee-wahs”). The genetics of simple traits can often be deduced from pedigrees, and people have been using that approach for millennia to selectively breed vegetables that taste better and cows that produce more milk. But many traits are not the result of a handful of genes that have clear, strong effects; rather, they are the product of tens of thousands of weaker genetic signals, often found in noncoding DNA. When it comes to those kinds of features — the ones that scientists are most interested in, from height, to blood pressure, to predispositions for schizophrenia — a problem arises. Although environmental factors can be controlled in agricultural settings so as not to confound the search for genetic influences, it’s not so straightforward to extricate the two in humans.
Not to be thwarted, over the past two decades experts have come up with robust statistical techniques to address the issue, using data collected from thousands of individuals. This approach has become particularly prevalent in human genetics, as researchers hope to predict, say, someone’s risk for a disease based on their genome. Some groups have even used these methods to probe how natural selection might have led to observed differences in height (and other traits) among populations. The findings generated further excitement about the potential applications in medicine and evolutionary biology for GWAS.
But now, two results published last month have cast doubt on those findings, and have illustrated that problems with interpretations of GWAS results are far more pervasive than anyone realized. The work has implications for how scientists think about the interactions between genetic and environmental effects. It also “raise[s] the ghosts of the possibility that we overestimate … how important genetics is in contributing to differences between people,” said Rasmus Nielsen, a biologist at the University of California, Berkeley.
Predictions and Dreams
The warning signs started quietly. Genome-wide association studies had already proved incredibly successful at identifying genetic markers for a wide array of traits, even in complex cases where it wasn’t obvious what the many, many variants were doing.
What had also emerged from that research as an “obvious, beguiling offshoot,” according to Nick Barton, an evolutionary biologist at the Institute of Science and Technology Austria, was a specific prediction known as a “polygenic score.” Beyond the associations themselves, GWAS could provide estimates of how individual variants in the genome corresponded to measurable changes in a trait; polygenic scores constituted the sum of all those tiny effects. For instance, with height, having a guanine base instead of a cytosine one in a particular DNA region might correlate with being 0.1 millimeter taller than average. The polygenic score would take all those approximations, add them up and spit out a prediction for some individual’s actual height.
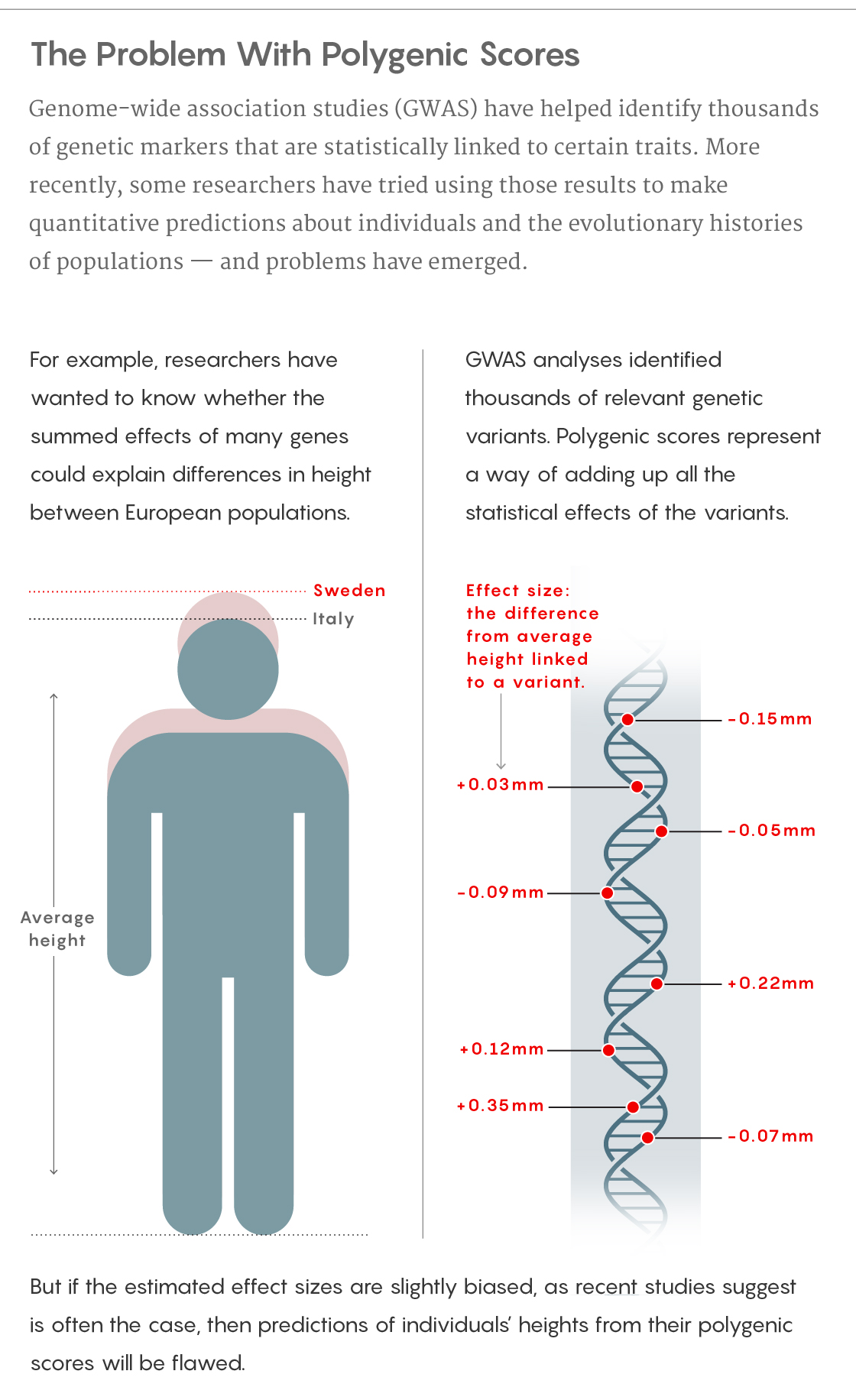
5W Infographics for Quanta Magazine
Polygenic scores offer a tantalizing opportunity to predict a person’s risk of developing a given disease, which could help inform when and how often to screen for it, what preventive measures to take and even which treatments to try. Some evolutionary biologists have hoped to use the scores to get a better picture of the phenotypes that were encoded in ancient genomes, and to better understand histories of adaptation in various populations.
Five years ago, a series of analyses on a European database made these dreams seem within reach. People of northern European ancestry are on average taller than those of southern European ancestry. Researchers wanted to explore whether those differences were a result of natural selection. They found that the polygenic scores for height did indeed increase from southern to northern Europe, much more so than would be expected from the random fluctuations in variant frequencies called genetic drift. That pattern supported the idea that genetics could explain a significant portion of why someone of Swedish descent was likely to be taller than someone with Italian roots — and, it seemed, those genetic differences reflected some process of natural selection and adaptation.
Of course, to reach these conclusions, the researchers had to make sure they weren’t being accidentally misled by subtle environmental factors instead. Take an oft-cited, trivial example: Imagine you have chosen to study the genetics underlying motor skills by investigating how well people use chopsticks. A naïve approach would yield strong associations between chopstick use and certain genetic markers — yet those markers might simply be common among groups of east Asian ancestry who have used chopsticks more often, rather than having anything to do with intrinsic motor skills.
But the scientists had ways to correct for those biases, and the signal of selection on height remained. “We were really excited about that, because we were finally getting to look at … adaptation operating on complex traits,” said Graham Coop, an evolutionary geneticist at the University of California, Davis, who led some of that work along with Jeremy Berg, an evolutionary biologist now at the University of Chicago. Several studies, including one that used sibling data (the closest thing to a “control” experts have in this line of research), replicated their results.
And then the two recent papers in eLife, both relying on a newer database called the U.K. Biobank, saw that signal disappear.
The Vanished Signal
That database was larger and more homogeneous than the one that had driven the previous findings, and had been compiled much more systematically, reducing some of its biases just a bit. As a result, it yielded slightly different estimates for the small effect sizes associated with each of the variants, which weren’t a big deal in individual cases but added up to significantly different overall polygenic scores.
“The new studies are really quite disconcerting,” Barton said, because they demonstrated that scientists had been mistaking biases in the polygenic score calculations for something biologically interesting. Their statistical methods of accounting for population structure were not so adequate after all.
Though it was always understood to be a problem, “no one realized how big of a problem it was,” said Shamil Sunyaev, a computational geneticist at Harvard Medical School who performed one of the new eLife analyses with his graduate student Mashaal Sohail and their colleagues.
“It was just that sort of feeling where the world shifts under your feet slightly,” said Coop, who with Berg and their colleagues coauthored the other eLife paper to try to confirm their earlier research. “It’s fairly humbling to see all of that work go away.”
Barton agreed. “The whole thing is tricky, because the origins of genetic variation in any population are really complicated,” he said. “Now you really can’t take at face value any of these methods over the last four or five years that use polygenic scores.”
“Maybe the Dutch just drink more milk, and this is why they’re taller,” Sunyaev added. “We can’t say otherwise with this analysis.”
And when the trait being studied isn’t height, that can be dangerous. It means that trying to use estimates made about the effects of variants on diseases in one population might not apply to individuals in another population. That’s particularly worrisome because the vast majority of genome-wide association studies rely on data from people of white European ancestry. “People are not homogeneous, and it can come back to haunt you,” said Magnus Nordborg, a population geneticist at the Austrian Academy of Sciences.
Given that some experts want to roll out polygenic scores in the clinic, it’s already clear that this flaw could deepen the disparity in health care. In a study published last month, researchers found that trying to translate insights gleaned from European data to make health predictions in people of African descent led to as much as a 4.5-fold drop in accuracy. Others have tried using polygenic scores to make poorly supported claims about differences in behavioral and social traits between populations (such as IQ and education attainment, which are far more difficult to define and unpack than height is, yet are being used to potentially inform future policymaking decisions). “It’s kind of scary,” said Sarah Tishkoff, a geneticist at the University of Pennsylvania who emphasized how critical it is to collect more underrepresented genomic information.
The eLife work underscores an urgent need for future studies to involve more people, a greater diversity in data and more family-based replication analyses. It also calls for more sophisticated statistical methods that can better control for population structure and other environmental factors — something researchers are already working on as they continue to delve into exactly what went wrong with the initial analyses. “The methods developed so far really think about genetics and environment as separate and orthogonal, as independent factors. When in truth, they’re not independent. The environment has had a strong impact on the genetics, and it probably interacts with the genetics,” said Gil McVean, a statistical geneticist at the University of Oxford. “We don’t really do a good job of … understanding [that] interaction.”
That’s not to say that genome-wide association studies do not have incredible power. They can still identify important markers for traits of interest. The small intrinsic biases don’t invalidate the thousands of regions of DNA such studies have already implicated in diseases and other conditions and traits. But the strengths of those individual and collective associations are general estimates. It’s when they’re accumulated to make inferences about differences between populations, both in evolutionary and medical contexts, that things can go wrong.
“We have to go back to the thinking box,” Nielsen said. “This is a major wake-up call … a game changer.”
Update added on April 24, 2019: The contributions of Mashaal Sohail and others to the work with Shamil Sunyaev were noted.